A process-based statistical seasonal prediction of May–July rainfall anomalies over Texas and the Southern Great Plains of the United States
Climate Services(2019)
摘要
With the aim of providing actionable drought early warning information that water managers and reservoir operators in Texas could use to implement drought contingency triggers on water supply sources, we have developed a statistical seasonal prediction system using a canonical correlation analysis prediction model to predict rainfall from May through July (MJJ), the main rainfall season over much of Texas and the Southern Great Plains. The statistical model is trained with data between 1982 and 2005 using standardized anomalous geopotential height at 500 hPa, convective inhibition energy, and soil moisture content in April as the predictors to generate tercile categorical forecasts of MJJ rainfall. Based on commonly used forecast skill metrics, this statistical prediction system provides 20–60% higher skill than that obtained from dynamical seasonal forecasts, and the exceeds skill due to the persistence of MJJ rainfall anomalies over Texas, western Louisiana, Oklahoma and the Southern Kansas. 2011 hindcast shows that below-normal MJJ rainfall anomalies comparable to those observed over most of the region. The forecasts for 2014 captured the above-normal MJJ rainfall anomalies as observed in that year. The forecasts since 2014 have shown acceptable prediction skills at one-to-three months’ lead-time. We have also extended the lead-time to generate probabilistic MJJ rainfall forecasts from January through March using a hybrid dynamical-statistical forecast scheme. The predictions have been used by the Texas Water Development Board to inform the Texas State Drought Preparedness Council and to support the implementation of drought contingency triggers for water supply sources by stakeholders, such as river authorities.
更多查看译文
关键词
Drought,Rainfall forecast,Texas,Early warning
AI 理解论文
溯源树
样例
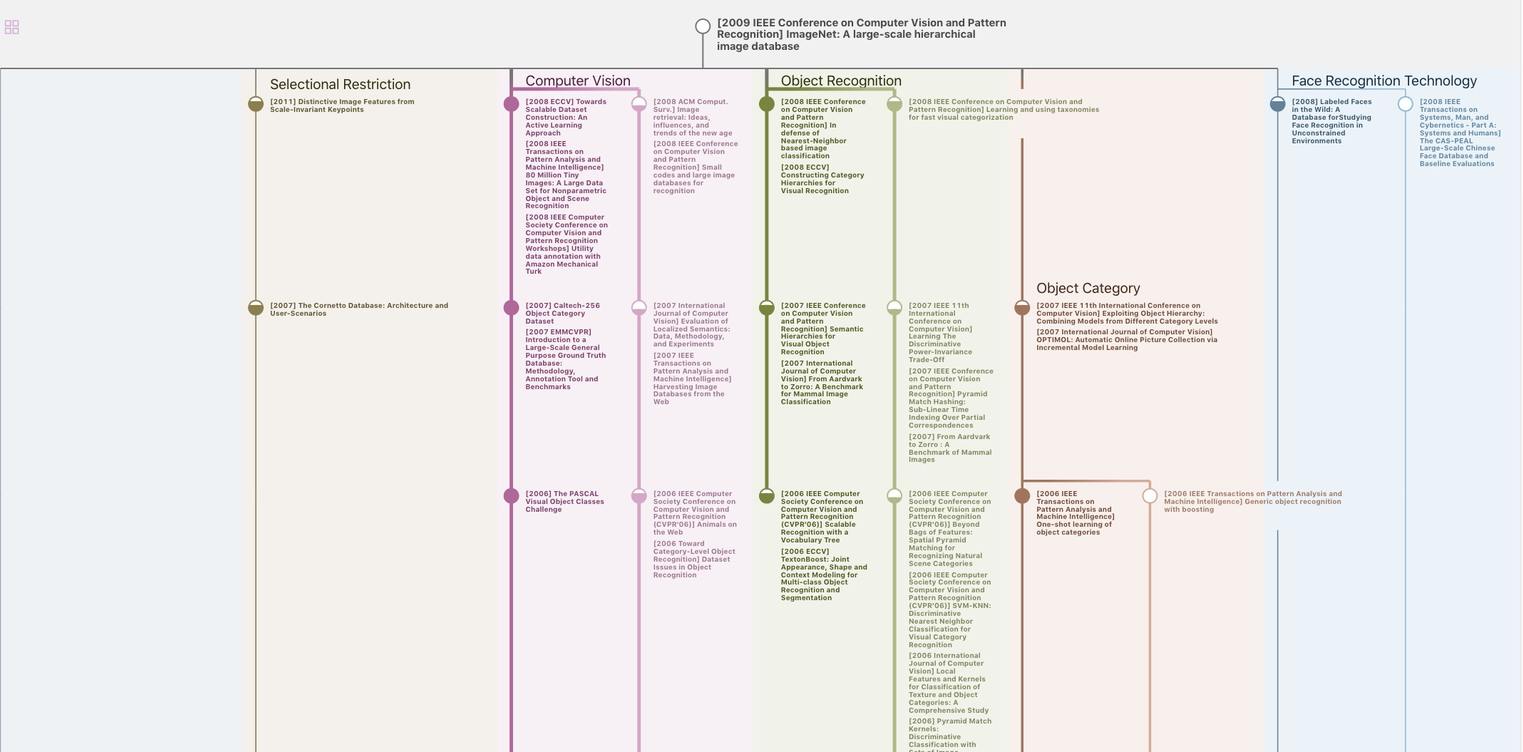
生成溯源树,研究论文发展脉络
Chat Paper
正在生成论文摘要