Enhancing The Accuracies Of Age Estimation With Heterogeneous Databases Using Modified Cyclegan
IEEE ACCESS(2019)
摘要
Age estimation using face images has been widely employed across various fields. Because the characteristics of face images usually vary greatly depending on race, camera type, lighting, and other environmental factors, the recognition ability of untrained heterogeneous face image databases is not accurate by previous methods. Therefore, various attempts have been made where different heterogeneous databases were combined to enable training; however, the training time is extended and diverse environmental variables in databases cannot be sufficiently trained. To address these issues, this study proposes modified cycle-consistent generative adversarial network (CycleGAN) that generates an even distribution of heterogeneous face data, and an age-estimation method that is effective for heterogeneous data based on comparative CNN for age estimation (CCNNAE). In addition, we propose the method of reducing the errors caused by image transformation in modified CycleGAN through adaptive selection between transformed and original face images for the input to CCNNAE, which is based on age similarity between the transformed face image and the original face image. Experiments with two open databases, MORPH and MegaAge databases showed that our method outperformed the state-of-the art methods.
更多查看译文
关键词
Age estimation, heterogeneous database, modified CycleGAN, CCNNAE
AI 理解论文
溯源树
样例
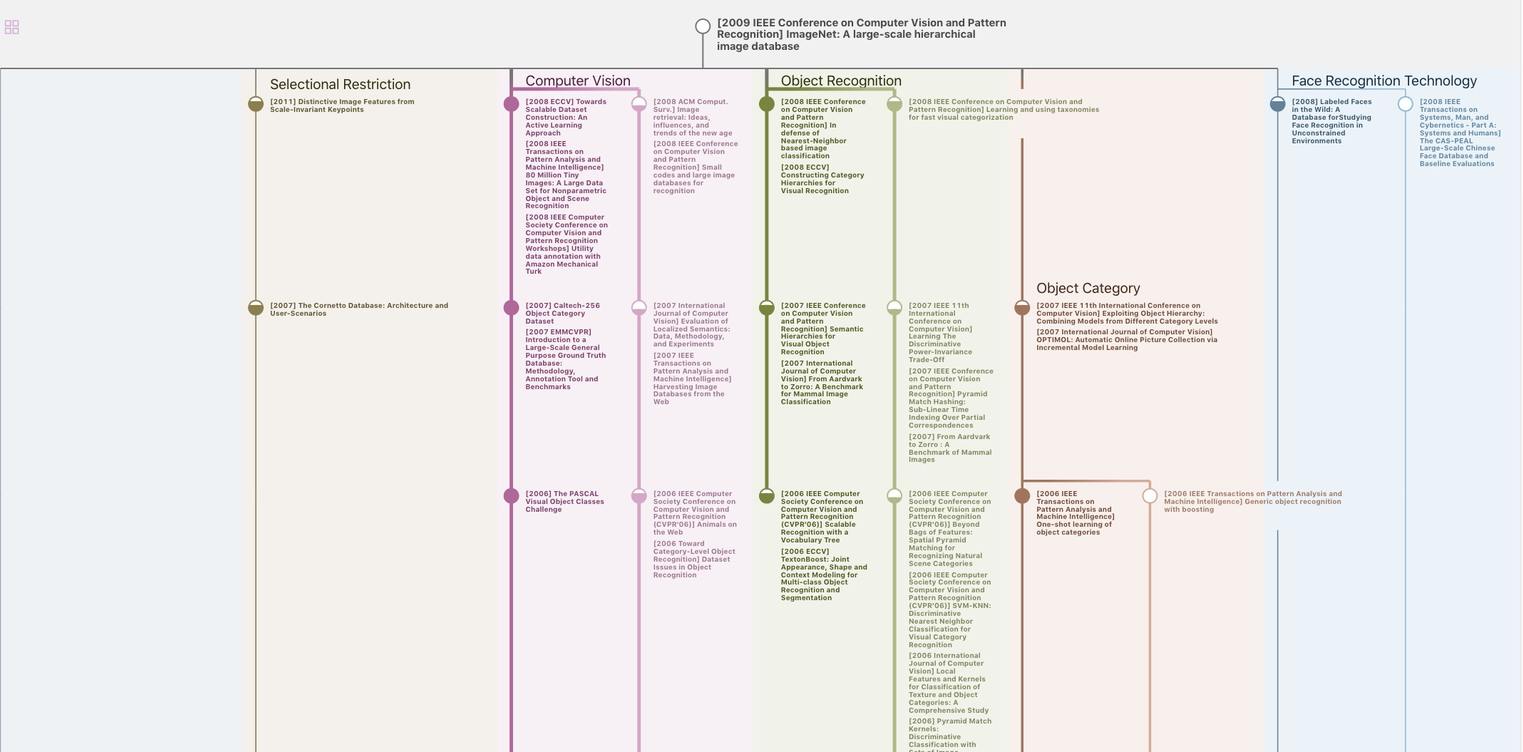
生成溯源树,研究论文发展脉络
Chat Paper
正在生成论文摘要