Towards Robust Discriminative Projections Learning via Non-greedy l 2,1 -Norm MinMax.
IEEE Transactions on Pattern Analysis and Machine Intelligence(2021)
摘要
Linear Discriminant Analysis (LDA) is one of the most successful supervised dimensionality reduction methods and has been widely used in many real-world applications. However, $\ell _2$ℓ2-norm is employed as the distance metric in the objective of LDA, which is sensitive to outliers. Many previous works improve the robustness of LDA by using $\ell _1$ℓ1-norm distance. However, the robustness again...
更多查看译文
关键词
Optimization,Robustness,Iterative algorithms,Dimensionality reduction,Principal component analysis,Prototypes,Search problems
AI 理解论文
溯源树
样例
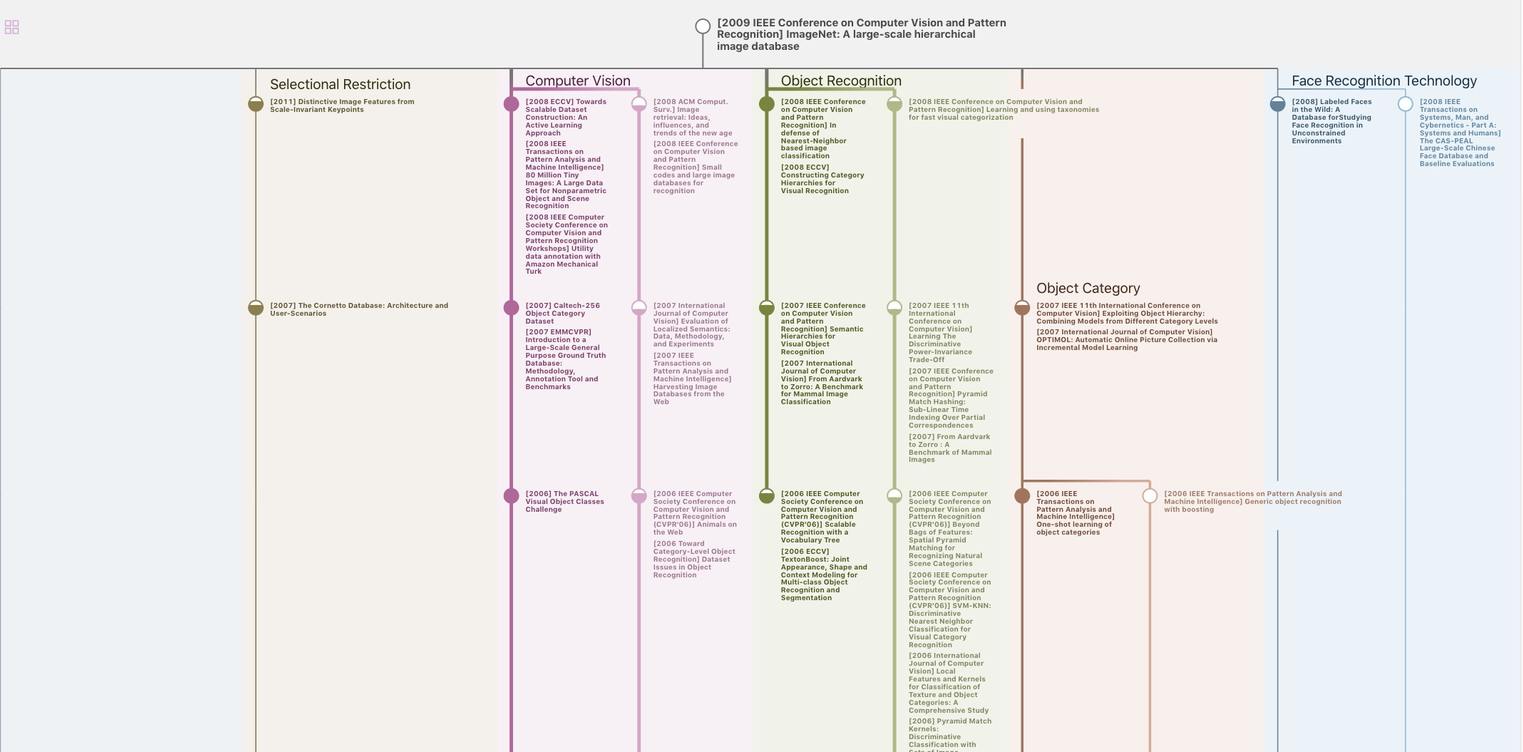
生成溯源树,研究论文发展脉络
Chat Paper
正在生成论文摘要