Bridging Computational Features Toward Multiple Semantic Features with Multi-task Regression: A Study of CT Pulmonary Nodules.
Medical Image Computing and Computer-Assisted Intervention – MICCAI 2016: 19th International Conference, Athens, Greece, October 17-21, 2016, Proceedings, Part II(2016)
摘要
The gap between the computational and semantic features is the one of major factors that bottlenecks the computer-aided diagnosis (CAD) performance from clinical usage. To bridge such gap, we propose to utilize the multi-task regression (MTR) scheme that leverages heterogeneous computational features derived from deep learning models of stacked denoising autoencoder (SDAE) and convolutional neural network (CNN) as well as Haar-like features to approach 8 semantic features of lung CT nodules. We regard that there may exist relations among the semantic features of “spiculation”, “texture”, “margin”, etc., that can be exploited with the multi-task learning technique. The Lung Imaging Database Consortium (LIDC) data is adopted for the rich annotations, where nodules were quantitatively rated for the semantic features from many radiologists. By treating each semantic feature as a task, the MTR selects and regresses the heterogeneous computational features toward the radiologists’ ratings with 10 fold cross-validation evaluation on the randomly selected LIDC 1400 nodules. The experimental results suggest that the predicted semantic scores from MTR are closer to the radiologists’ rating than the predicted scores from single-task LASSO and elastic net regression methods. The proposed semantic scoring scheme may provide richer quantitative assessments of nodules for deeper analysis and support more sophisticated clinical content retrieval in medical databases.
更多查看译文
AI 理解论文
溯源树
样例
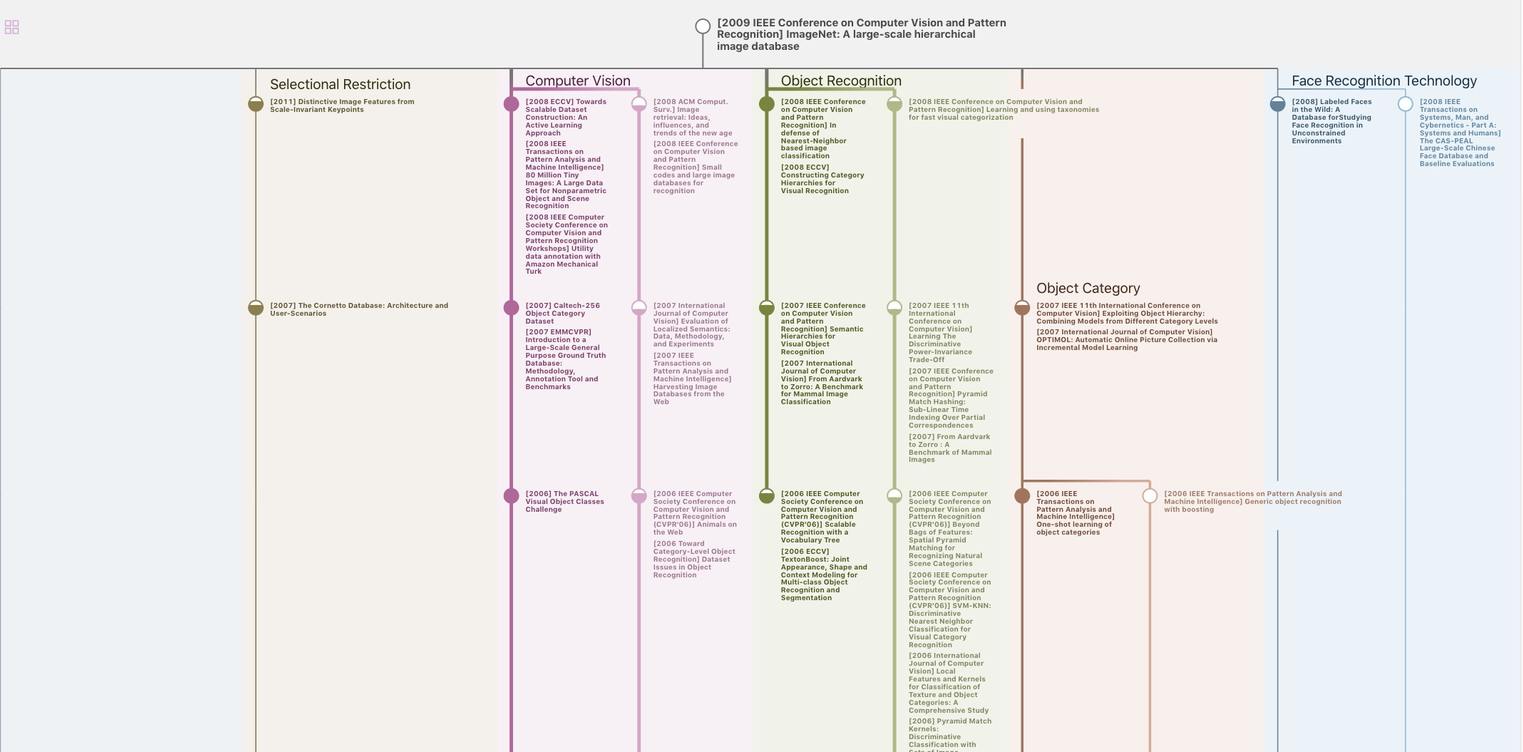
生成溯源树,研究论文发展脉络
Chat Paper
正在生成论文摘要