Convolutional autoencoder and conditional random fields hybrid for predicting spatial-temporal chaos.
CHAOS(2019)
摘要
We present an approach for data-driven prediction of high-dimensional chaotic time series generated by spatially-extended systems. The algorithm employs a convolutional autoencoder for dimension reduction and feature extraction combined with a probabilistic prediction scheme operating in the feature space, which consists of a conditional random field. The future evolution of the spatially-extended system is predicted using a feedback loop and iterated predictions. The excellent performance of this method is illustrated and evaluated using Lorenz-96 systems and Kuramoto-Sivashinsky equations of different size generating time series of different dimensionality and complexity. Published under license by AIP Publishing.
更多查看译文
关键词
convolutional autoencoder,chaos,conditional random fields,spatial-temporal
AI 理解论文
溯源树
样例
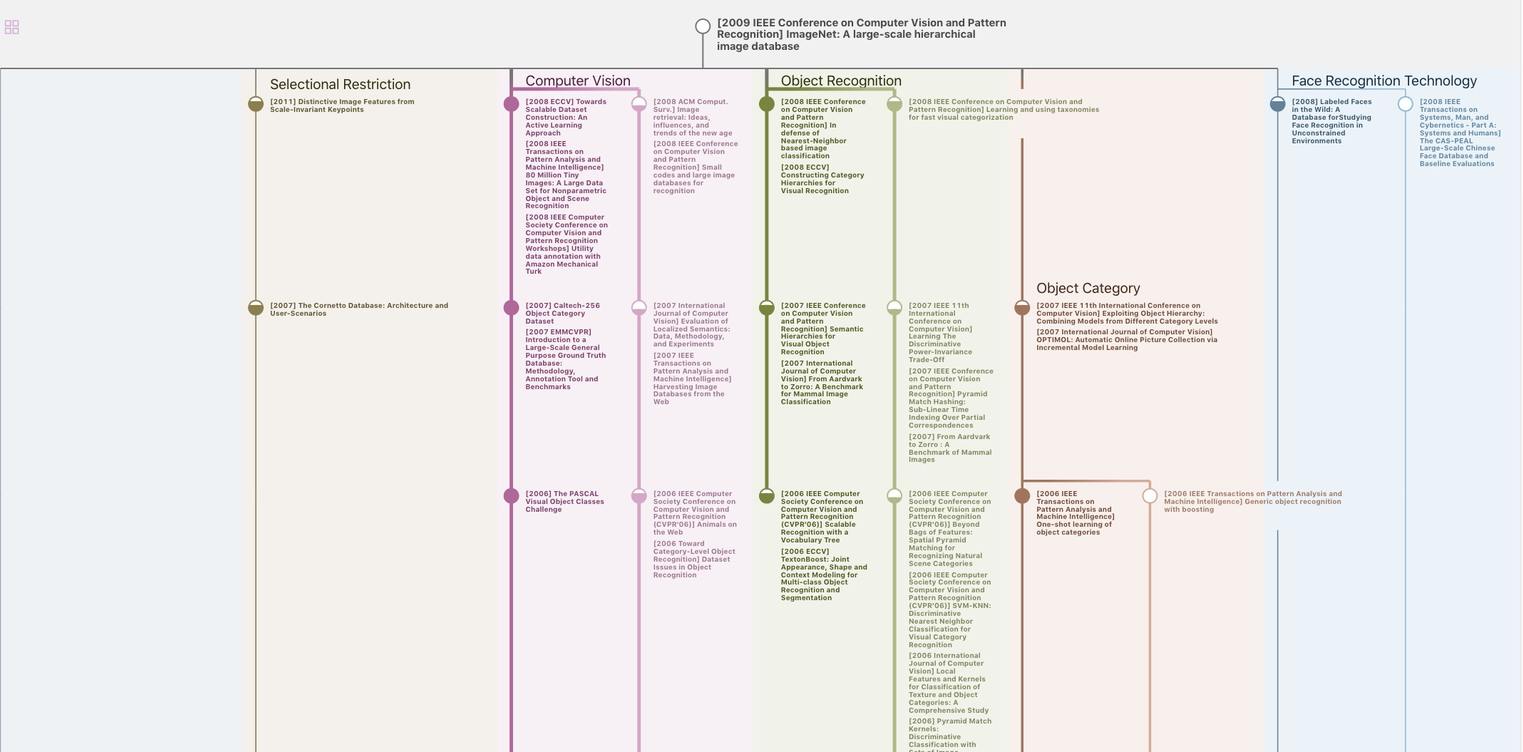
生成溯源树,研究论文发展脉络
Chat Paper
正在生成论文摘要