Improving multilayer perceptron neural network using chaotic grasshopper optimization algorithm to forecast iron ore price volatility
RESOURCES POLICY(2020)
Abstract
Developing an accurate forecasting model for the volatility of iron ore price plays a vital role in future investments and decisions for mining projects and related companies. Viewed from this perspective, this paper proposes a novel model for accurately forecasting monthly iron ore price volatilities. This model integrates chaotic behavior into a recent meta-heuristic method grasshopper optimization algorithm (GOA) to form a new GOA algorithm called chaotic grasshopper optimization algorithm (CGOA), which is used as a trainer to learn the multilayer perceptron neural network (NN). The results of the proposed model (CGOA-NN) are compared to other models, including the conventional grasshopper optimization algorithm for NN (GOA-NN), Particle swarm optimization for NN (PSO-NN), Genetic Algorithm for NN (GA-NN), and classic NN. Empirical results demonstrate the superiority of the hybrid CGOA-NN model over other models. Moreover, the proposed CGOA-NN model demonstrates an improvement in the forecasting accuracy obtained from classic NN, GA-NN, PSO-NN, and GOA-NN models by 60.82%, 32.18%, 16.49%, and 38.71% decrease in mean square error, respectively.
MoreTranslated text
Key words
Chaotic grasshopper optimization algorithm,Multilayer perceptron neural network,Iron ore price volatility,Forecasting,Training neural networks
AI Read Science
Must-Reading Tree
Example
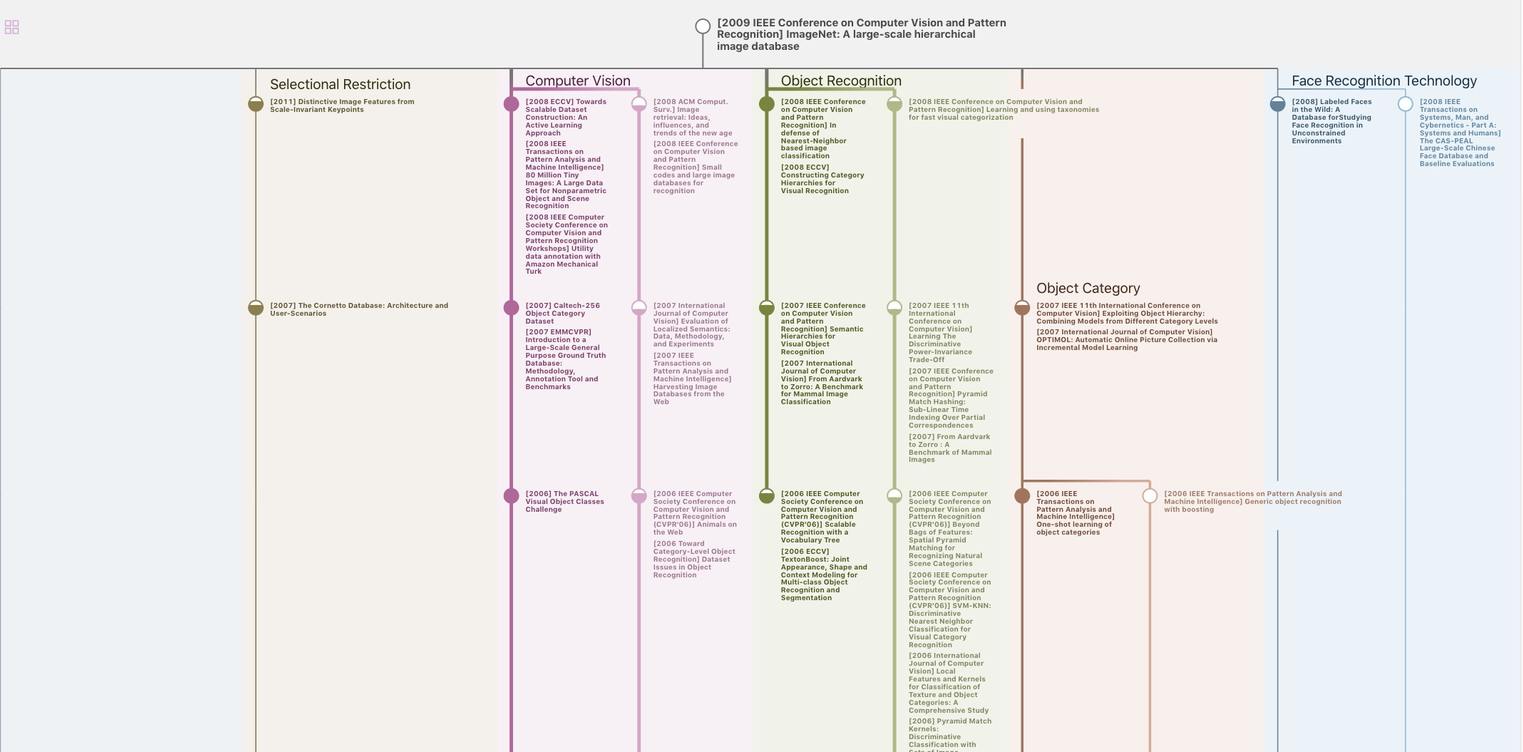
Generate MRT to find the research sequence of this paper
Chat Paper
Summary is being generated by the instructions you defined