Learning Functions Varying along a Central Subspace\ast
SIAM JOURNAL ON MATHEMATICS OF DATA SCIENCE(2024)
摘要
Many functions of interest are in a high -dimensional space but exhibit low -dimensional structures. This paper studies regression of an s -Ho"\lder function f in R D which varies along a central subspace of dimension d while d \ll D . A direct approximation of f in R D with an \varepsilon accuracy requires the number of samples n in the order of \varepsilon - (2s +D )/s . In this paper, we analyze the generalized contour regression (GCR) algorithm for the estimation of the central subspace and use piecewise polynomials for function approximation. GCR is among the best estimators for the central subspace, but its sample complexity is an open question. In this paper, we partially answer this questions by proving that if a variance quantity is exactly known, GCR leads to a mean squared estimation error of O ( n - 1 ) for the central subspace. The estimation error of this variance quantity is also given in this 2s paper. The mean squared regression error of f is proved to be in the order of ( n/ log n ) - 2s +d , where the exponent depends on the dimension of the central subspace d instead of the ambient space D . This result demonstrates that GCR is effective in learning the low -dimensional central subspace. We also propose a modified GCR with improved efficiency. The convergence rate is validated through several numerical experiments.
更多查看译文
关键词
nonparametric regression,central subspace,dimension reduction,sample complexity
AI 理解论文
溯源树
样例
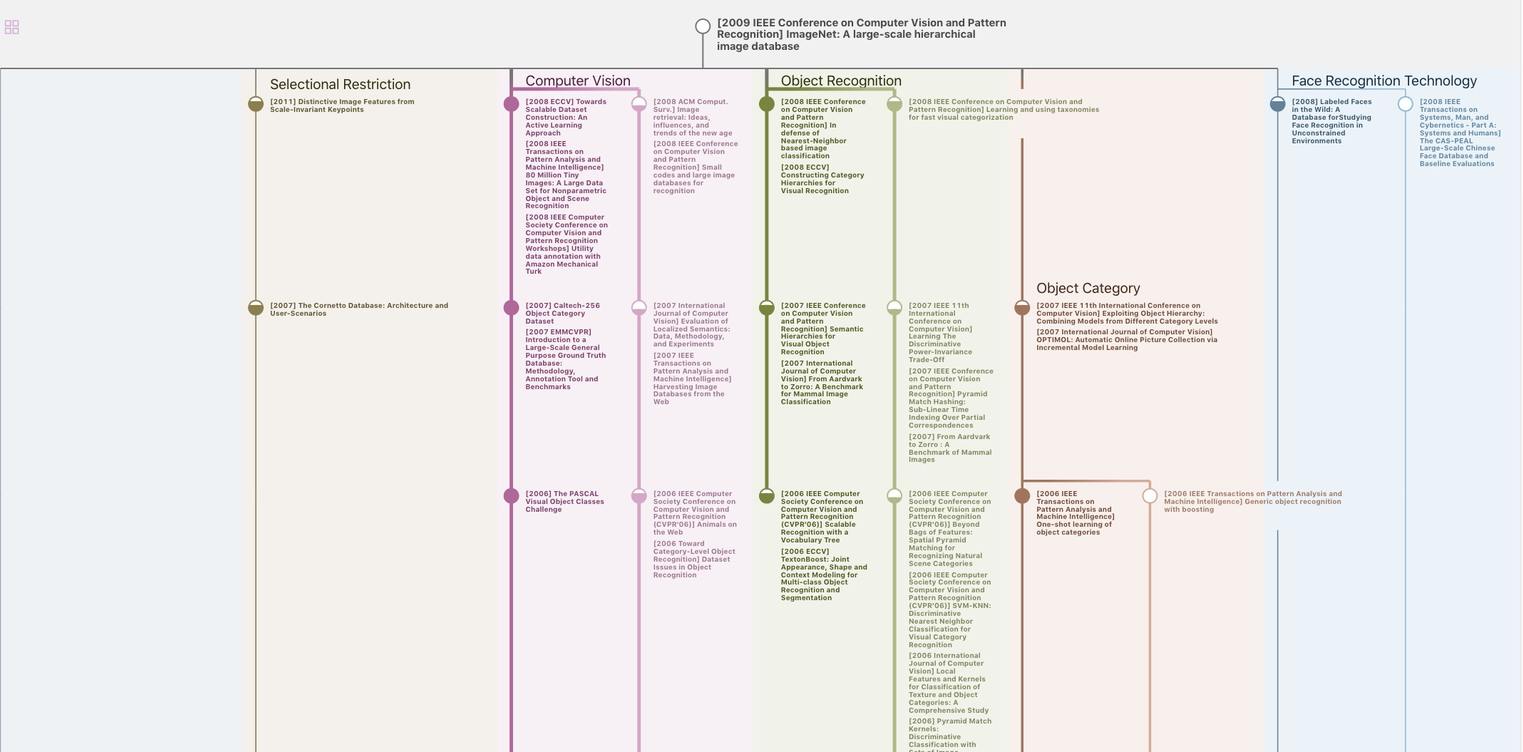
生成溯源树,研究论文发展脉络
Chat Paper
正在生成论文摘要