Temporal Tensor Transformation Network for Multivariate Time Series Prediction
2020 IEEE INTERNATIONAL CONFERENCE ON BIG DATA (BIG DATA)(2020)
Abstract
Multivariate time series prediction has applications in a wide variety of domains and is considered to be a very challenging task, especially when the variables have correlations and exhibit complex temporal patterns, such as seasonality and trend. Many existing methods suffer from strong statistical assumptions, numerical issues with high dimensionality, manual feature engineering efforts, and scalability. In this work, we present a novel deep learning architecture, known as Temporal Tensor Transformation Network, which transforms the original multivariate time series into a higher order of tensor through the proposed Temporal-Slicing Stack Transformation. This yields a new representation of the original multivariate time series, which enables the convolution kernel to extract complex and non-linear features as well as variable interactional signals from a relatively large temporal region. Experimental results show that Temporal Tensor Transformation Network outperforms several state-of-the-art methods on window-based predictions across various tasks. The proposed architecture also demonstrates robust prediction performance through an extensive sensitivity analysis.
MoreTranslated text
Key words
multivariate time series,deep learning,prediction,convolution,tensor transformation
AI Read Science
Must-Reading Tree
Example
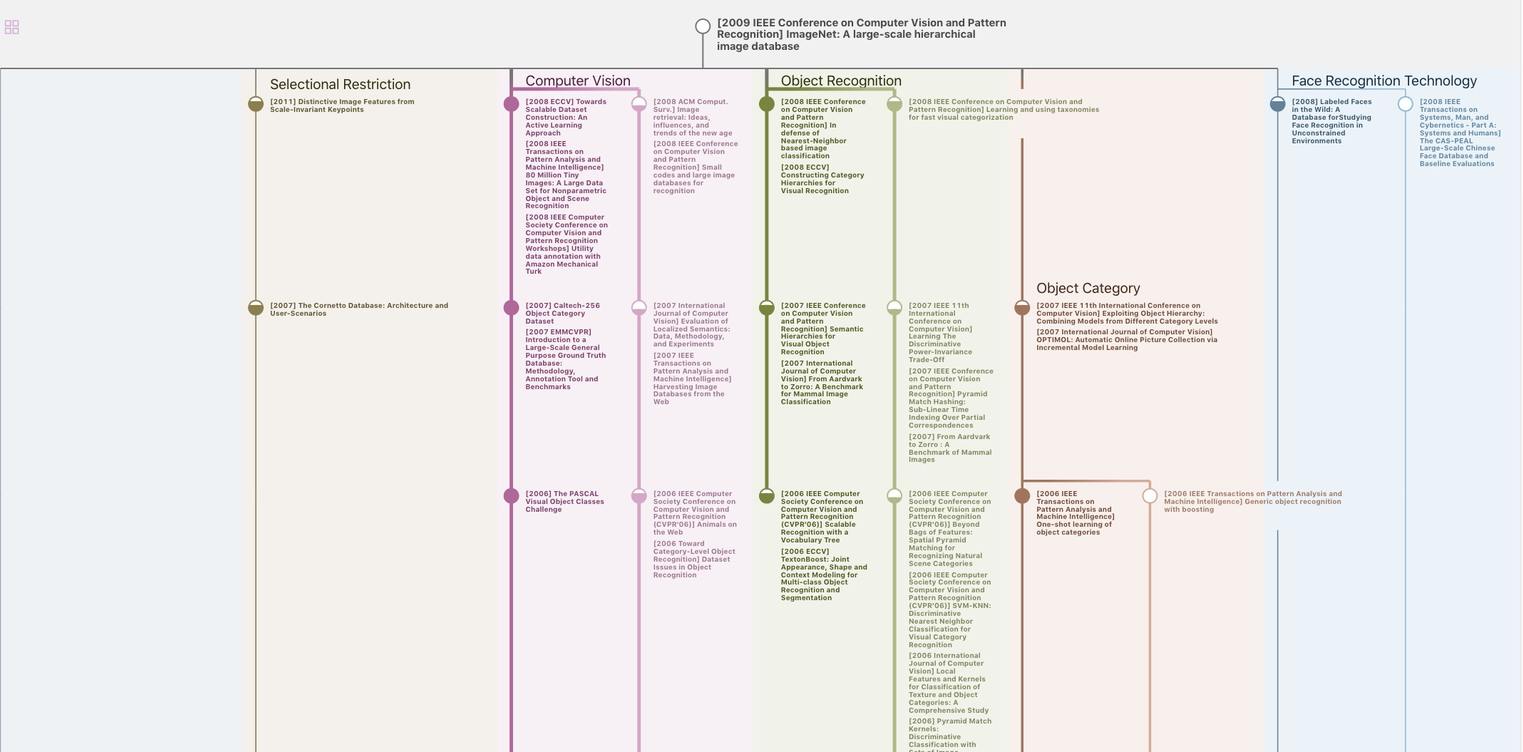
Generate MRT to find the research sequence of this paper
Chat Paper
Summary is being generated by the instructions you defined