An Intelligent Bidding Strategy Based on Model-Free Reinforcement Learning for Real-Time Bidding in Display Advertising
2019 Seventh International Conference on Advanced Cloud and Big Data (CBD)(2019)
Abstract
In recent years, the most important paradigm in online display advertising is real-time bidding (RTB). It allows advertisers to buy individual ad impressions through real-time auctions, to obtain maximum revenue. However, the existing strategies usually bid an ad impression independently, ignoring the impacts of each bid on the overall revenue during the whole ad delivery period. Thus, the recent research suggests that using the reinforcement learning (RL) framework to learn the optimal bidding strategy in RTB, based on both the immediate and future rewards. In this paper, we formulate budget constrained bidding as a model-free reinforcement learning problem, where the state space is presented by the impressions' feature parameters and the auction information, while an action is to set the bidding price. Different from the prior value-based model-free work, which suffers from the convergence problem, we learn the optimal bidding strategy by employing the policy gradient model. Additionally, we design four reward functions according to different auction results and user feedback to the learned bidding strategy more in line with the optimization objectives. We evaluate the performance of the proposed bidding strategy based on a real-world dataset, and the experimental results have demonstrated the superior performance and high efficiency compared to state-of-the-art methods.
MoreTranslated text
Key words
Real-Time Bidding, Bidding Strategy, Reinforcement Learning
AI Read Science
Must-Reading Tree
Example
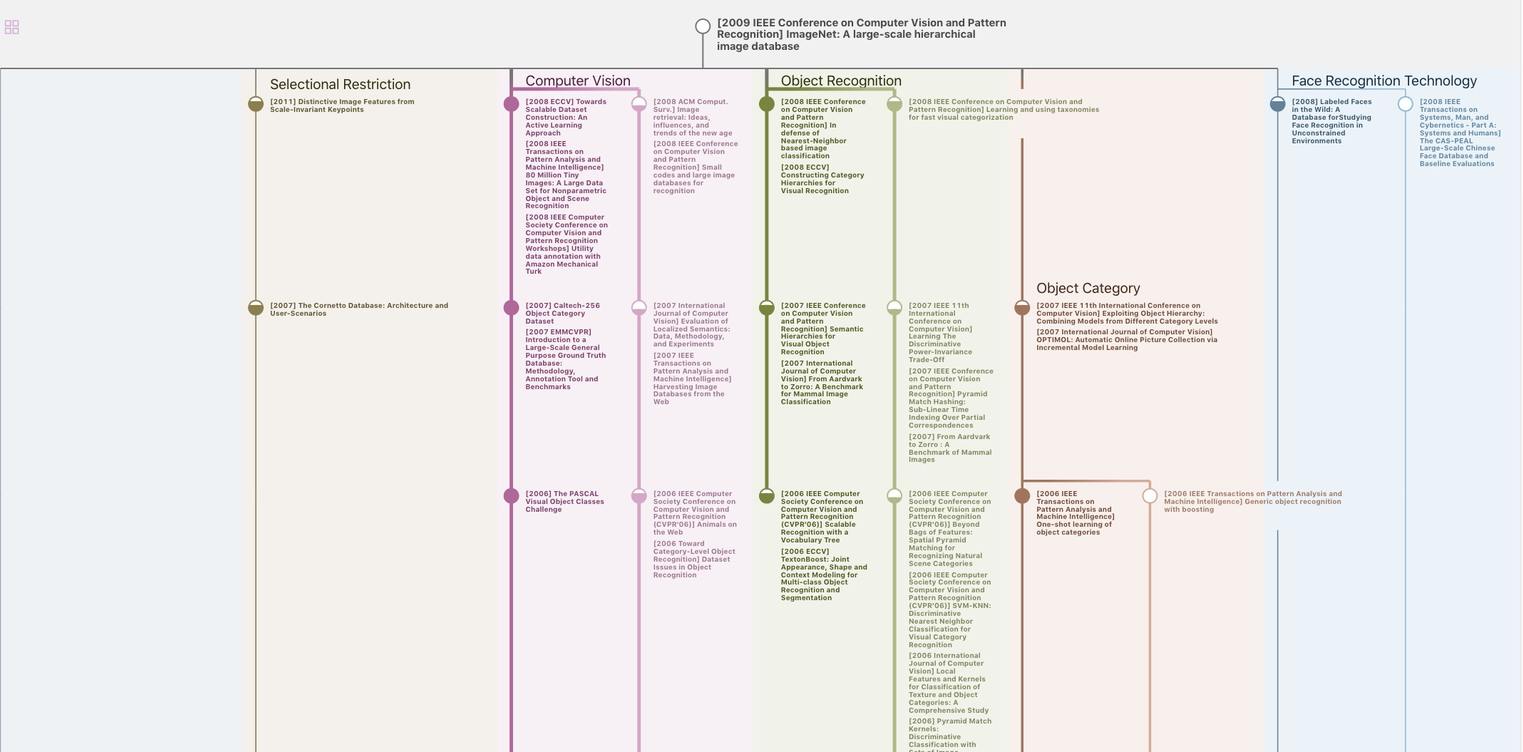
Generate MRT to find the research sequence of this paper
Chat Paper
Summary is being generated by the instructions you defined