Machine learning challenges in bat biosonar
mag(2019)
摘要
Many bat species thrive in complex natural environments where their biosonar pulses trigger echoes with complex, unpredictable waveforms. In most cases, it remains unknown how the bats obtain sensory information they need from such clutter echoes. Machine learning methods that can extract relationships from large data sets could have a transformative impact on these research challenges. In particular, these methods hold considerable potential for answering the following questions: (i) What is the nature of biosonar echoes from complex environments; (ii) how can low-level navigation tasks such as contour-following and passageway finding be accomplished; (iii) what are acoustic landmarks for navigation and habitat selection; (iv) which clues exist for identifying prey in clutter; and (v) how does biosonar-based guidance in dense vegetation work at the system level? Machine learning methods are well suited for identifying models of echoes from complex vegetation that can be used to create large synthetic data sets with a known ground truth. Similarly, they can be employed to analyze (e.g., cluster) large physical echo datasets and to discover features associated with specific biosonar sensing tasks. Hence, machine learning could lead to the discovery of novel signal features to enable successful operation in much more complex environments.
更多查看译文
关键词
Social Learning
AI 理解论文
溯源树
样例
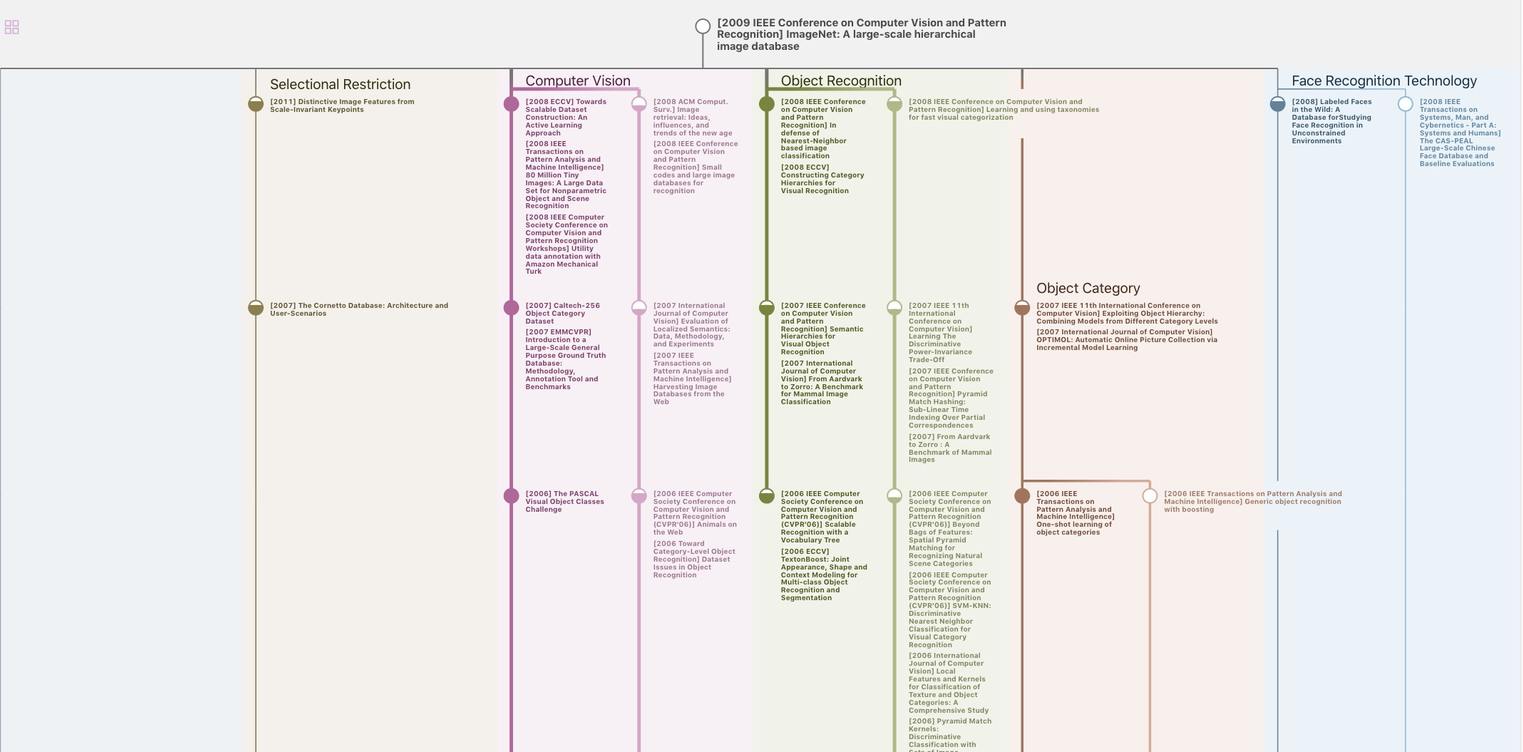
生成溯源树,研究论文发展脉络
Chat Paper
正在生成论文摘要