Single View 3D Point Cloud Reconstruction using Novel View Synthesis and Self-Supervised Depth Estimation
2019 Digital Image Computing: Techniques and Applications (DICTA)(2019)
摘要
Capturing large amounts of accurate and diverse 3D data for training is often time consuming and expensive, either requiring many hours of artist time to model each object, or to scan from real world objects using depth sensors or structure from motion techniques. To address this problem, we present a method for reconstructing 3D textured point clouds from single input images without any 3D ground truth training data. We recast the problem of 3D point cloud estimation as that of performing two separate processes, a novel view synthesis and a depth/shape estimation from the novel view images. To train our models we leverage the recent advances in deep generative modelling and self-supervised learning. We show that our method outperforms recent supervised methods, and achieves state of the art results when compared with another recently proposed unsupervised method. Furthermore, we show that our method is capable of recovering textural information which is often missing from many previous approaches that rely on supervision.
更多查看译文
关键词
Deep Learning,3D Reconstruction,Deep Generative Modelling,Self-Supervised Learning,Depth Estimation
AI 理解论文
溯源树
样例
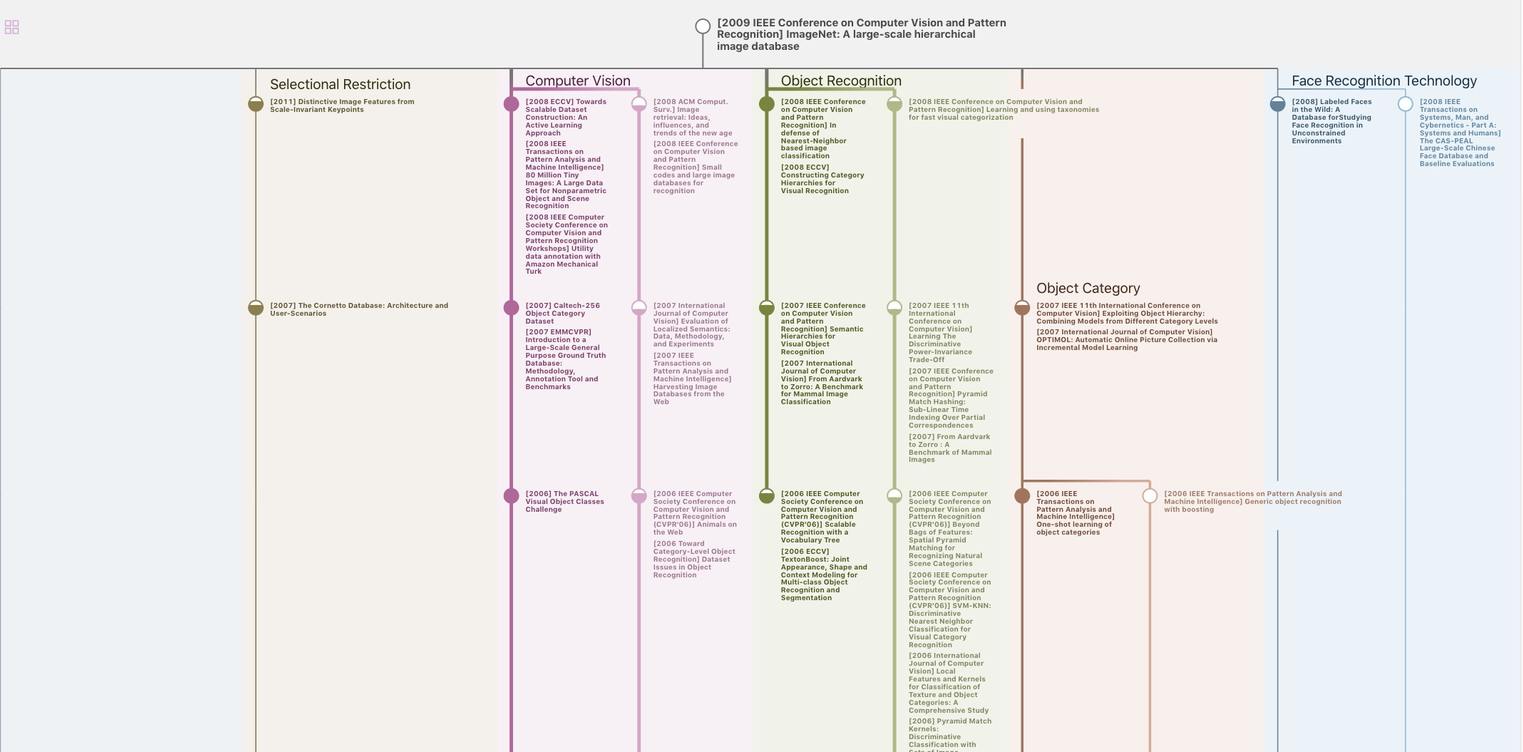
生成溯源树,研究论文发展脉络
Chat Paper
正在生成论文摘要