Scalable Data Analytics Market Basket Model For Transactional Data Streams
INTERNATIONAL JOURNAL OF ADVANCED COMPUTER SCIENCE AND APPLICATIONS(2019)
摘要
Transactional data streams (TDS) are incremental in nature thus, the process of mining is complicated. Such complications arise from challenges such as infinite length, feature evolution, concept evolution and concept drift. Tracking concept drift challenge is very difficult, thus very important for Market Basket Analysis (MBA) applications. Hence, the need for a strategy to accurately determine the suitability of item pairs within the available billions of pairs to solve concept drift chalenge of TDS in MBA. In this work, a Scalable Data Analytics Market Basket Model (SDAMBM) that handles concept drift issues in MBA was developed. Transactional data of 1,112,000 were extracted from a grocery store using Extraction, Transformation and Loading approach and 556,000 instances of the data were simulated from a cloud database. Calibev function was used to caliberate the data nodes. Lugui 7.2.9 and Comprehensive R Archive Network were used for table pivoting between the simulated data and the data collected. The SDAMBM was developed using a combination of components from elixir big data architecture, the research conceptual model and consumer behavior theories. Toad Modeler was then used to assemble the model. The SDAMBM was implemented using Monarch and Tableau to generate insights and data visualization of the transactions. Intelligent interpreters for auto decision grid, selectivity mechanism and customer insights were used as metrics to evaluate the model. The result showed that 79% of the customers from the customers' consumption pattern of the SDAMBM preferred buying snacks and drink as shown in the visualization report through the SDAMBM visualization dashboard. Finally, this study provided a data analytics approach for managing concept drift challenge in customers' buying pattern. Furthermore, a distinctive model for managing concept drift was also achieved. It is therefore recommended that the SDAMBM should be adopted for the enhancement of customers buying and consumption pattern by business ventures, organizations and retailers.
更多查看译文
关键词
Association rule mining, big data analytics, concept drift, market basket analysis, transactional data streams
AI 理解论文
溯源树
样例
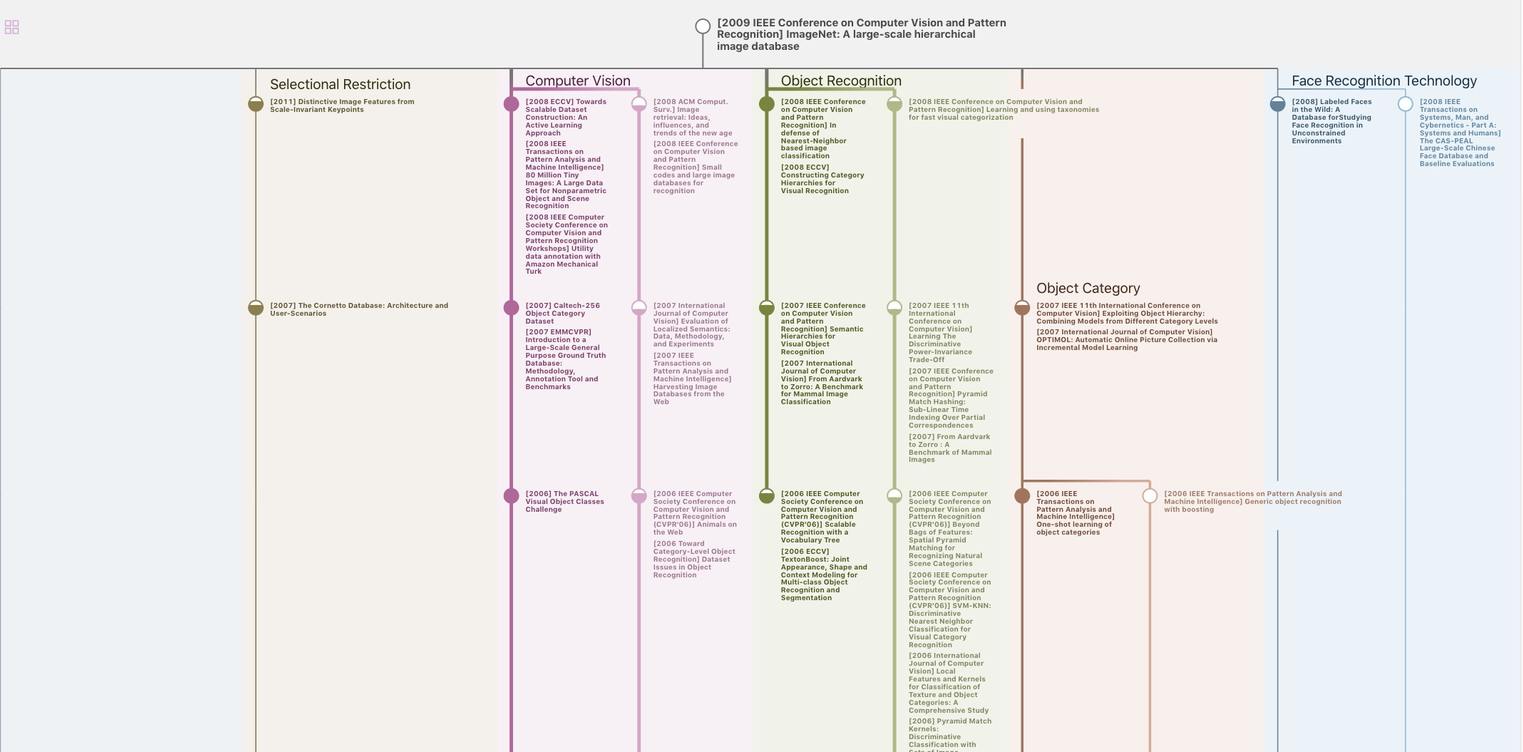
生成溯源树,研究论文发展脉络
Chat Paper
正在生成论文摘要