Multi-Level Metric Learning Network For Fine-Grained Classification
IEEE ACCESS(2019)
摘要
The application of fine-grained image classification can be problematic due to subtle differences between classes. The existing global feature-based methods have worse accuracies than regional feature-based methods, because regional feature-based methods focus on the determination of differentiated features within local regions. To learn more discriminative global features, in this paper, we proposed the use of L2 normalization to tackle a neglected conflict between the widely used metric loss (triplet loss) and classification loss (softmax loss) in global feature-based methods. Furthermore, a multi-level metric learning network (MMLN) is proposed for fine-grained image classification based on global features. In the MMLN, multi-level metric learning objectives and classification objectives are present at multiple high-level layers. The multi-level metric learning objectives work together to supervise the network in order to learn highly discriminative features. In addition, a new probability aggregation strategy (PAS) is proposed to produce a fused prediction by combining the multi-level predictive probabilities. Experiments were conducted on three standard fine-grained classification datasets (CUB-200-2011, Stanford Cars, and FGVC-Aircraft). Results demonstrated that our MMLN achieved accuracies of 88.0%, 94.6% and 92.4% respectively and outperformed state-of-the-art methods, substantially improving fine-grained classification tasks. Besides, gradient-weighted class activation mapping (Grad-CAM) shows that the MMLN is able to pay more attention to the discriminative local regions due to the application of multi-level metric learning.
更多查看译文
关键词
Fine-grained recognition,metric learning,multi-level objectives,classification
AI 理解论文
溯源树
样例
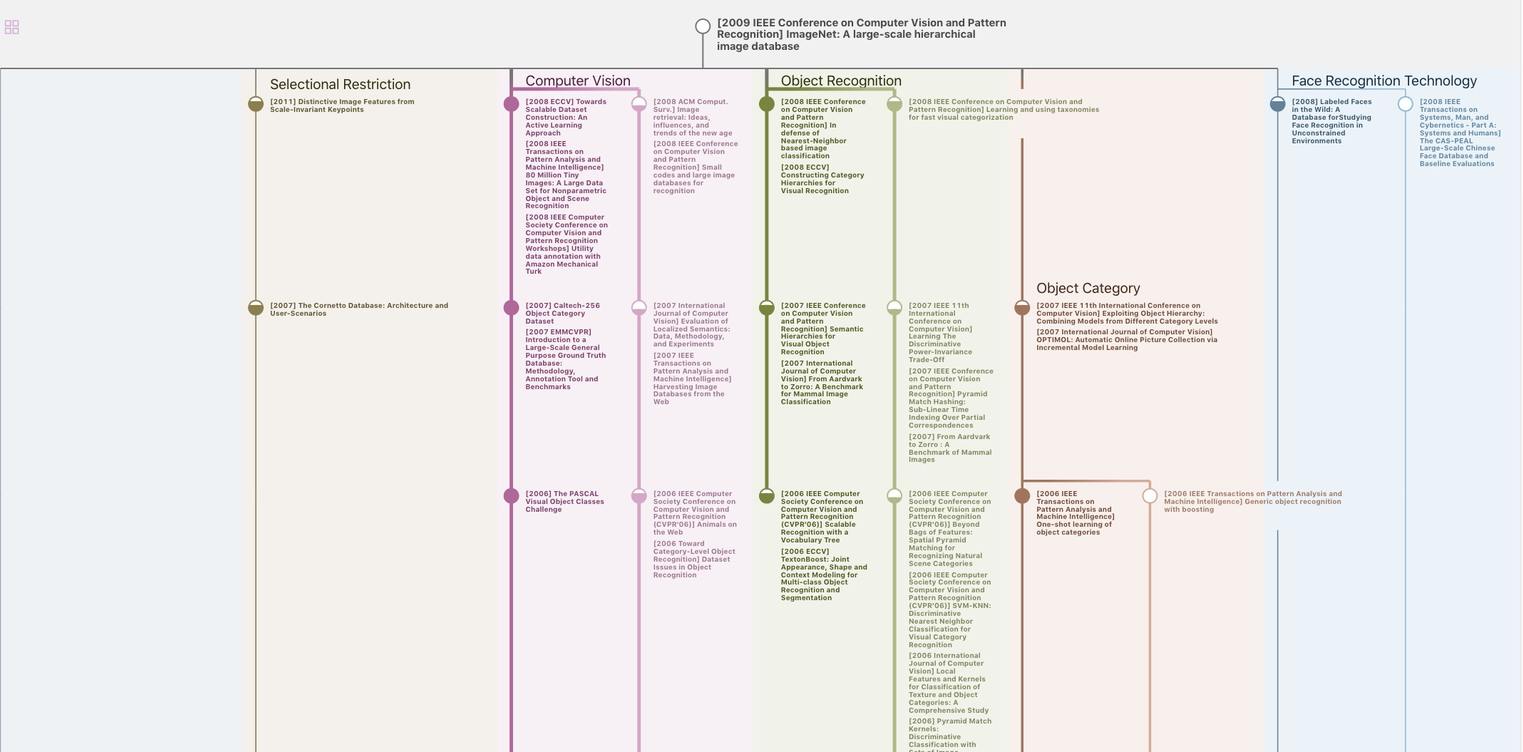
生成溯源树,研究论文发展脉络
Chat Paper
正在生成论文摘要