Optimization of Robust Model Predictive Controllers for Second-Order Biological Processes Using Markov Chain Monte Carlo Techniques
INDUSTRIAL & ENGINEERING CHEMISTRY RESEARCH(2019)
摘要
For biological fermentation processes in vaccine manufacturing, the development of a robust controller is essential to manage uncertainty in the process model and external disturbances in the control setup. A possible control strategy for this purpose is to use model predictive control (MPC), which utilizes a process model to compute a sequence of control moves via a receding horizon approach. To determine each control move, an MPC controller solves an optimization problem which can be modified to a min-max problem in order to achieve robustness. Extensive work has been undertaken to develop and test the min-max approach to obtain robust MPC; however, there is a paucity of control literature that has focused on the offline optimization of MPC control parameters (e.g., MPC horizons, control weights) to achieve robustness. To address this issue, a model predictive controller for a second-order process model representing a microbial fermentation process is developed. Uncertainty is simulated by generating 500 mismatched process models based on observed experimental data. The developed MPC controller is then applied to these process models, resulting in 500 scenarios of plant and nominal model mismatch, from which the average control performance and variation in control performance are computed. Markov Chain Monte Carlo (MCMC) techniques are successfully used to find the optimal settings that maximize control performance and minimize variation in control performance in response to step changes in both the set-point and the input disturbance. This novel approach may serve as a foundation for future MPC tuning studies which seek to determine the optimal combination of MPC settings that result in robust control.
更多查看译文
AI 理解论文
溯源树
样例
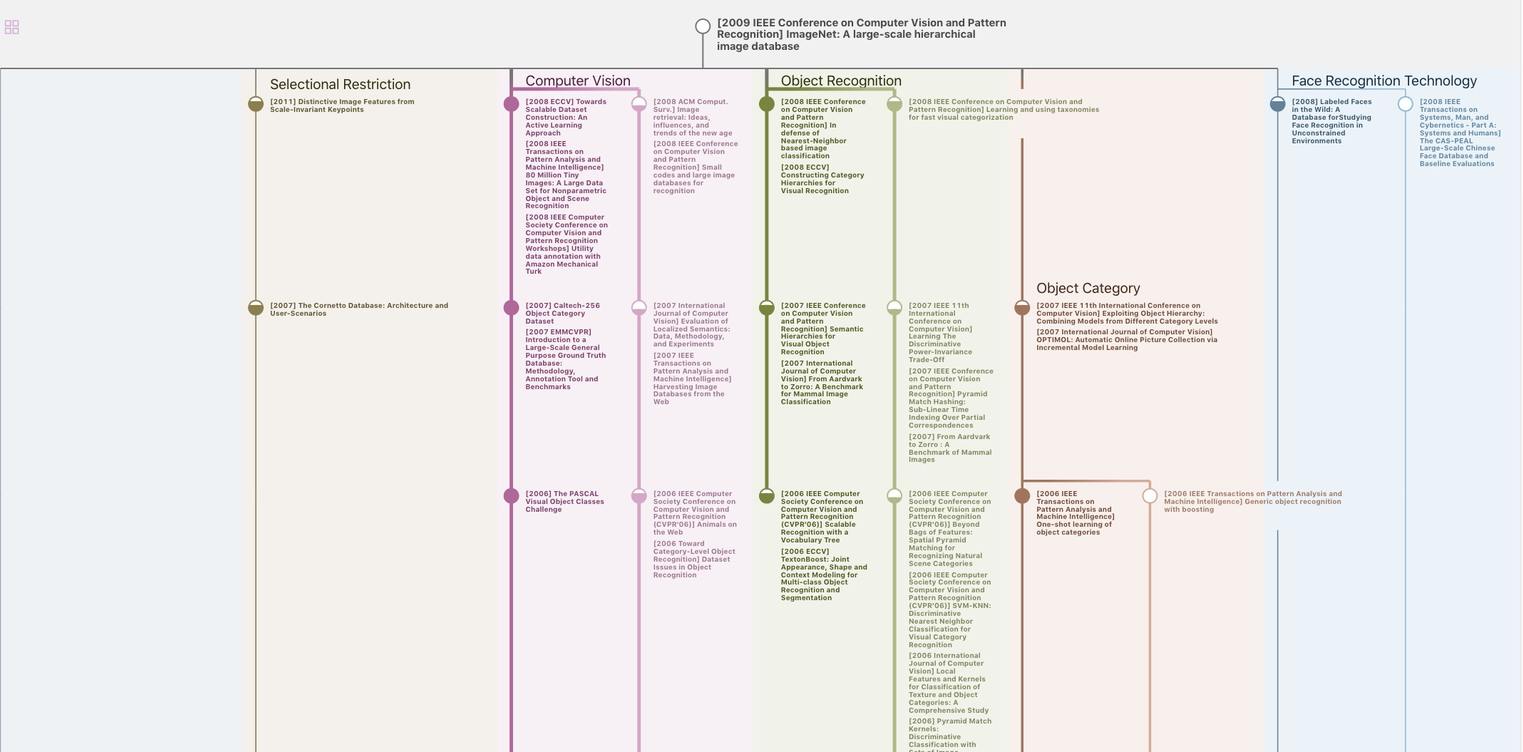
生成溯源树,研究论文发展脉络
Chat Paper
正在生成论文摘要