Group Discriminative Least Square Regression
Proceedings of the Third International Symposium on Image Computing and Digital Medicine(2019)
Abstract
Due to mathematical simplicity and computational efficiency, the least square regression (LSR) has become a popular method for multicategory classification. However, the discriminative and generalization capability of existing methods are limited. In this work, we pursue a structure constraint of the predicted label indicators of data from the same class because such structure ensures good discriminative capability and stability of the classification. To guarantee this, we propose a new regularization on the label transformation matrix. Specifically, we regularize the label transformation matrix by minimizing the difference l2,1 ofnorm and l2,2 norm of the predicted label matrix of each class. We call it group discriminative least square regression (GDLSR). In addition, we also adopt the -dragging technique to relax the binary label for better generalization capability. Experiments on several commonly used datasets show that our method outperforms other state-of-the-art LSR-based methods.
MoreTranslated text
Key words
Group sparsity, Least square regression, Multicategory classification
AI Read Science
Must-Reading Tree
Example
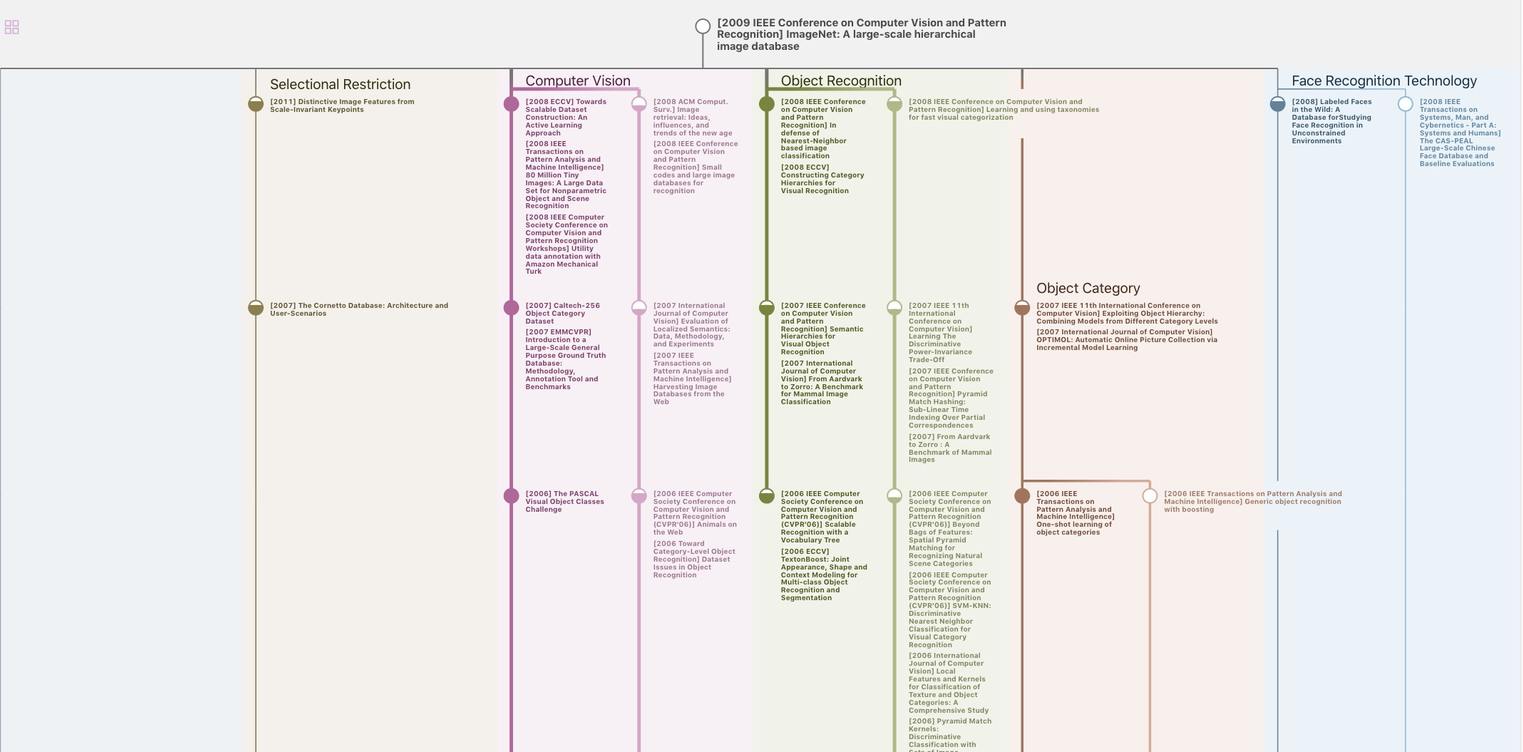
Generate MRT to find the research sequence of this paper
Chat Paper
Summary is being generated by the instructions you defined