Unsupervised Learning of Camera Pose with Compositional Re-estimation
2020 IEEE Winter Conference on Applications of Computer Vision (WACV)(2020)
摘要
We consider the problem of unsupervised camera pose estimation. Given an input video sequence, our goal is to estimate the camera pose (i.e. the camera motion) between consecutive frames. Traditionally, this problem is tackled by placing strict constraints on the transformation vector or by incorporating optical flow through a complex pipeline. We propose an alternative approach that utilizes a compositional re-estimation process for camera pose estimation. Given an input, we first estimate a depth map. Our method then iteratively estimates the camera motion based on the estimated depth map. Our approach significantly improves the predicted camera motion both quantitatively and visually. Furthermore, the re-estimation resolves the problem of out-of-boundaries pixels in a novel and simple way. Another advantage of our approach is that it is adaptable to other camera pose estimation approaches. Experimental analysis on KITTI benchmark dataset demonstrates that our method outperforms existing state-of-the-art approaches in unsupervised camera ego-motion estimation.
更多查看译文
关键词
camera motion prediction,unsupervised camera ego-motion estimation,unsupervised learning,camera pose,input video sequence,optical flow,compositional re-estimation process,depth map estimation,KITTI benchmark dataset,transformation vector
AI 理解论文
溯源树
样例
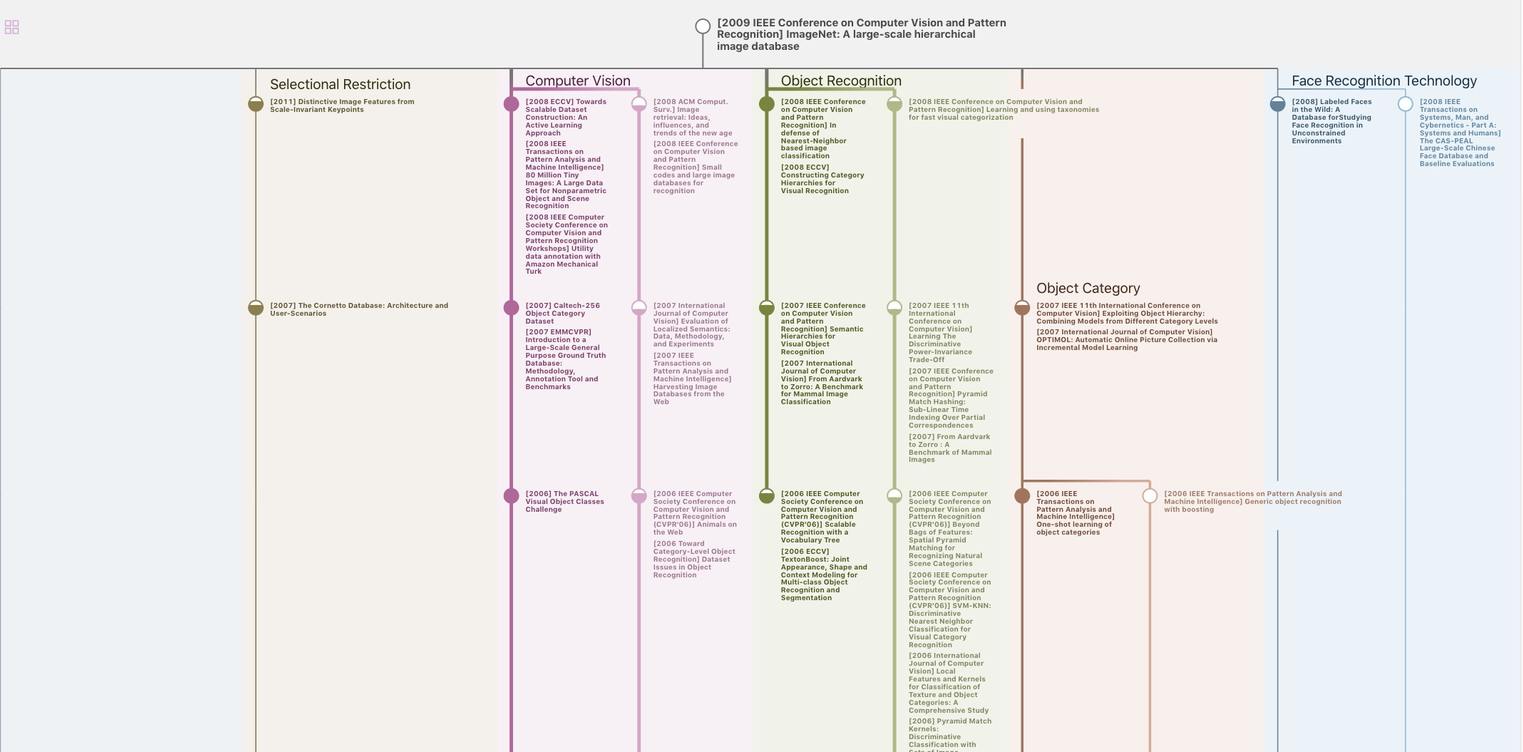
生成溯源树,研究论文发展脉络
Chat Paper
正在生成论文摘要