Autonomous Embedded System Enabled 3-D Object Detector: (with Point Cloud and Camera)
2019 IEEE International Conference on Vehicular Electronics and Safety (ICVES)(2019)
摘要
An Autonomous vehicle or present day smart vehicle is equipped with several ADAS safety features such as Blind Spot Detection, Forward Collision Warning, Lane Departure and Parking Assistance, Surround View System, Vehicular communication System. Recent research utilize deep learning algorithms as a counterfeit for these traditional methods, using optimal sensors. This paper discusses the perception tasks related to autonomous vehicle, specifically the computer-vision approach of 3D object detection and thus proposes a model compatible with embedded system using the RTMaps framework. The proposed model is based on the sensors: camera and Lidar connected to an autonomous embedded system, providing the sensed inputs to the deep learning classifier which on the basis of theses inputs estimates the position and predicts a 3-d bounding box on the physical objects. The Frustum PointNet a contemporary architecture for 3-D object detection is used as base model and is implemented with extended functionality. The architecture is trained and tested on the KITTI dataset and is discussed with the competitive validation precision and accuracy. The Presented model is deployed on the Bluebox 2.0 platform with the RTMaps Embedded framework.
更多查看译文
关键词
Autonomous vehicles,BLBX2,camera,lidar,KITTI,object detection,RTMaps
AI 理解论文
溯源树
样例
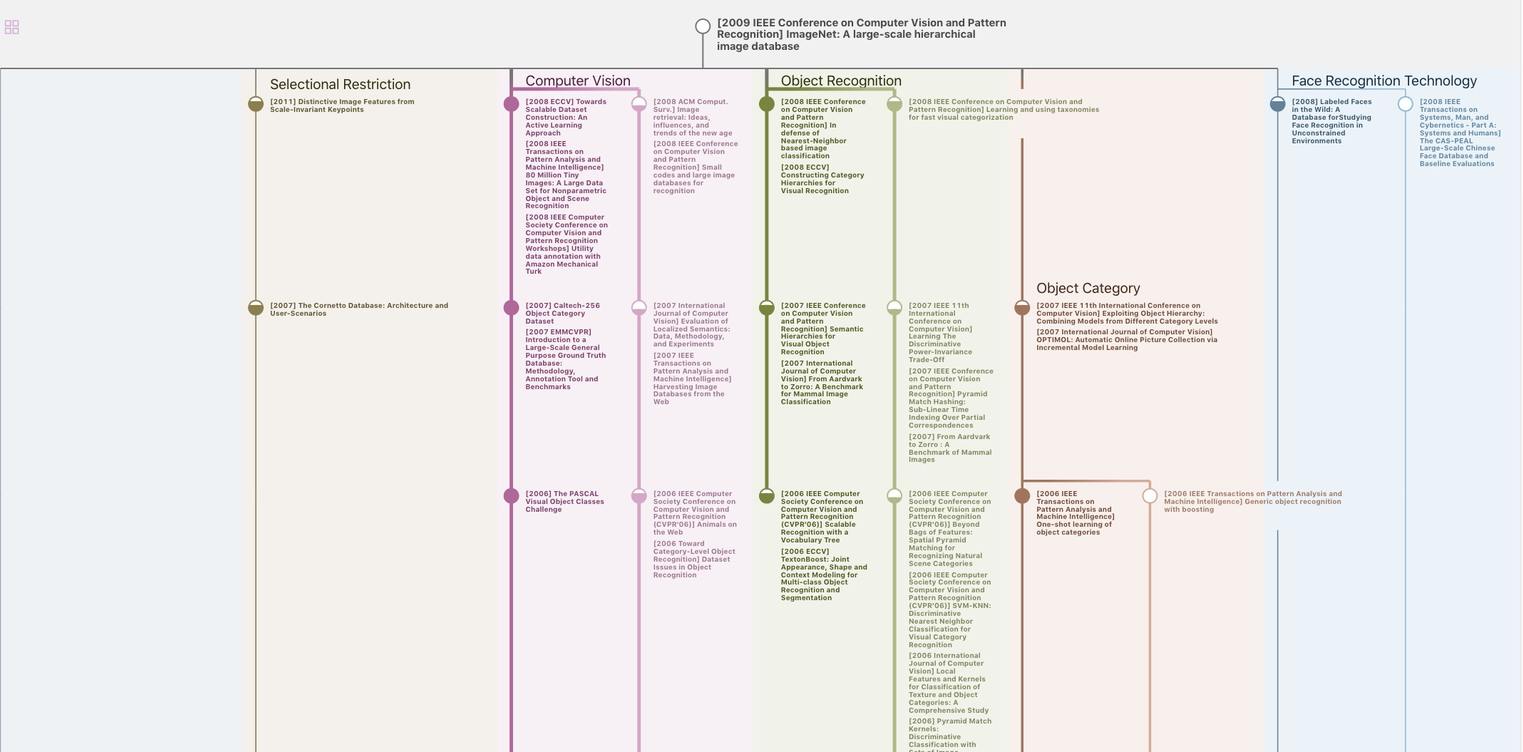
生成溯源树,研究论文发展脉络
Chat Paper
正在生成论文摘要