Weakly Supervised Representation Learning for Audio-Visual Scene Analysis
IEEE/ACM Transactions on Audio, Speech, and Language Processing(2020)
Abstract
Audio-visual (AV) representation learning is an important task from the perspective of designing machines with the ability to understand complex events. To this end, we propose a novel multimodal framework that instantiates multiple instance learning. Specifically, we develop methods that identify events and localize corresponding AV cues in unconstrained videos. Importantly, this is done using weak labels where only video-level event labels are known without any information about their location in time. We show that the learnt representations are useful for performing several tasks such as event/object classification, audio event detection, audio source separation and visual object localization. An important feature of our method is its capacity to learn from unsynchronized audio-visual events. We also demonstrate our framework's ability to separate out the audio source of interest through a novel use of nonnegative matrix factorization. State-of-the-art classification results, with a F1-score of 65.0, are achieved on DCASE 2017 smart cars challenge data with promising generalization to diverse object types such as musical instruments. Visualizations of localized visual regions and audio segments substantiate our system's efficacy, especially when dealing with noisy situations where modality-specific cues appear asynchronously.
MoreTranslated text
Key words
Visualization,Task analysis,Videos,Proposals,Feature extraction,Event detection,Source separation
AI Read Science
Must-Reading Tree
Example
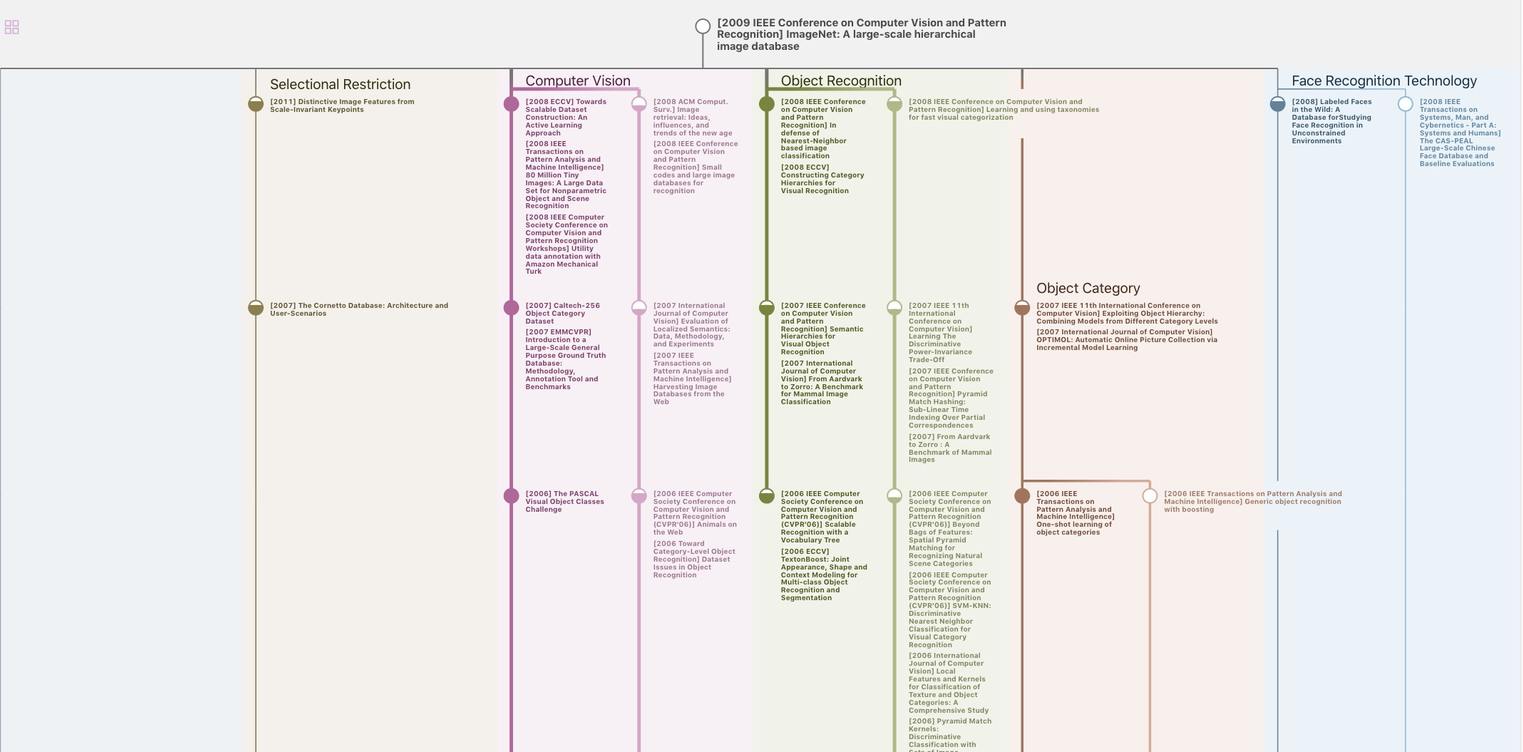
Generate MRT to find the research sequence of this paper
Chat Paper
Summary is being generated by the instructions you defined