Q-Learning in enormous action spaces via amortized approximate maximization
arxiv(2020)
摘要
Applying Q-learning to high-dimensional or continuous action spaces can be difficult due to the required maximization over the set of possible actions. Motivated by techniques from amortized inference, we replace the expensive maximization over all actions with a maximization over a small subset of possible actions sampled from a learned proposal distribution. The resulting approach, which we dub Amortized Q-learning (AQL), is able to handle discrete, continuous, or hybrid action spaces while maintaining the benefits of Q-learning. Our experiments on continuous control tasks with up to 21 dimensional actions show that AQL outperforms D3PG (Barth-Maron et al, 2018) and QT-Opt (Kalashnikov et al, 2018). Experiments on structured discrete action spaces demonstrate that AQL can efficiently learn good policies in spaces with thousands of discrete actions.
更多查看译文
AI 理解论文
溯源树
样例
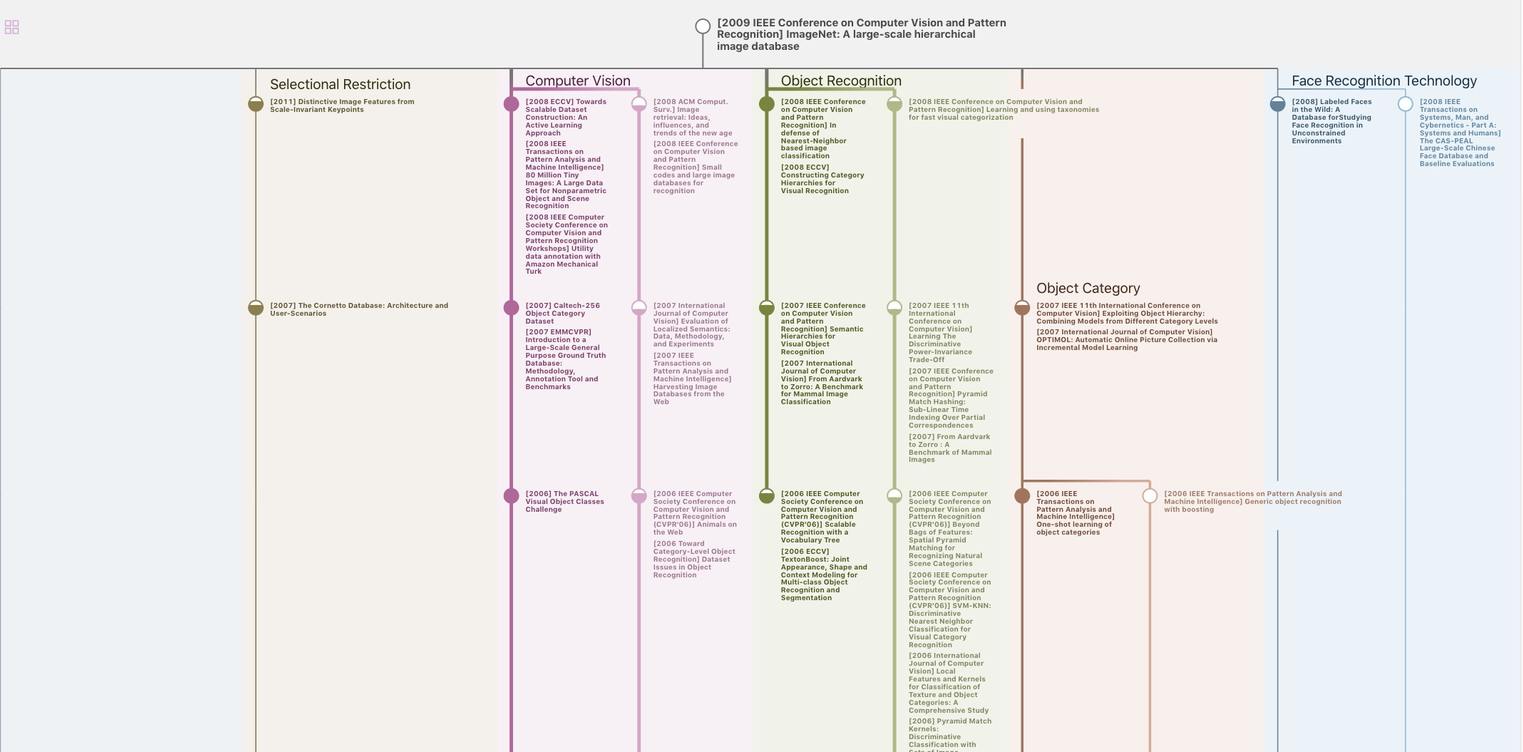
生成溯源树,研究论文发展脉络
Chat Paper
正在生成论文摘要