Filtering approaches for online train motion estimation with onboard power measurements
Periodicals(2020)
摘要
AbstractAbstractFuture railway systems will need the support of more precise and reliable information on train motion during operation, to fully exploit the potential of new technologies. Train motion information are here intended as time series of train state characteristics, that is, position, speed, and acceleration. This paper investigates the use of two different filtering formulations for better online estimations of train state along the track. Specifically, extended Kalman filters (EKFs) and particle filters (PFs) are used to fuse kinematic measurements collected by means of Global Navigation Satellite Systems (GNSSs) with information collected by the trains on the used tractive power. The EKFs need linearity assumptions and are based on statistical evaluations of the train state, the PFs are instead conceived for nonlinear models and they are based on probability functions related to train state measurements and estimates. A set of experiments with real data of trains operating on a Swiss line is presented to analyze the capabilities of these two filters when applied to rail operation in non‐urban environments. We discuss the extent by which the proposed filtering approaches match the latest technical requirements for implementation. We expect such an enhanced train motion estimation to enable reliable and continuous positioning, available at train level and at the traffic control center, while trackside equipment will be gradually reduced.
更多查看译文
AI 理解论文
溯源树
样例
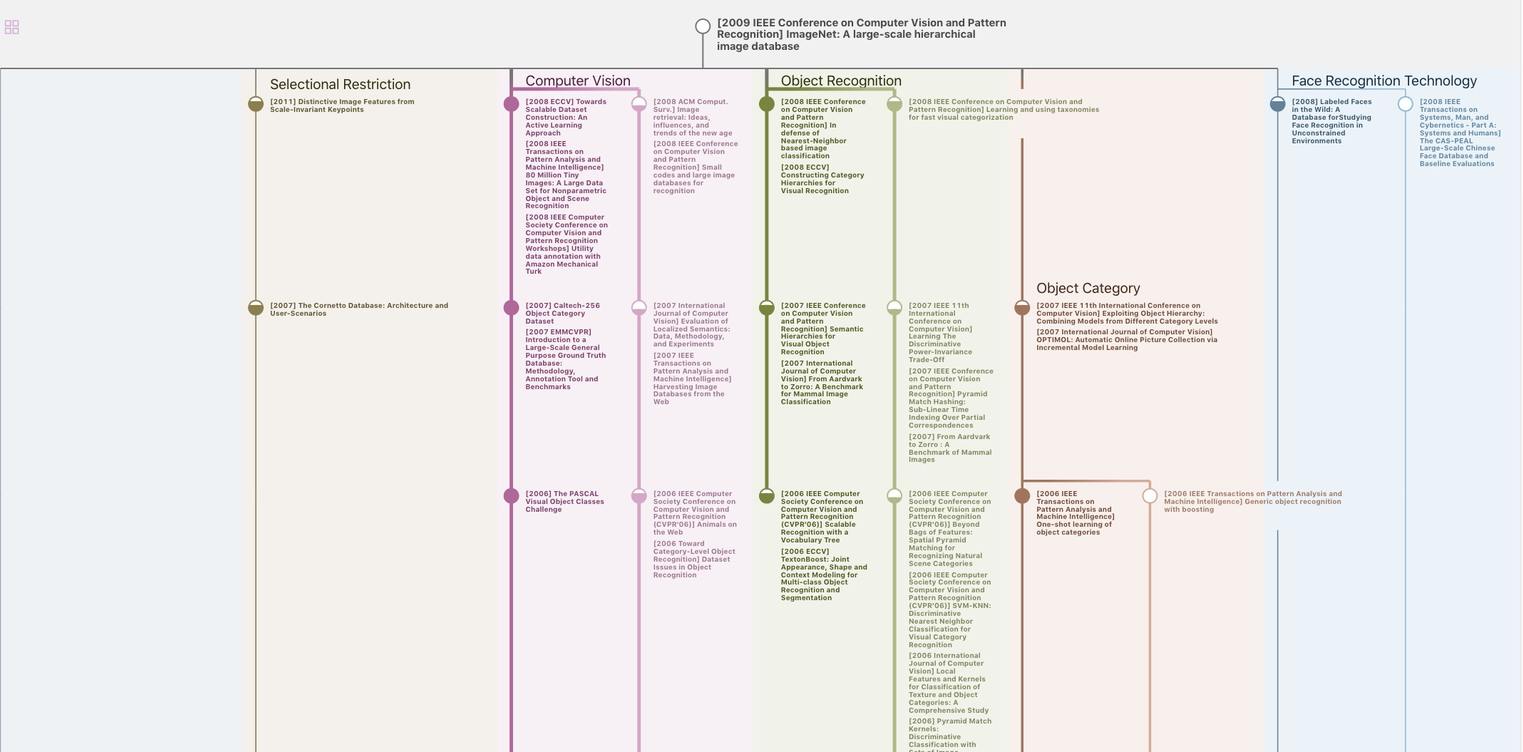
生成溯源树,研究论文发展脉络
Chat Paper
正在生成论文摘要