Favoured Cycles And Other Features Of Anomalous Forecast Uncertainty As Revealed By Time Series Of Tigge Data
QUARTERLY JOURNAL OF THE ROYAL METEOROLOGICAL SOCIETY(2020)
摘要
The time evolution of forecast uncertainty for a given lead time is examined using 9 years of daily ensemble data from the TIGGE archive and a classical time-series approach, with a focus on anomalies in the ensemble variance of T+120h 500 hPa geopotential height averaged over select regions. Spectral analysis suggests the presence of favoured cycles in the anomaly time series, manifested by strong peaks in the spectra at certain periods between 10 and 40 days. Such favoured cycles imply that the time evolution of the forecast uncertainty has some element of predictability. Coincident with these cycles are episodes of two-week to monthly time-scale local trends ("ramp ups" and "ramp downs") in the variance anomaly series. A correlation analysis with typical flow indices is carried out to gauge what physical processes might influence the behaviour of the variance anomaly time series. From the broadest perspective, the Eady index is clearly the most influential of the indices and it is inferred that low-frequency peaks in anomalously positive Eady index presage low-frequency peaks in anomalously positive variance. Considering particular regions and conditioning on season and MJO phase helps reveal the influences of the other indices. There are also indications that anomalously high forecast uncertainty preferentially occurs during specific changes in sign of the indices. Nonetheless, the correlation analysis suggests that much of the behaviour of the variance anomaly time series remains to be explained. Principal component analysis and k-means cluster analysis identify a leading mode of variability in which the variance is jointly elevated or suppressed across the hemisphere (with concentration in the Pacific and Atlantic Oceans storm tracks).
更多查看译文
关键词
ensemble variance, forecast uncertainty, predictability, TIGGE, time series
AI 理解论文
溯源树
样例
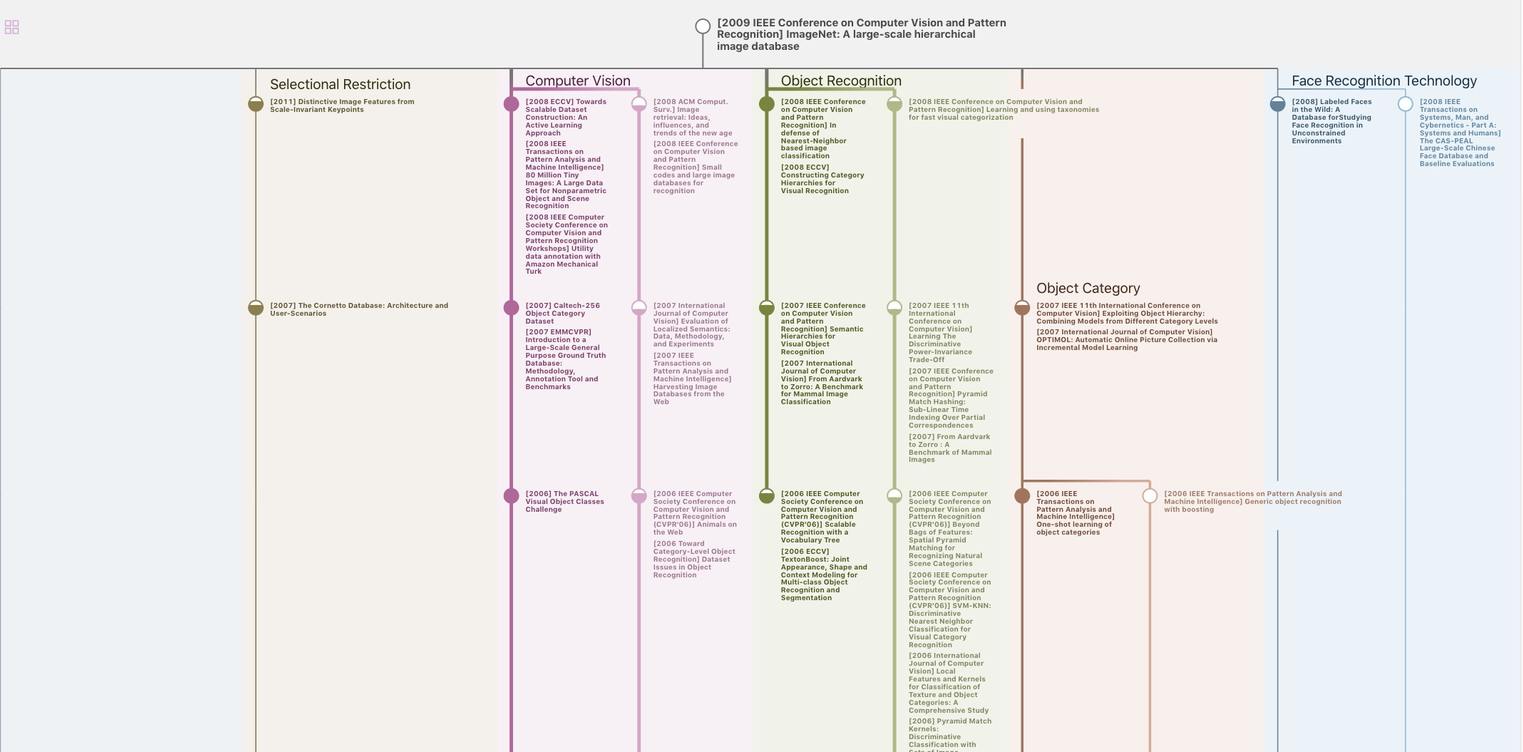
生成溯源树,研究论文发展脉络
Chat Paper
正在生成论文摘要