Epileptic Seizure Classification With Symmetric and Hybrid Bilinear Models
IEEE Journal of Biomedical and Health Informatics(2020)
摘要
Epilepsy affects nearly 1% of the global population, of which two thirds can be treated by anti-epileptic drugs and a much lower percentage by surgery. Diagnostic procedures for epilepsy and monitoring are highly specialized and labour-intensive. The accuracy of the diagnosis is also complicated by overlapping medical symptoms, varying levels of experience and inter-observer variability among clinical professions. This paper proposes a novel hybrid bilinear deep learning network with an application in the clinical procedures of epilepsy classification diagnosis, where the use of surface electroencephalogram (sEEG) and audiovisual monitoring is standard practice. Hybrid bilinear models based on two types of feature extractors, namely Convolutional Neural Networks (CNNs) and Recurrent Neural Networks (RNNs), are trained using Short-Time Fourier Transform (STFT) of one-second sEEG. In the proposed hybrid models, CNNs extract spatio-temporal patterns, while RNNs focus on the characteristics of temporal dynamics in relatively longer intervals given the same input data. Second-order features, based on interactions between these spatio-temporal features are further explored by bilinear pooling and used for epilepsy classification. Our proposed methods obtain an F1-score of 97.4% on the Temple University Hospital Seizure Corpus and 97.2% on the EPILEPSIAE dataset, comparing favourably to existing benchmarks for sEEG-based seizure type classification. The open-source implementation of this study is available at https://github.com/NeuroSyd/Epileptic-SeizureClassification.
更多查看译文
关键词
Algorithms,Deep Learning,Diagnosis, Computer-Assisted,Electroencephalography,Fourier Analysis,Humans,Neural Networks, Computer,Seizures
AI 理解论文
溯源树
样例
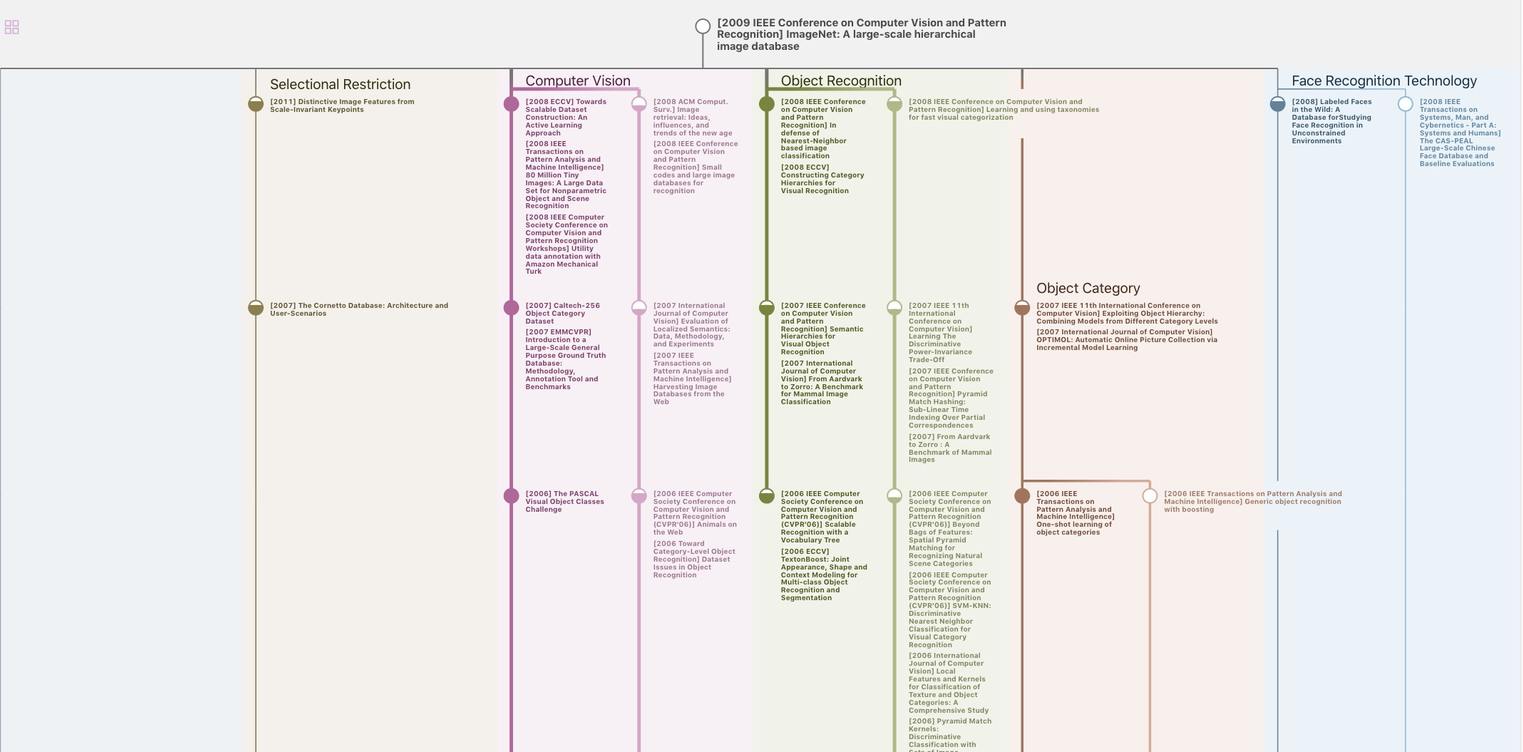
生成溯源树,研究论文发展脉络
Chat Paper
正在生成论文摘要