Feature uncertainty bounds for explicit feature maps and large robust nonlinear SVM classifiers
Annals of Mathematics and Artificial Intelligence(2019)
摘要
We consider the binary classification problem when data are large and subject to unknown but bounded uncertainties. We address the problem by formulating the nonlinear support vector machine training problem with robust optimization. To do so, we analyze and propose two bounding schemes for uncertainties associated to random approximate features in low dimensional spaces. The proposed bound calculations are based on Random Fourier Features and the Nyström methods. Numerical experiments are conducted to illustrate the benefit of the technique. We also emphasize the decomposable structure of the proposed robust nonlinear formulation that allows the use of efficient stochastic approximation techniques when datasets are large.
更多查看译文
关键词
Robust classification,Random Fourier features,Nyström method,Robust optimization,Support vector machines,Machine learning
AI 理解论文
溯源树
样例
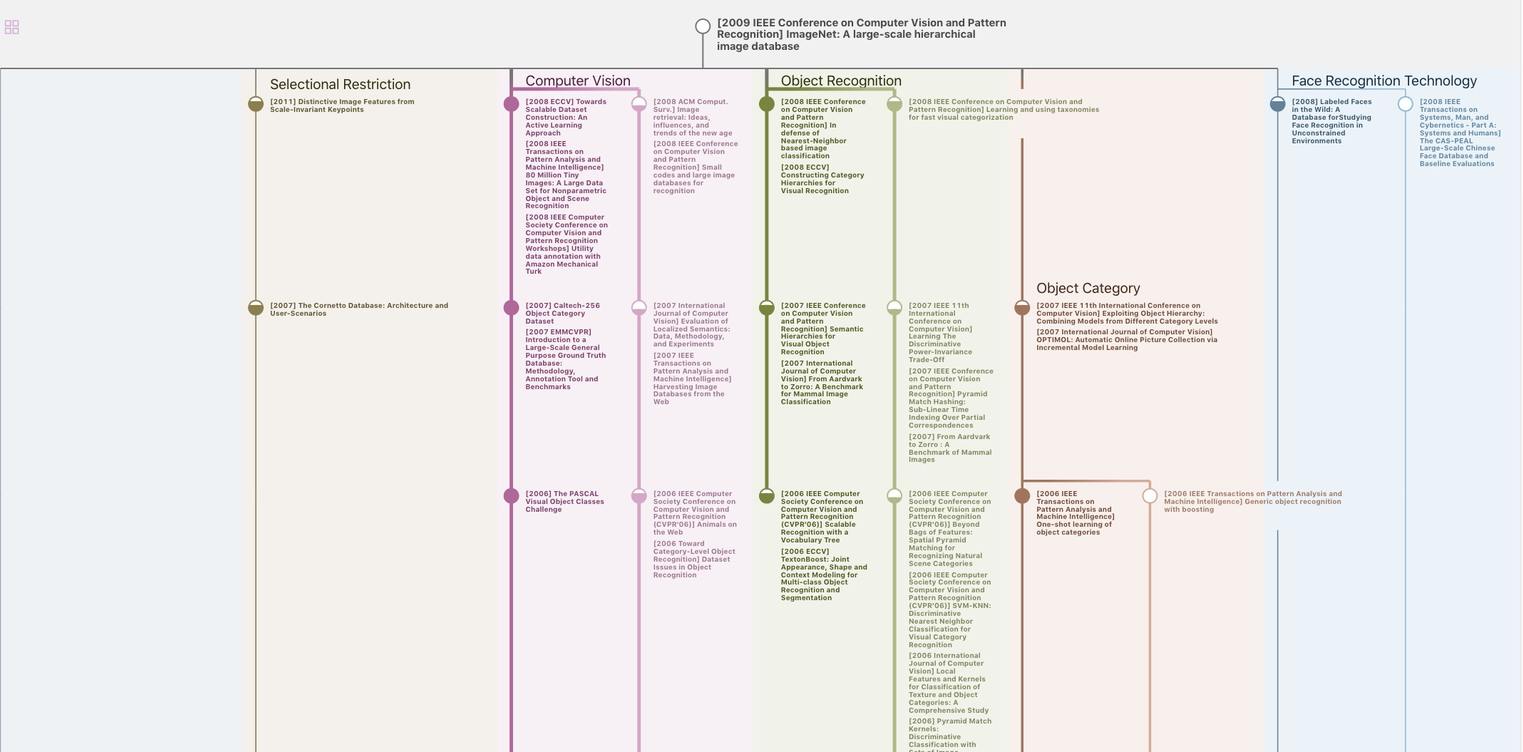
生成溯源树,研究论文发展脉络
Chat Paper
正在生成论文摘要