MixTConv: Mixed Temporal Convolutional Kernels for Efficient Action Recognition
arxiv(2021)
摘要
To efficiently extract spatiotemporal features of video for action recognition, most state-of-the-art methods integrate 1D temporal convolutional filters into 2D CNN backbones. However, they all exploit 1D temporal convolutional filters of fixed kernel size (i.e., 3) in their network building block, thus have suboptimal temporal modeling capability to handle both long-term and short-term actions. To address this problem, we first investigate the impacts of different kernel sizes for the 1D temporal convolutional filters. Then, we propose a simple yet efficient operation called Mixed Temporal Convolution (MixTConv), which consists of multiple depthwise 1D convolutional filters with different kernel sizes. By plugging MixTConv into the conventional 2D CNN backbone ResNet-50, we further propose an efficient and effective network architecture named MSTNet for action recognition, and achieve state-of-the-art results on multiple large-scale benchmarks.
更多查看译文
关键词
MixTConv,action recognition,1D temporal convolutional filters,suboptimal temporal modeling capability,short-term actions,kernel sizes,multiple depthwise 1D convolutional filters,mixed temporal convolutional kernels,MSTNet architecture
AI 理解论文
溯源树
样例
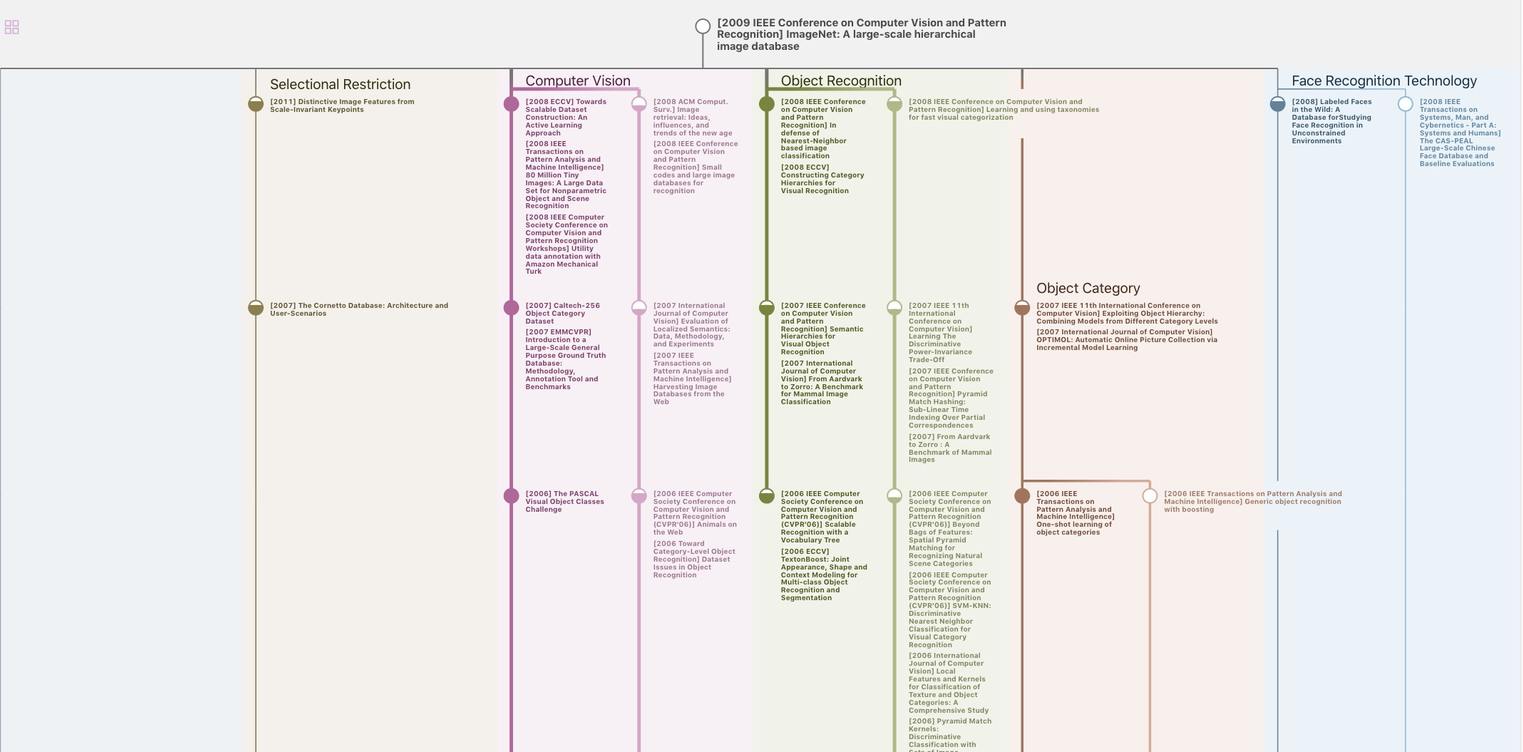
生成溯源树,研究论文发展脉络
Chat Paper
正在生成论文摘要