Pruning 3D Filters for Accelerating 3D ConvNets
IEEE Transactions on Multimedia(2020)
摘要
Many methods have been proposed to accelerate 2D ConvNets by removing redundant parameters. However, few efforts are devoted to the problem of accelerating 3D Convolutional Networks. The 3D ConvNets, which are mainly designed for extracting spatiotemporal features, have been widely used in many video analytics tasks, such as action recognition and scene analysis. In this paper, we focus on accelerating 3D ConvNets for two motivations: (1) Fast video processing techniques are in dire need due to the explosive growth of video data; (2) Compared with individual images, video data consist of consecutively similar frames, thus are inherently more redundant. In this paper, we present a novel algorithm to dramatically accelerate 3D ConvNets by pruning redundant convolutional filters, while preserving the discriminative power of the networks. Specifically, we formulate the filter pruning from 3D ConvNets as a subset selection problem where each filter is regarded as a candidate. Determinantal Point Processes (DPPs) are employed to discriminatively select the filter candidates which are informative and yet diverse. We evaluate our method using two popular 3D networks, C3D and Pseudo-3D, on Sports-1 M dataset for video classification. Extensive experimental results demonstrate both the efficiency and performance advantages of our method. We also show that the proposed method can be easily generalized to 2D ConvNets pruning with promising experimental results on VGGnet and ResNet.
更多查看译文
关键词
Three-dimensional displays,Two dimensional displays,Acceleration,Feature extraction,Task analysis,Computational efficiency,Redundancy
AI 理解论文
溯源树
样例
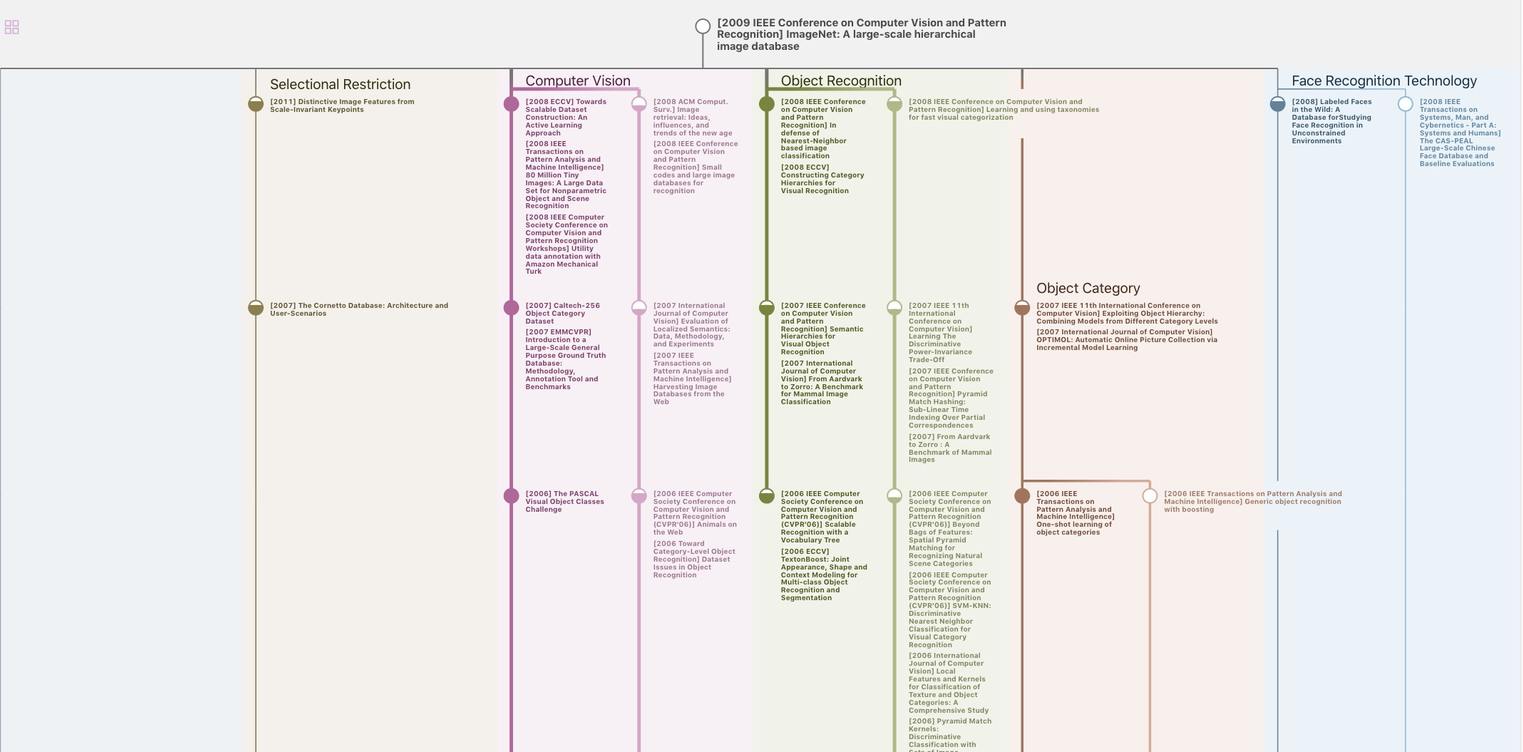
生成溯源树,研究论文发展脉络
Chat Paper
正在生成论文摘要