Efficient Data Analytics on Augmented Similarity Triplets
CoRR(2023)
摘要
Data analysis require a pairwise proximity measure over objects. Recent work has extended this to situations where the distance information between objects is given as comparison results of distances between three objects (triplets). Humans find the comparison tasks much easier than the exact distance computation and such data can be easily obtained in big quantity via crowd-sourcing. In this work, we propose triplets augmentation, an efficient method to extend the triplets data by inferring the hidden implicit information form the existing data. Triplets augmentation improves the quality of kernel-based and kernel-free data analytics. We also propose a novel set of algorithms for common data analysis tasks based on triplets. These methods work directly with triplets and avoid kernel evaluations, thus are scalable to big data. We demonstrate that our methods outperform the current best-known techniques and are robust to noisy data.
更多查看译文
关键词
Data augmentation,Crowd-sourcing,Triplets,Relative Similarity
AI 理解论文
溯源树
样例
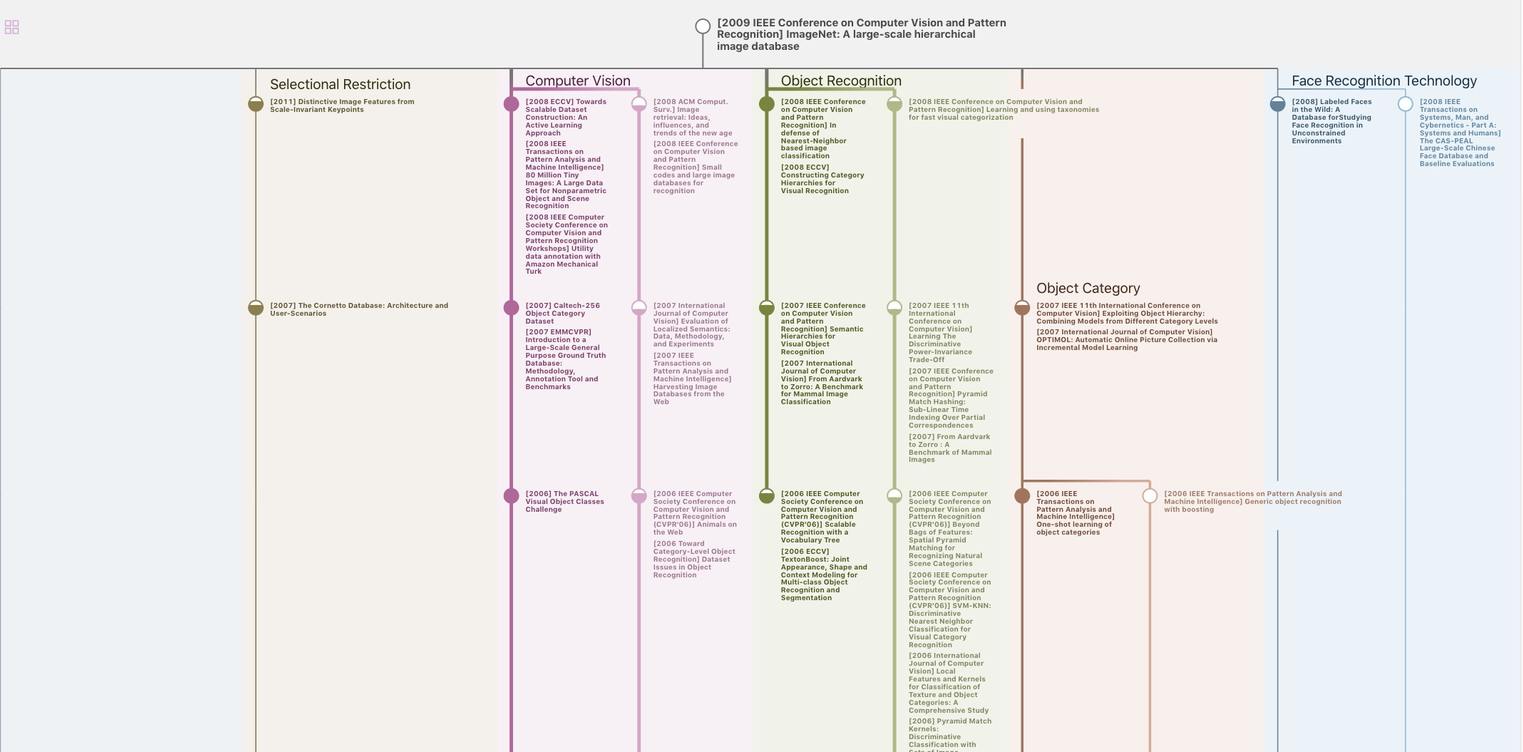
生成溯源树,研究论文发展脉络
Chat Paper
正在生成论文摘要