Inference for linear forms of eigenvectors under minimal eigenvalue separation: Asymmetry and heteroscedasticity
arxiv(2021)
摘要
A fundamental task that spans numerous applications is inference and uncertainty quantification for linear functionals of the eigenvectors of an unknown low-rank matrix. We prove that this task can be accomplished in a setting where the true matrix is symmetric and the additive noise matrix contains independent (and non-symmetric) entries. Specifically, we develop algorithms that produce confidence intervals for linear forms of individual eigenvectors, based on eigen-decomposition of the asymmetric data matrix followed by a careful de-biasing scheme. The proposed procedures and the accompanying theory enjoy several important features: (1) distribution-free (i.e. prior knowledge about the noise distributions is not needed); (2) adaptive to heteroscedastic noise; (3) statistically optimal under Gaussian noise. Along the way, we establish procedures to construct optimal confidence intervals for the eigenvalues of interest. All this happens under minimal eigenvalue separation, a condition that goes far beyond what generic matrix perturbation theory has to offer. Our studies fall under the category of "fine-grained" functional inference in low-complexity models.
更多查看译文
关键词
Estimation,Eigenvalues and eigenfunctions,Symmetric matrices,Uncertainty,Perturbation methods,Matrix decomposition,Matrix converters
AI 理解论文
溯源树
样例
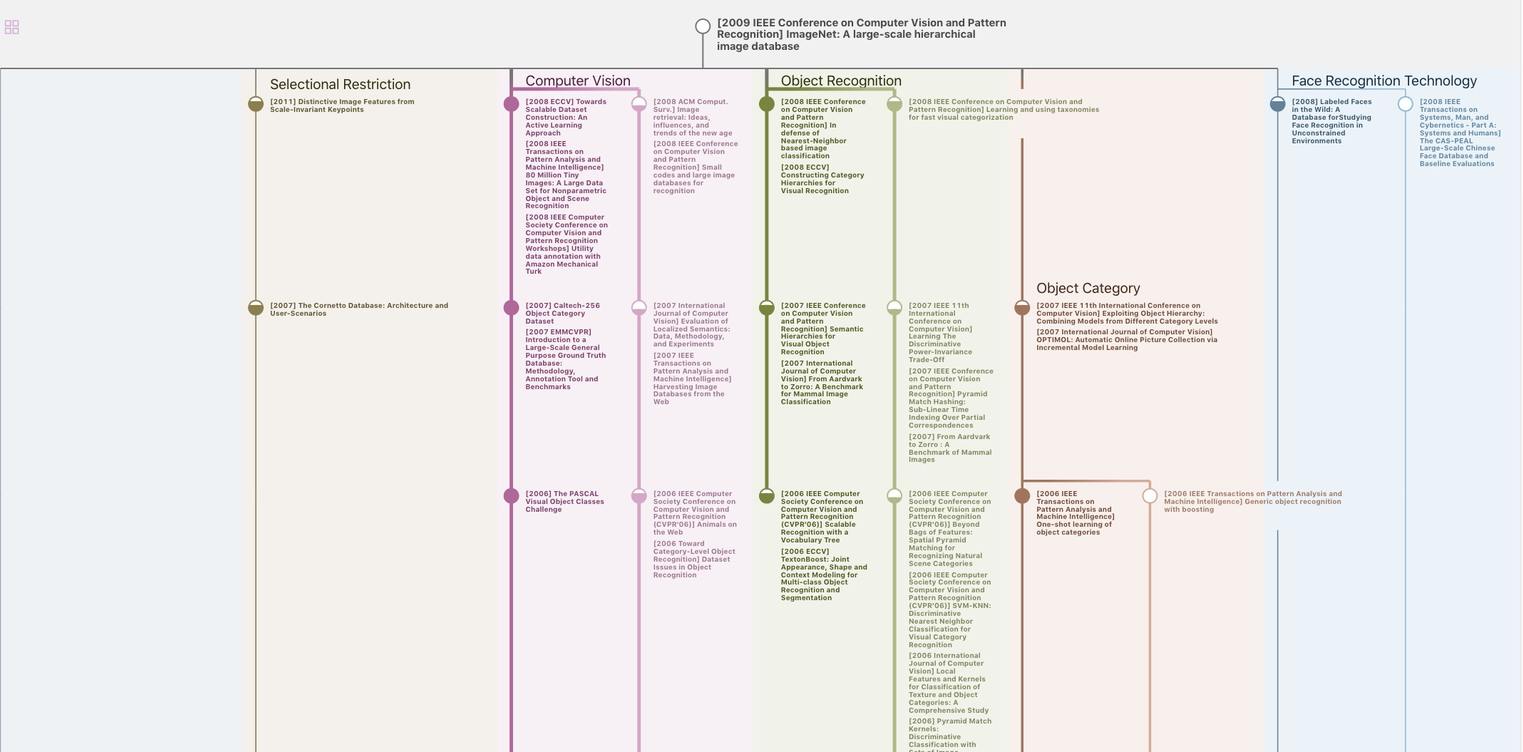
生成溯源树,研究论文发展脉络
Chat Paper
正在生成论文摘要