Approximation smooth and sparse functions by deep neural networks without saturation
arxiv(2020)
摘要
Constructing neural networks for function approximation is a classical and longstanding topic in approximation theory. In this paper, we aim at constructing deep neural networks (deep nets for short) with three hidden layers to approximate smooth and sparse functions. In particular, we prove that the constructed deep nets can reach the optimal approximation rate in approximating both smooth and sparse functions with controllable magnitude of free parameters. Since the saturation that describes the bottleneck of approximate is an insurmountable problem of constructive neural networks, we also prove that deepening the neural network with only one more hidden layer can avoid the saturation. The obtained results underlie advantages of deep nets and provide theoretical explanations for deep learning.
更多查看译文
关键词
Approximation theory,Deep learning,Deep neural networks,Localized approximation,Sparse approximation
AI 理解论文
溯源树
样例
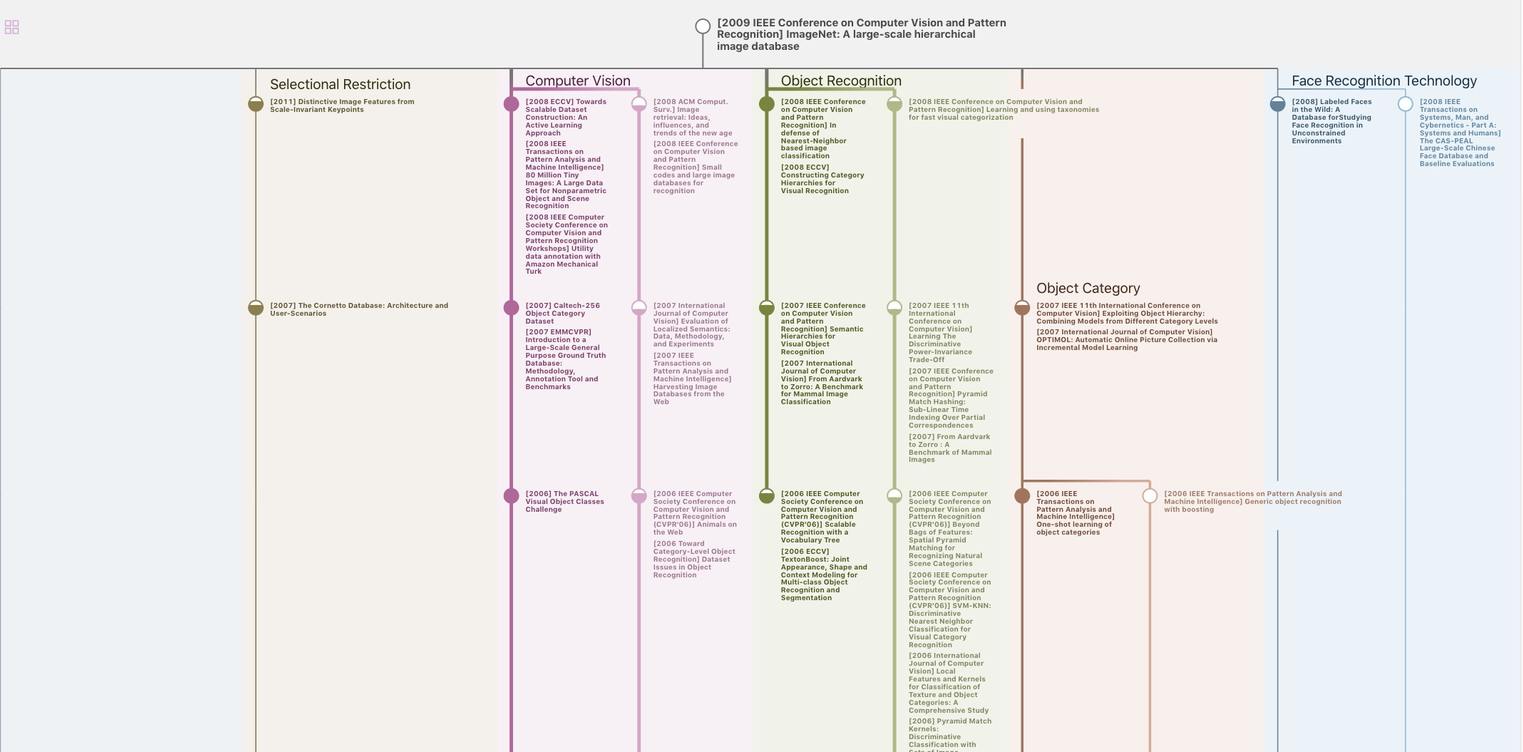
生成溯源树,研究论文发展脉络
Chat Paper
正在生成论文摘要