Bayesian Approach to Probabilistic Design Space Characterization: A Nested Sampling Strategy
INDUSTRIAL & ENGINEERING CHEMISTRY RESEARCH(2020)
摘要
Quality by design in pharmaceutical manufacturing hinges on computational methods and tools that are capable of accurate quantitative prediction of the design space. This paper investigates Bayesian approaches to design space characterization, which determine a feasibility probability that can be used as a measure of reliability and risk by the practitioner. An adaptation of nested sampling-a Monte Carlo technique introduced to compute Bayesian evidence-is presented. The nested sampling algorithm maintains a given set of live points through regions with increasing probability feasibility until reaching a desired reliability level. It furthermore leverages efficient strategies from Bayesian statistics for generating replacement proposals during the search. Features and advantages of this algorithm are demonstrated by means of a simple numerical example and two industrial case studies. It is shown that nested sampling can outperform conventional Monte Carlo sampling and be competitive with flexibility-based optimization techniques in low-dimensional design space problems. Practical aspects of exploiting the sampled design space to reconstruct a feasibility probability map using machine learning techniques are also discussed and illustrated. Finally, the effectiveness of nested sampling is demonstrated on a higher-dimensional problem, in the presence of a complex dynamic model and significant model uncertainty.
更多查看译文
关键词
probabilistic design space characterization,nested sampling strategy
AI 理解论文
溯源树
样例
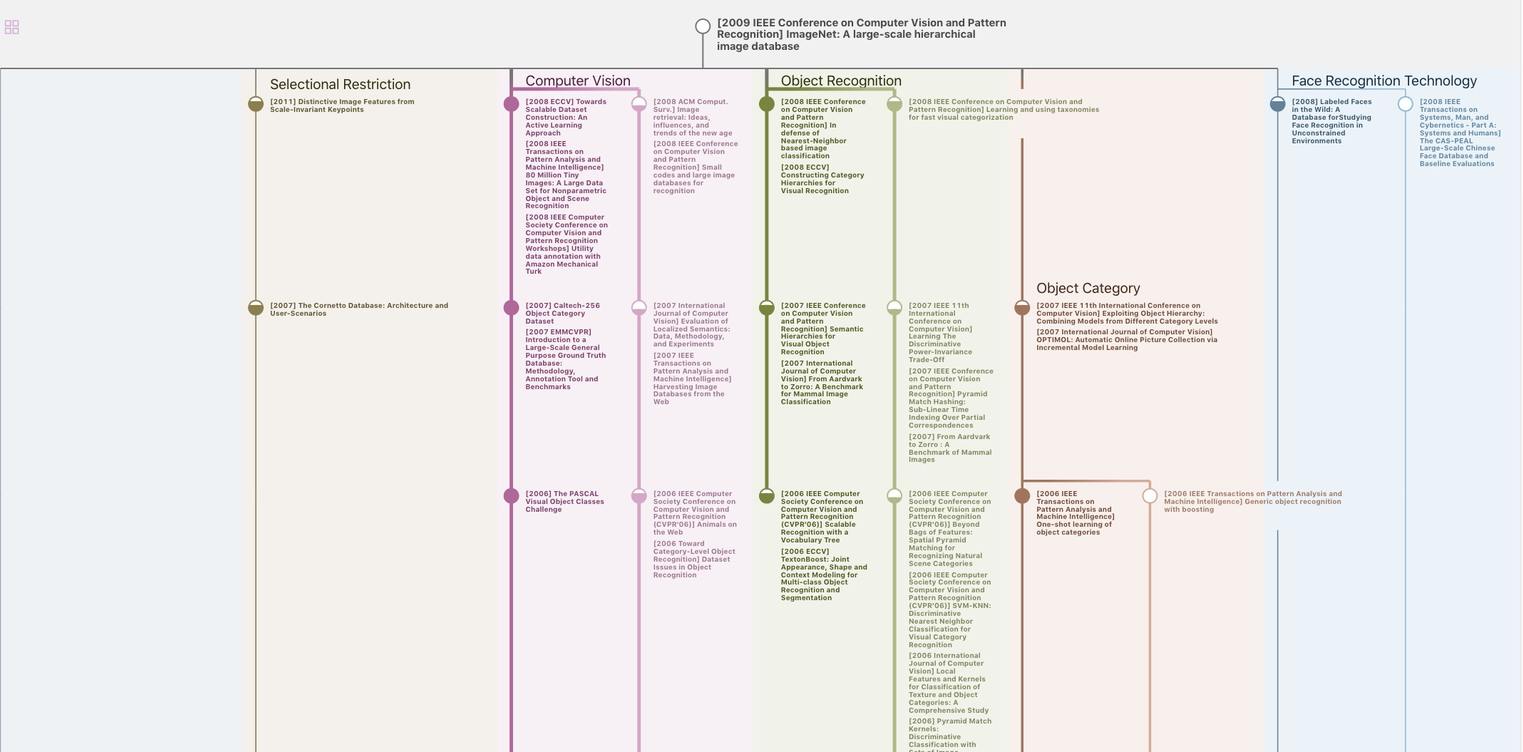
生成溯源树,研究论文发展脉络
Chat Paper
正在生成论文摘要