TMOD-14. RADIOGRAPHIC, STIMULATED RAMAN HISTOLOGIC, AND MULTIPLEXED RNA-SEQUENCING ANALYSIS OF POST-TREATMENT RECURRENT HIGH-GRADE GLIOMAS
NEURO-ONCOLOGY(2019)
Abstract
Abstract High-grade gliomas (HGGs) nearly always recur after standard initial treatment, and the resulting mixture of recurrent tumor and treatment-induced reactive changes presents major diagnostic challenges. Anatomical imaging, such as MRI, cannot adequately distinguish progressive disease from treatment effect (pseudo-progression). Furthermore, there is marked intra-tumoral heterogeneity, such that some areas of a tumor may demonstrate necrotic treatment effect and others frank recurrence. Due to this difficulty reliably differentiating between these two clinical findings, analytic methods using multiple modalities are necessary to further our understanding of this disease process. To this end, we sought to correlate radiographic, histopathologic and molecular features of surgically sampled post-treatment suspected recurrence to identify markers distinguishing tumor growth from treatment effect. We performed Stimulated Raman Histology (SRH) imaging and highly multiplexed RNA-sequencing (PLATE-seq) on 84 MRI-localized biopsies from 39 patients with clinically suspected recurrent HGG. The SRH images were classified as recurrent tumor or gliotic/reactive tissue using a convolutional neural network trained on an independent cohort including a large set of recurrent HGG, and an automated cell-counting algorithm was used to quantify cellularity from the SRH image of each sample. Differential gene expression analysis of the PLATE-seq data was used to identify gene sets that distinguish recurrent tumor from treatment effect, and single sample gene set variation analysis (GSVA) was used to further assess the molecular and cellular composition of each MRI-localized sample. The histopathologic and molecular features of each sample were also correlated with the MRI features of the corresponding biopsy sites, and this data is currently being used to train machine learning models that predict the distribution of recurrent tumor and treatment-induced reactive changes within a patient’s radiographic lesion. These predictive radiomic models will help to guide neurosurgical sampling, and improve our ability to monitor glioma progression and response to therapy.
MoreTranslated text
Key words
gliomas,stimulated raman histologic,rna-sequencing,post-treatment,high-grade
AI Read Science
Must-Reading Tree
Example
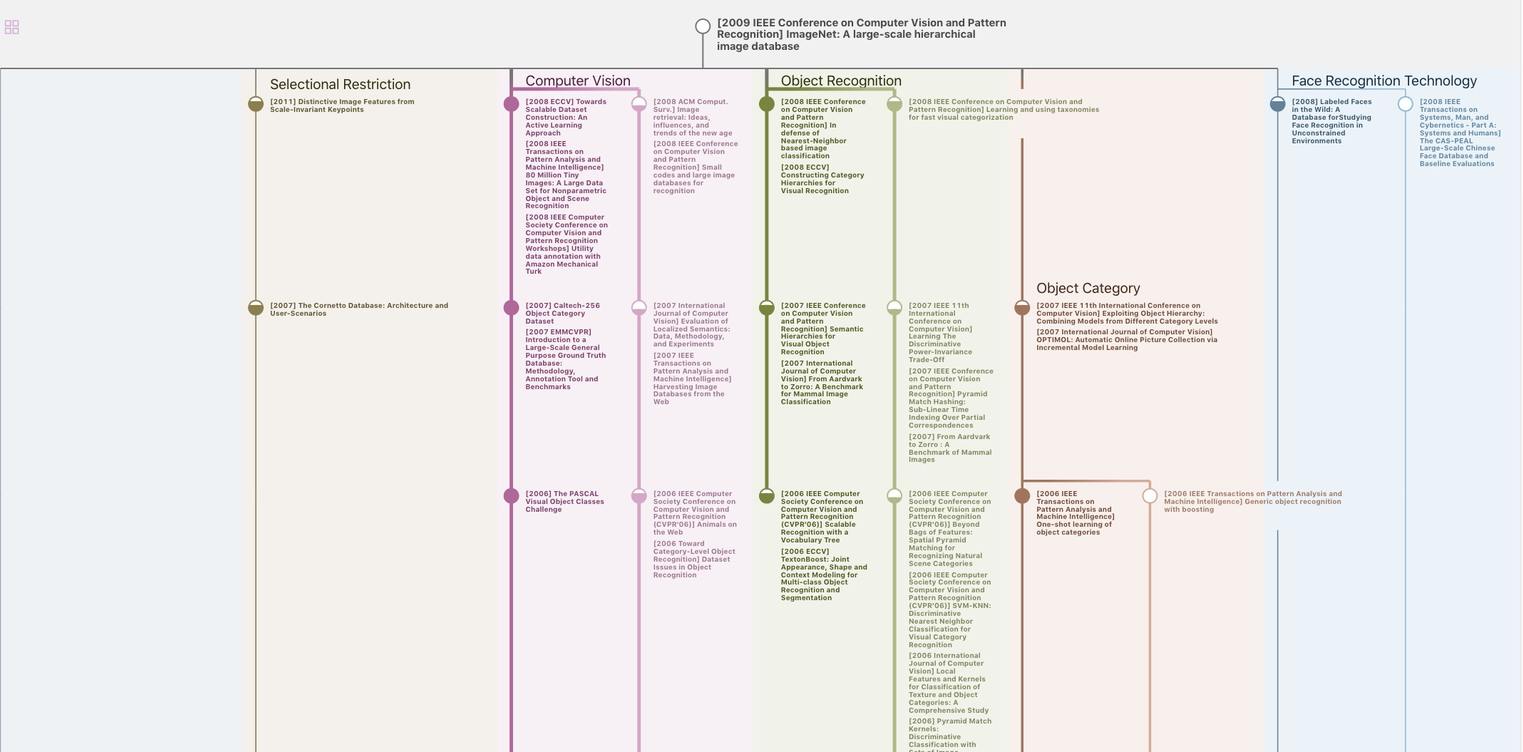
Generate MRT to find the research sequence of this paper
Chat Paper
Summary is being generated by the instructions you defined