Embedded 1-Mb ReRAM-Based Computing-in- Memory Macro With Multibit Input and Weight for CNN-Based AI Edge Processors
IEEE Journal of Solid-State Circuits(2020)
摘要
Computing-in-memory (CIM) based on embedded nonvolatile memory is a promising candidate for energy-efficient multiply-and-accumulate (MAC) operations in artificial intelligence (AI) edge devices. However, circuit design for NVM-based CIM (nvCIM) imposes a number of challenges, including an area-latency-energy tradeoff for multibit MAC operations, pattern-dependent degradation in signal margin, and small read margin. To overcome these challenges, this article proposes the following: 1) a serial-input non-weighted product (SINWP) structure; 2) a down-scaling weighted current translator (DSWCT) and positive–negative current-subtractor (PN-ISUB); 3) a current-aware bitline clamper (CABLC) scheme; and 4) a triple-margin small-offset current-mode sense amplifier (TMCSA). A 55-nm 1-Mb ReRAM-CIM macro was fabricated to demonstrate the MAC operation of 2-b-input, 3-b-weight with 4-b-out. This nvCIM macro achieved
$T_{\text {MAC}}= 14.6$
ns at 4-b-out with peak energy efficiency of 53.17 TOPS/W.
更多查看译文
关键词
Nonvolatile memory,Artificial intelligence,Periodic structures,Resistance,Program processors,Kernel,Common Information Model (computing)
AI 理解论文
溯源树
样例
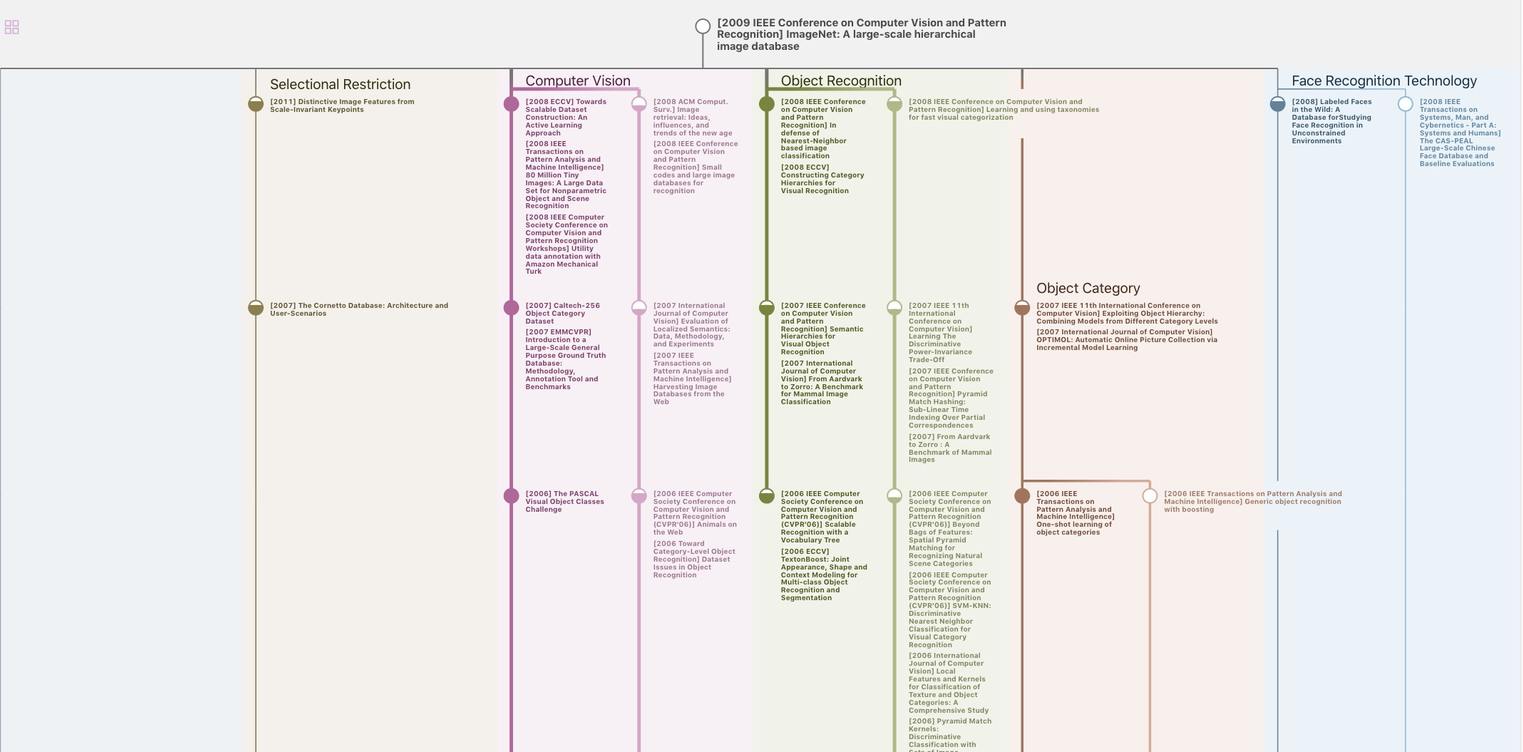
生成溯源树,研究论文发展脉络
Chat Paper
正在生成论文摘要