Efficient Adversarial Training With Transferable Adversarial Examples
2020 IEEE/CVF CONFERENCE ON COMPUTER VISION AND PATTERN RECOGNITION (CVPR)(2020)
摘要
Adversarial training is an effective defense method to protect classification models against adversarial attacks. However, one limitation of this approach is that it can require orders of magnitude additional training time due to high cost of generating strong adversarial examples during training. In this paper, we first show that there is high transferability between models from neighboring epochs in the same training process, i.e., adversarial examples from one epoch continue to be adversarial in subsequent epochs. Leveraging this property, we propose a novel method, Adversarial Training with Transferable Adversarial Examples (ATTA), that can enhance the robustness of trained models and greatly improve the training efficiency by accumulating adversarial perturbations through epochs. Compared to state-of-the-art adversarial training methods, AIM enhances adversarial accuracy by up to 7.2% on CIFAR10 and requires 12 similar to 14x less training time on MNIST and CIFAR10 datasets with comparable model robustness.
更多查看译文
关键词
effective defense method,classification models,adversarial attacks,epoch,adversarial perturbations,adversarial accuracy,adversarial training with transferable adversarial examples,ATTA,CIFAR10 dataset,MNIST dataset
AI 理解论文
溯源树
样例
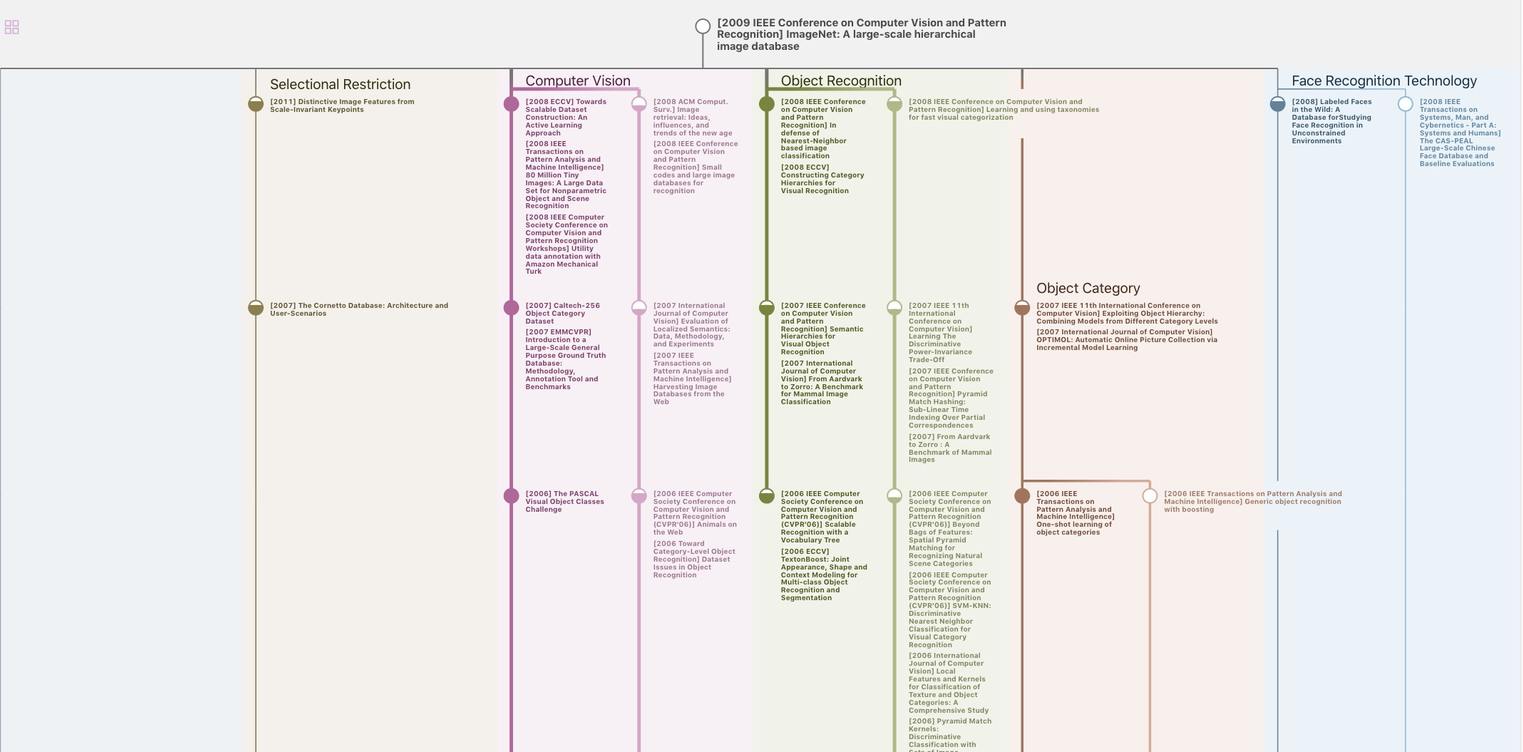
生成溯源树,研究论文发展脉络
Chat Paper
正在生成论文摘要