Battery Model Parameterization using Manufacturer Datasheet and Field Measurement for Real-time HIL Applications
IEEE Transactions on Smart Grid(2020)
摘要
This paper presents a novel battery model parameterization method using actual field measurement and manufacturer datasheet for real-time hardware-in-the-loop (HIL) applications. It is critical that real-time HIL models can accurately reproduce field test results so that tests can be conducted on HIL testbeds instead of in the field. In the past, numerical heuristic optimization algorithms were often used to derive parameters for battery models. However, the deterministic algorithms often reach a locally optimal solution and stochastic heuristic searching strategies suffer from low searching efficiency. Therefore, in this paper, we propose a global-local searching enhanced genetic algorithm (GL-SEGA). By applying the generalized opposition-based learning mechanism, GL-SEGA can efficiently explore the global solution space. By using the trust-region-reflective method to perform the local search, the GL-SEGA can improve the accuracy and convergence in its local exploitations. Field measurements and manufactory datasheets are used to test and validate the accuracy and robustness of the GL-SEGA algorithm.
更多查看译文
关键词
Battery model,genetic algorithm,opposition based learning,parameterization,trust-region-reflective
AI 理解论文
溯源树
样例
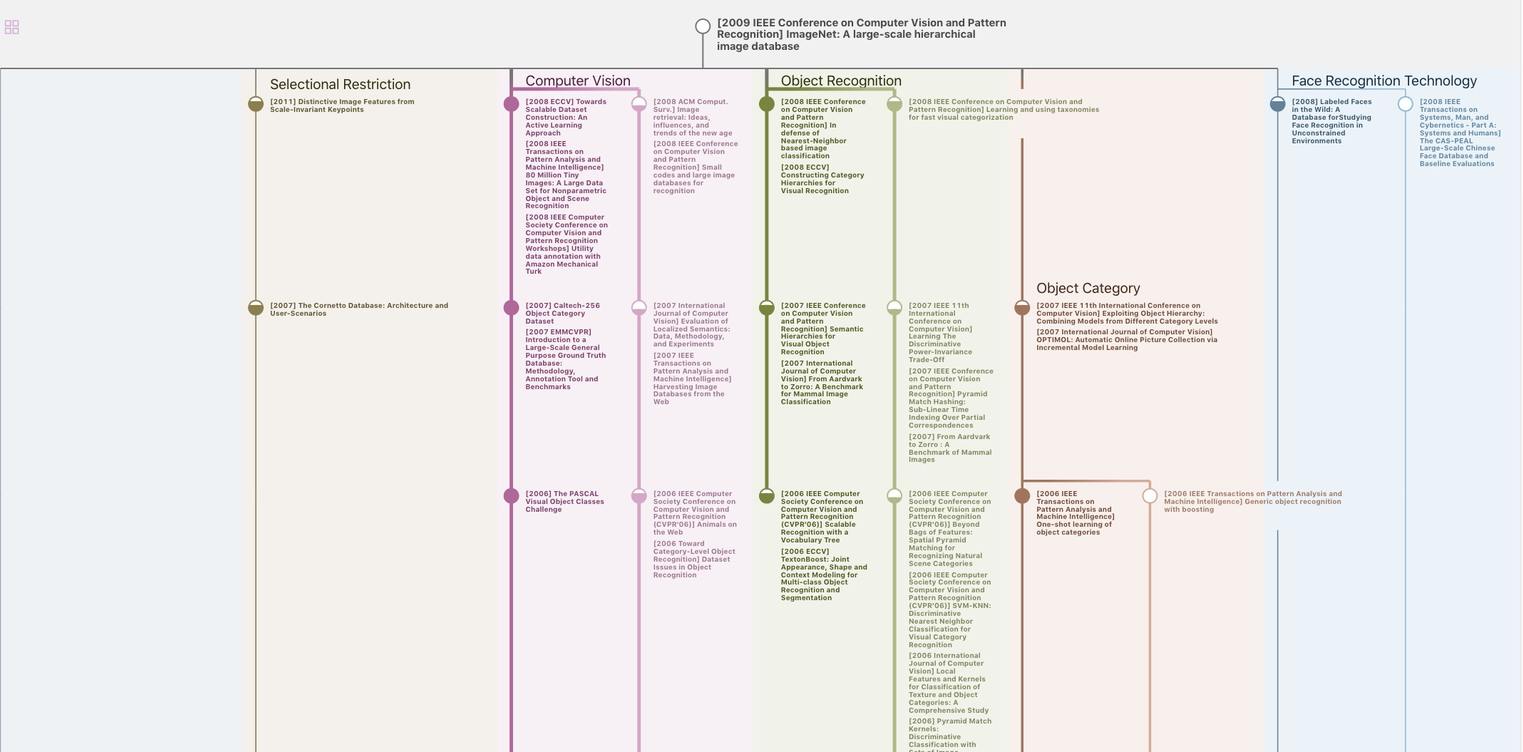
生成溯源树,研究论文发展脉络
Chat Paper
正在生成论文摘要