NIMG-30. REPRODUCIBLE RADIOMIC MAPPING OF TUMOR CELL DENSITY BY MACHINE LEARNING AND DOMAIN ADAPTATION
NEURO-ONCOLOGY(2019)
摘要
Abstract BACKGROUND An important challenge in radiomics research is reproducibility. Images are collected on different image scanners and protocols, which introduces significant variability even for the same type of image across institutions. In the present proof-of-concept study, we address the reproducibility issue by using domain adaptation – an algorithm that transforms the radiomic features of each new patient to align with the distribution of features formed by the patient samples in a training set. METHOD Our dataset included 18 patients in training with a total of 82 biopsy sample. The pathological tumor cell density was available for each sample. Radiomic (statistical + texture) features were extracted from the region of six image contrasts locally matched with each biopsy sample. A Gaussian Process (GP) classifier was built to predict tumor cell density using radiomic features. Another 6 patients were used to test the training model. These patients had a total of 31 biopsy samples. The images of each test patient were purposely normalized using a different approach, i.e., using the CSF instead of the whole brain as the reference. This was to mimic the practical scenario of image source discrepancy between different patients. Domain adaptation was applied to each test patient. RESULTS Among the 18 training patients, the leave-one-patient-out cross validation accuracy is 0.81 AUC, 0.78 sensitivity, and 0.83 specificity. When the trained model was applied to the 6 test patients (purposely normalized using a different approach than that of the training data), the accuracy dramatically reduced to 0.39 AUC, 0.08 sensitivity, and 0.61 specificity. After using domain adaption, the accuracy improved to 0.68 AUC, 0.62 sensitivity, and 0.72 specificity. CONCLUSION We provide candidate enabling tools to address reproducibility in radiomics models by using domain adaption algorithms to account for discrepancy of the images between different patients.
更多查看译文
关键词
reproducible radiomic mapping
AI 理解论文
溯源树
样例
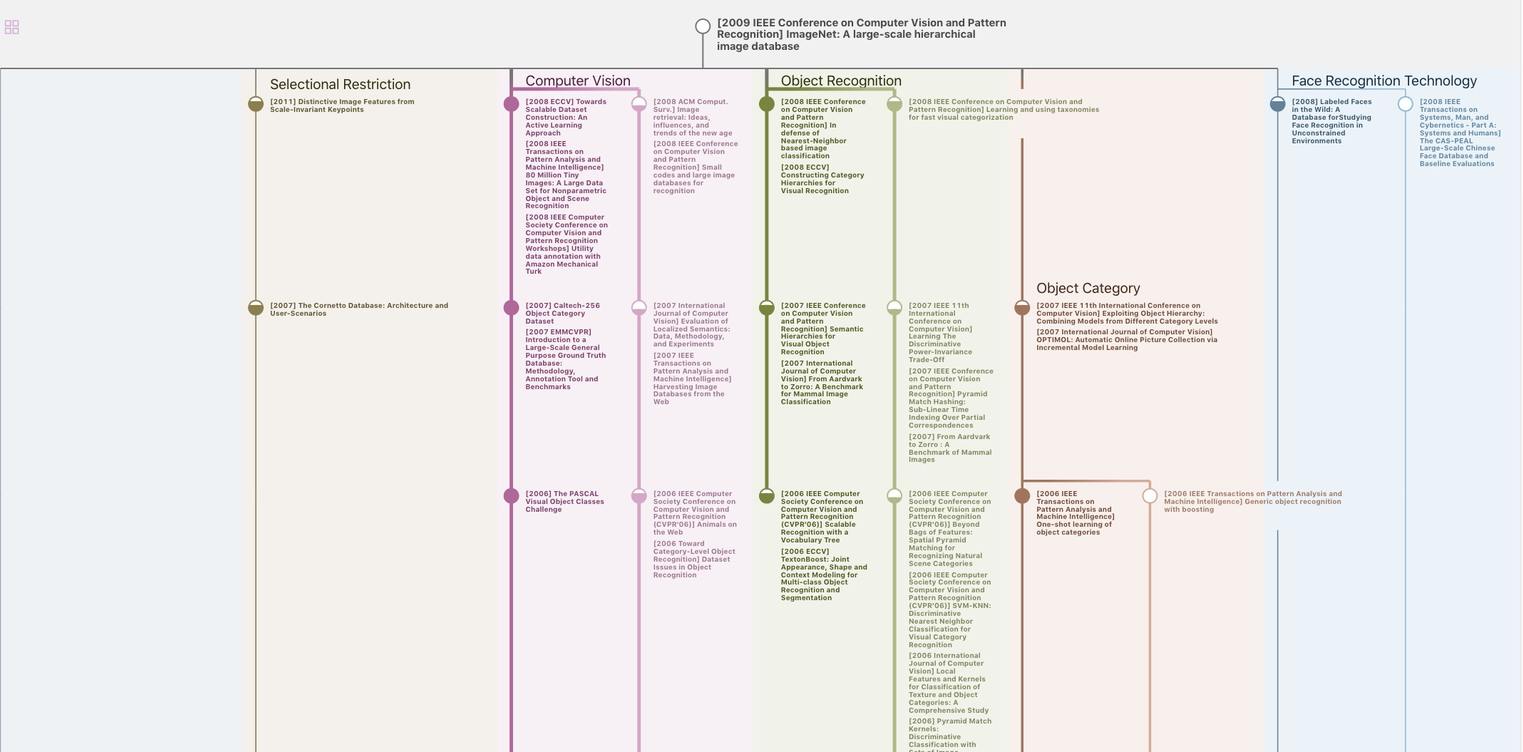
生成溯源树,研究论文发展脉络
Chat Paper
正在生成论文摘要