A 1096fps Hardware Architecture For Fast Training In Object Tracking
2019 IEEE 11TH INTERNATIONAL CONFERENCE ON COMMUNICATION SOFTWARE AND NETWORKS (ICCSN 2019)(2019)
摘要
In recent years, Discriminative Correlation Filter based methods have significantly outperformed the state-of-the art in tracking accuracy. However, the high-complexity training process makes it hard for the tracking task to keep both high accuracy and speed. In this work, the training algorithm is optimized to significantly reduce its computation with acceptable accuracy loss. Then a dedicated hardware is designed to further accelerate the training process with high accuracy. First, time constraints are released to turn serial module into parallel module; Second, the symmetry and sparsity of regularization filter kernel is utilized to reduce 80% computation of regularization convolution; Third, the computation of inner product module in training is reduced by turning complex numbers calculations into real and imaginary numbers calculations respectively. In conclusion, about 24.19% computation of training process is reduced and 4.30% parallel processing time is saved to get a 1.32x hardware resources improvement and 1.05x speedup than the original process. The simulation results show that the throughput of this hardware achieves 1096fps at 250 MHz, which is especially suitable for tracking tasks with high speed and accuracy requirement.
更多查看译文
关键词
discriminative correlation filter, tracking, training, hardware, high speed
AI 理解论文
溯源树
样例
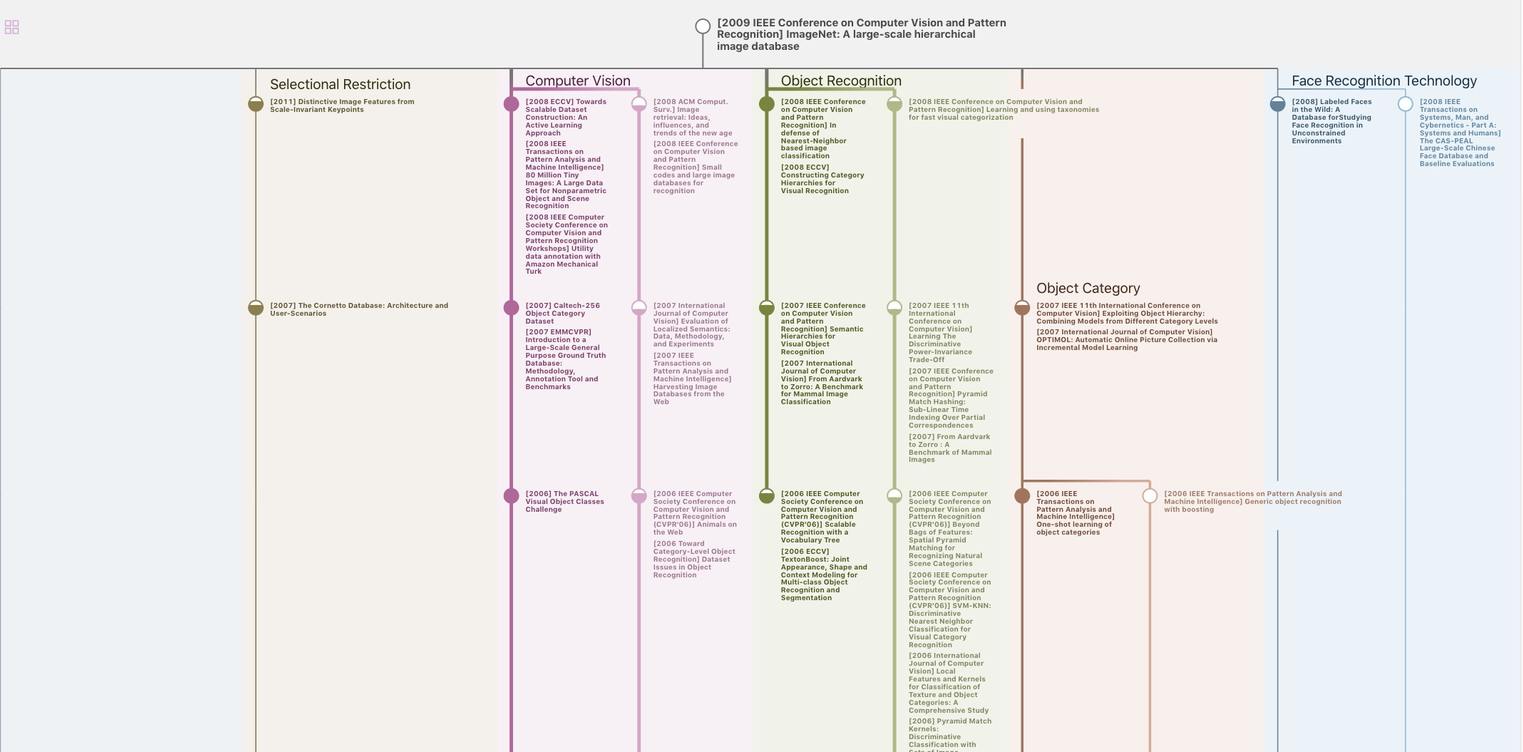
生成溯源树,研究论文发展脉络
Chat Paper
正在生成论文摘要