Multicategory Angle-Based Learning for Estimating Optimal Dynamic Treatment Regimes With Censored Data
JOURNAL OF THE AMERICAN STATISTICAL ASSOCIATION(2022)
摘要
An optimal dynamic treatment regime (DTR) consists of a sequence of decision rules in maximizing long-term benefits, which is applicable for chronic diseases such as HIV infection or cancer. In this article, we develop a novel angle-based approach to search the optimal DTR under a multicategory treatment framework for survival data. The proposed method targets to maximize the conditional survival function of patients following a DTR. In contrast to most existing approaches which are designed to maximize the expected survival time under a binary treatment framework, the proposed method solves the multicategory treatment problem given multiple stages for censored data. Specifically, the proposed method obtains the optimal DTR via integrating estimations of decision rules at multiple stages into a single multicategory classification algorithm without imposing additional constraints, which is also more computationally efficient and robust. In theory, we establish Fisher consistency and provide the risk bound for the proposed estimator under regularity conditions. Our numerical studies show that the proposed method outperforms competing methods in terms of maximizing the conditional survival probability. We apply the proposed method to two real datasets: Framingham heart study data and acquired immunodeficiency syndrome clinical data. for this article are available online.
更多查看译文
关键词
Classification, Inverse probability weighting, Kaplan-Meier estimator, Outcome weighted learning, Precision medicine, Survival function
AI 理解论文
溯源树
样例
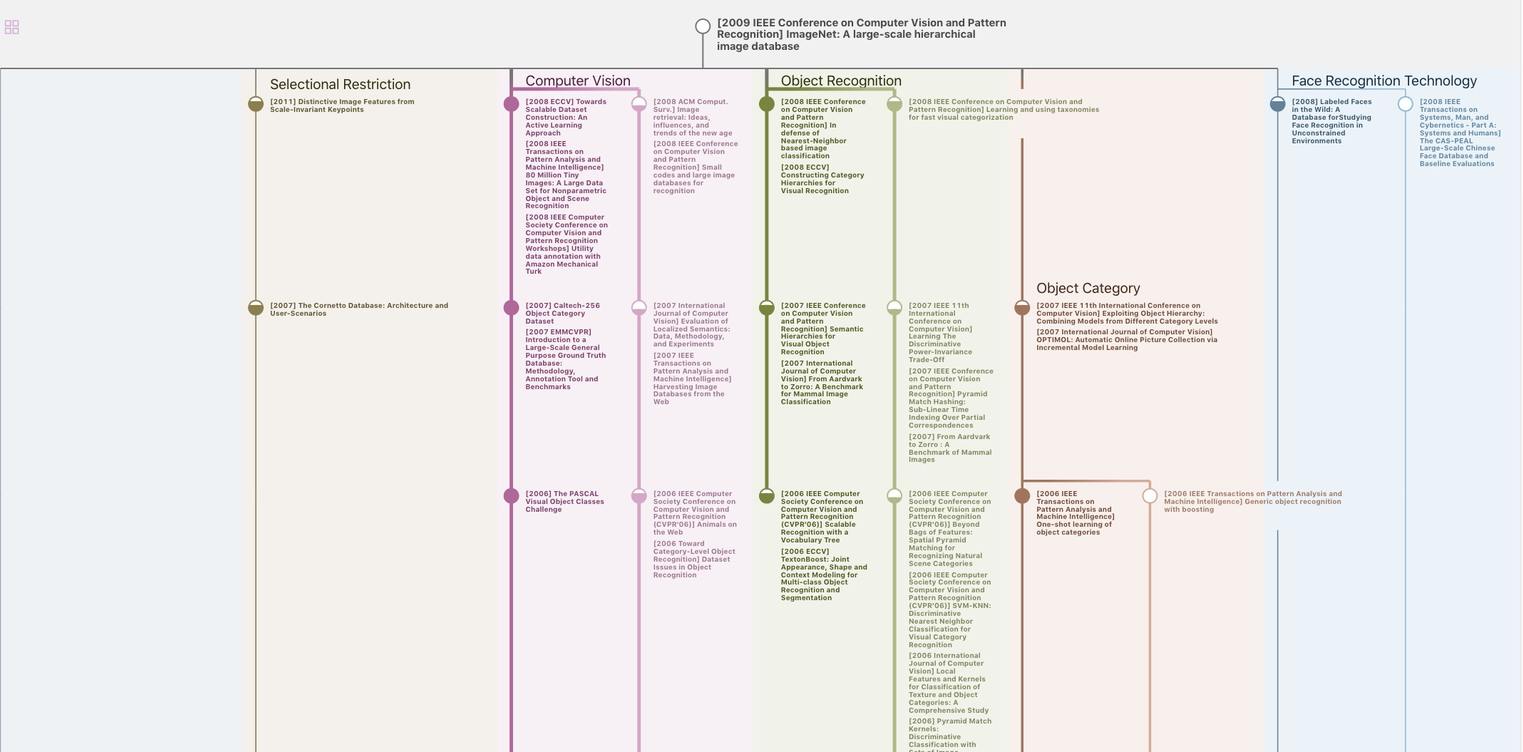
生成溯源树,研究论文发展脉络
Chat Paper
正在生成论文摘要