PrivacyBot: Detecting Privacy Sensitive Information in Unstructured Texts
2019 Sixth International Conference on Social Networks Analysis, Management and Security (SNAMS)(2019)
摘要
With the swift proliferation of Internet services and always connected smart devices, users continue to (un)intentionally share copious amount of data on daily basis. While the availability of such a big amount of data is useful to extract interesting nuggets in areas such as behavioral or medical research, it also brings about unprecedented user information privacy violation consequences, e.g., identity theft and reputation damage of target users. This is aggravated when users share Privacy Sensitive Information (PSI) on-line, often times, including to unintended audience. In this regard, detecting PSI disclosure becomes an essential step towards tackling the long(short) term privacy consequences of divulging such information. As such, in this paper, we present PrivacyBot, a machine-learning based proof-of-concept that detects PSI in user-generated unstructured texts. A rigorous set of experiments show that our approach can detect PSI with an accuracy of up-to 95%. Furthermore, PrivacyBot provides a fine-grained category of PSI types (with an accuracy of up-to 88%), defined based on existing work and Art. 9 of the European Union (EU) General Data Protection Regulation (GDPR). Results are promising and shed light on the possibility of integrating such tools to support users in making informed privacy related decisions when disclose PSI on-line.
更多查看译文
关键词
Information privacy,EU GDPR,machine learning
AI 理解论文
溯源树
样例
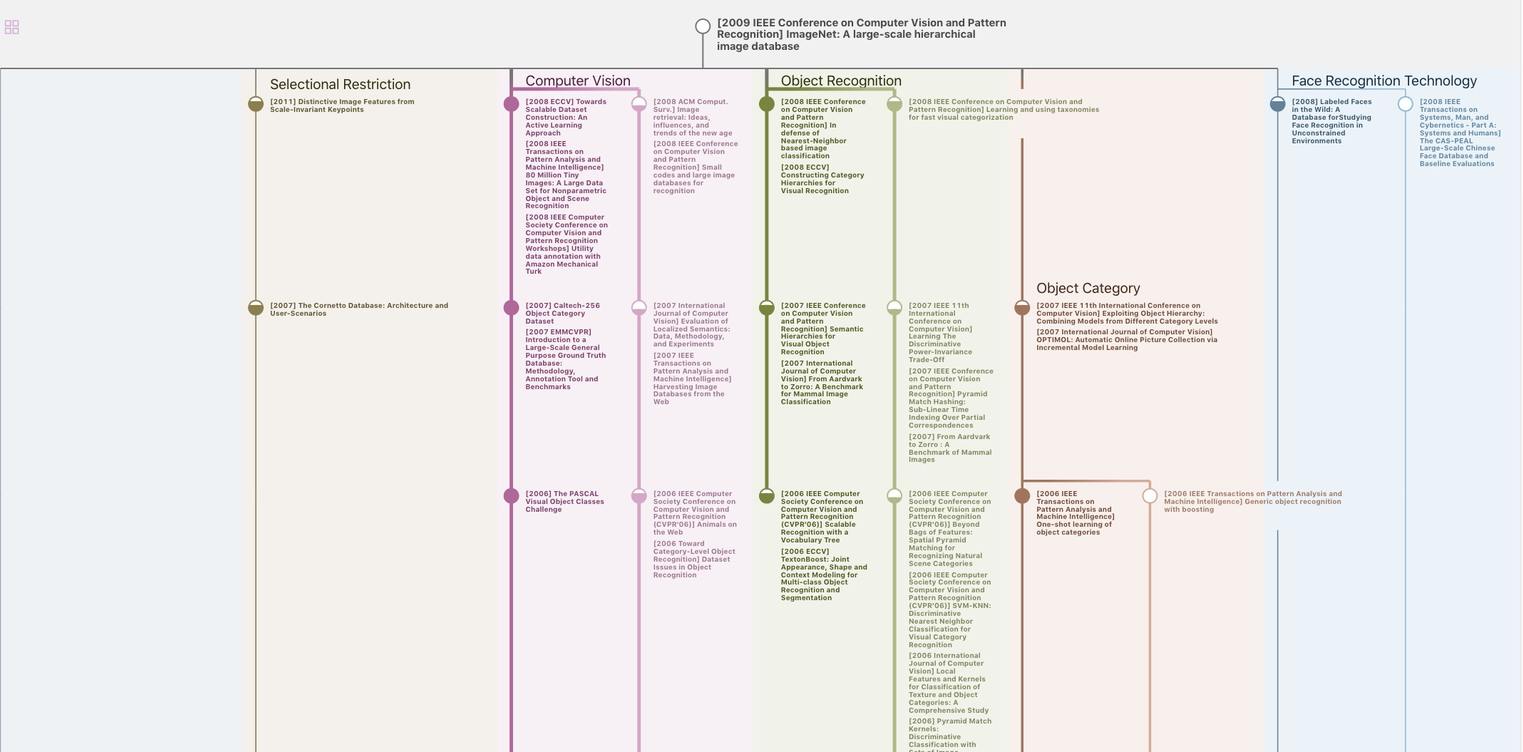
生成溯源树,研究论文发展脉络
Chat Paper
正在生成论文摘要