Smart Data Driven Decision Trees Ensemble Methodology for Imbalanced Big Data
Cognitive Computation(2024)
摘要
Differences in data size per class, also known as imbalanced data distribution, have become a common problem affecting data quality. Big Data scenarios pose a new challenge to traditional imbalanced classification algorithms, since they are not prepared to work with such amount of data. Split data strategies and lack of data in the minority class due to the use of MapReduce paradigm have posed new challenges for tackling the imbalance between classes in Big Data scenarios. Ensembles have been shown to be able to successfully address imbalanced data problems. Smart Data refers to data of enough quality to achieve high-performance models. The combination of ensembles and Smart Data, achieved through Big Data preprocessing, should be a great synergy. In this paper, we propose a novel Smart Data driven Decision Trees Ensemble methodology for addressing the imbalanced classification problem in Big Data domains, namely SD_DeTE methodology. This methodology is based on the learning of different decision trees using distributed quality data for the ensemble process. This quality data is achieved by fusing random discretization, principal components analysis, and clustering-based random oversampling for obtaining different Smart Data versions of the original data. Experiments carried out in 21 binary adapted datasets have shown that our methodology outperforms random forest.
更多查看译文
关键词
Big data,Smart data,Classification,Ensemble,Imbalanced data,Decision tree
AI 理解论文
溯源树
样例
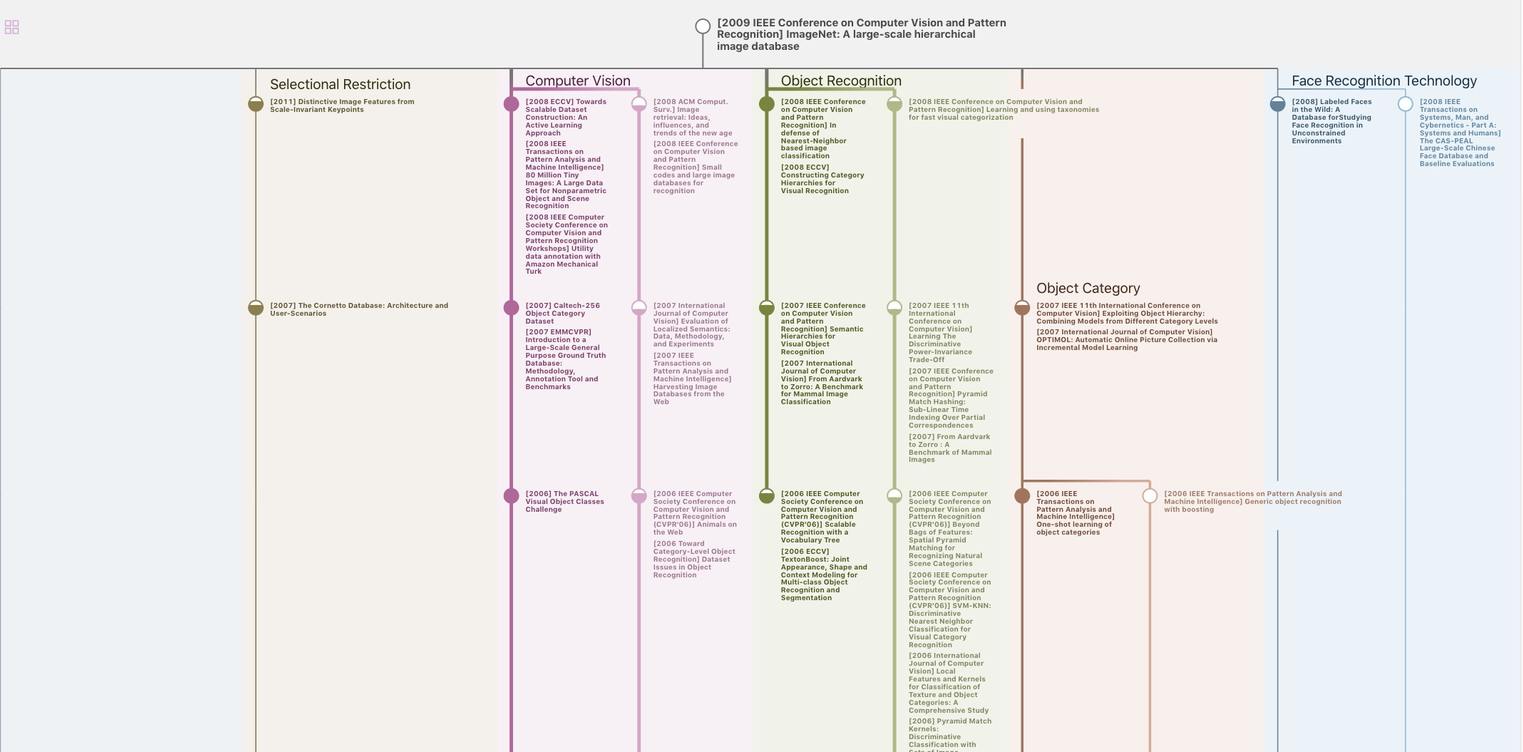
生成溯源树,研究论文发展脉络
Chat Paper
正在生成论文摘要