A Machine Learning Approach to Crater Classification from Topographic Data
REMOTE SENSING(2019)
摘要
Craters contain important information on geological history and have been widely used for dating absolute age and reconstructing impact history. The impact process results in a lot of ejected fragments and these fragments may form secondary craters. Studies on distinguishing primary craters from secondary craters are helpful in improving the accuracy of crater dating. However, previous studies about distinguishing primary craters from secondary craters were either conducted by manual identification or used approaches mainly concerning crater spatial distribution, which are time-consuming or have low accuracy. This paper presents a machine learning approach to distinguish primary craters from secondary craters. First, samples used for training and testing were identified and unified. The whole dataset contained 1032 primary craters and 4041 secondary craters. Then, considering the differences between primary and secondary craters, features mainly related to crater shape, depth, and density were calculated. Finally, a random forest classifier was trained and tested. This approach showed a favorable performance. The accuracy and F1-score for fivefold cross-validation were 0.939 and 0.839, respectively. The proposed machine learning approach enables an automated method of distinguishing primary craters from secondary craters, which results in better performance.
更多查看译文
关键词
moon,distinguish primary craters from secondary craters,machine learning,crater characteristics
AI 理解论文
溯源树
样例
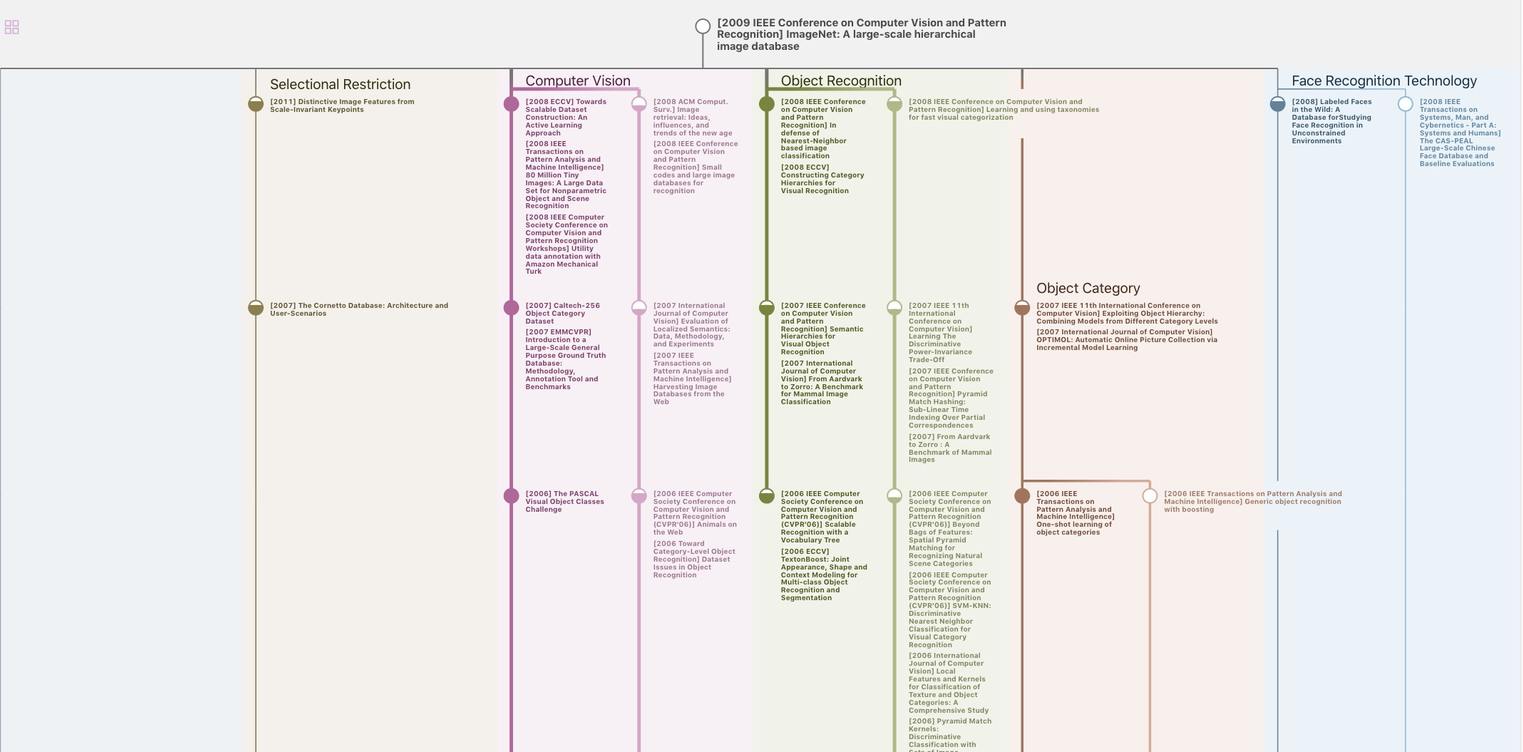
生成溯源树,研究论文发展脉络
Chat Paper
正在生成论文摘要