PCP-2LSTM: Two Stacked LSTM-Based Prediction Model for Power Consumption in Data Centers
2019 Seventh International Conference on Advanced Cloud and Big Data (CBD)(2019)
摘要
As the size of data centers and cloud computing continue to expand, power consumption in data centers is rapidly increasing. It has a great significance to predict and analyze power consumption in the data center because power consumption prediction can help data center operators perform workflow scheduling, manage energy efficiency, provide high quality-of-service (QoS), and meet the requirements of green energy use. The current methods are mainly divided into two scopes: the one is establishing a static relationship between power consumption and relevant components/applications, and the other one is treating power consumption as sequential temporal data. However, the first scope does not consider the dynamic fluctuation of power, and the other one ignores the characteristics of the power consumption data. To solve these issues, in this paper, we present a power consumption prediction framework called PCP-2LSTM based on the mean smoothing and long short-term memory (LSTM) network. We first build a power consumption system to collect data and analyze the stationary of the power series. Then we use the mean smoothing to remove the noise from the time series of power consumption. After data preprocessing, because the time for workflow and container scheduling is usually 30 seconds, we use a stacked LSTM model to predict 30s power consumption in the future. The experimental result indicates that our approach outperforms other baselines.
更多查看译文
关键词
Data center, time series prediction, energy efficiency, power consumption
AI 理解论文
溯源树
样例
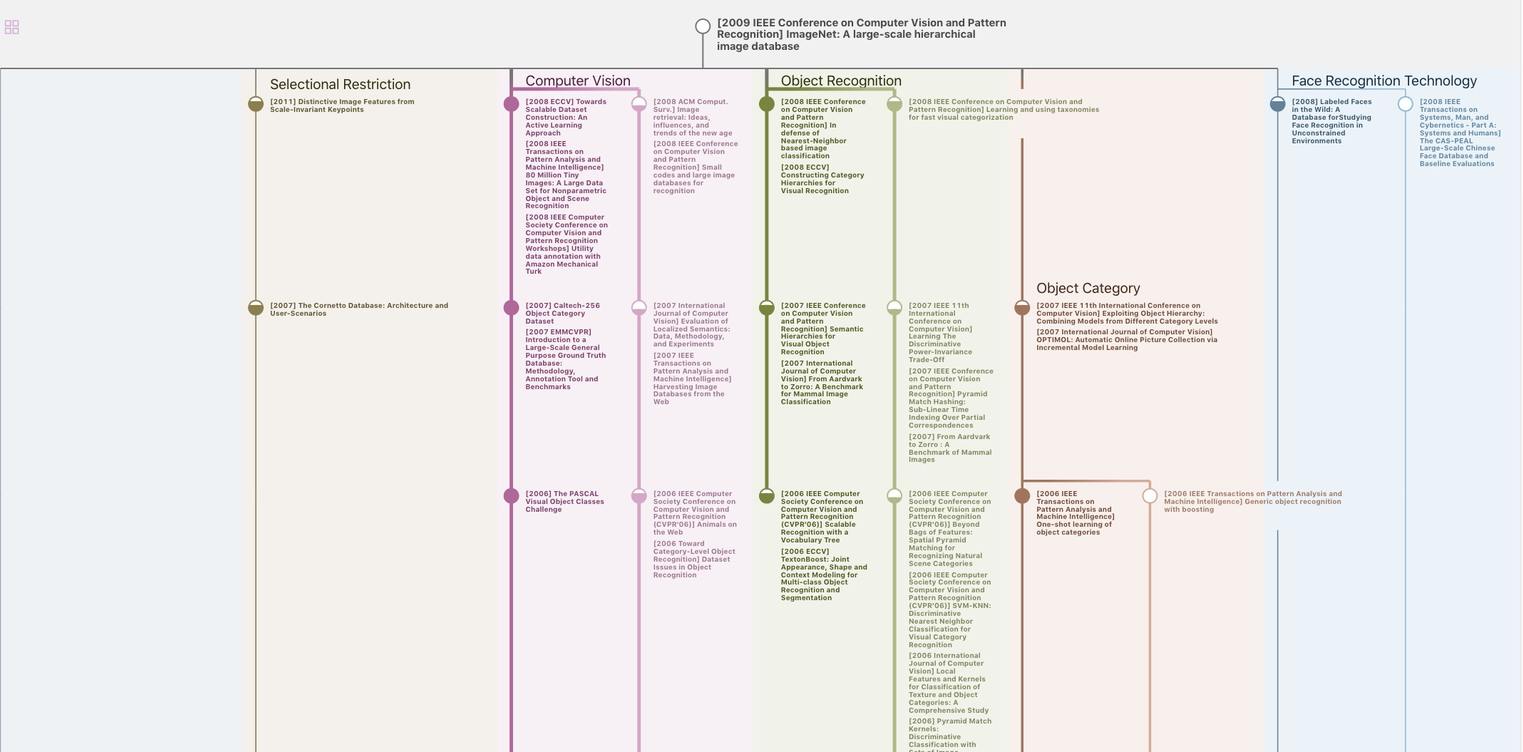
生成溯源树,研究论文发展脉络
Chat Paper
正在生成论文摘要