A scalable method for parameter-free simulation and validation of mechanistic cellular signal transduction network models
NPJ SYSTEMS BIOLOGY AND APPLICATIONS(2020)
摘要
The metabolic modelling community has established the gold standard for bottom-up systems biology with reconstruction, validation and simulation of mechanistic genome-scale models. Similar methods have not been established for signal transduction networks, where the representation of complexes and internal states leads to scalability issues in both model formulation and execution. While rule- and agent-based methods allow efficient model definition and execution, respectively, model parametrisation introduces an additional layer of uncertainty due to the sparsity of reliably measured parameters. Here, we present a scalable method for parameter-free simulation of mechanistic signal transduction networks. It is based on rxncon and uses a bipartite Boolean logic with separate update rules for reactions and states. Using two generic update rules, we enable translation of any rxncon model into a unique Boolean model, which can be used for network validation and simulation—allowing the prediction of system-level function directly from molecular mechanistic data. Through scalable model definition and simulation, and the independence of quantitative parameters, it opens up for simulation and validation of mechanistic genome-scale models of signal transduction networks.
更多查看译文
关键词
Biochemical networks,Molecular biology,Software
AI 理解论文
溯源树
样例
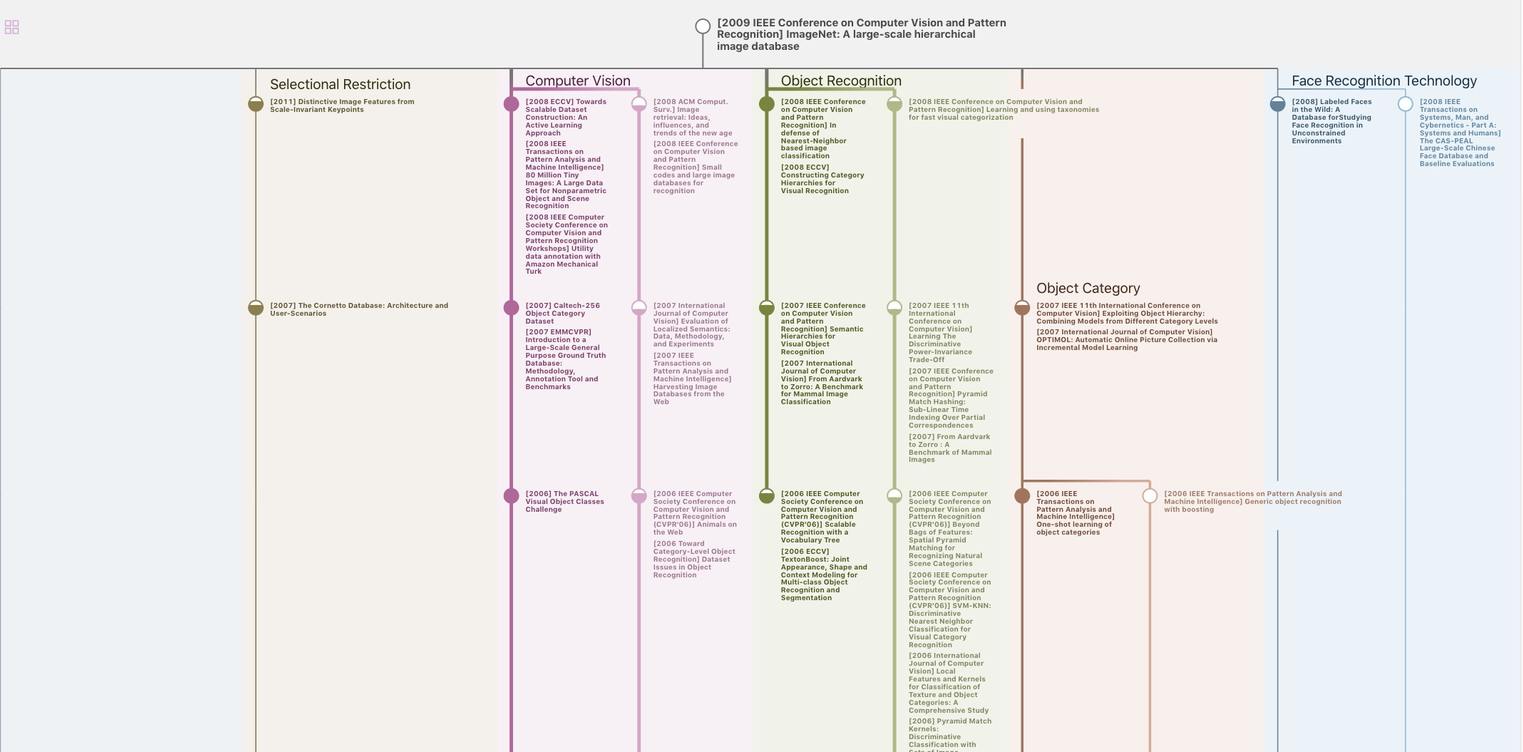
生成溯源树,研究论文发展脉络
Chat Paper
正在生成论文摘要