Predicting severity and prognosis in Parkinson's disease from brain microstructure and connectivity.
NeuroImage. Clinical(2019)
摘要
OBJECTIVES:Investigating biomarkers to demonstrate progression of Parkinson's disease (PD) is of high priority. We investigated the association of brain structural properties with progression of clinical outcomes and their ability to differentiate clinical subtypes of PD.
METHODS:A comprehensive set of clinical features was evaluated at baseline and 4.5-year follow-up for 144 de-novo PD patients from the Parkinson's Progression Markers Initiative. We created a global composite outcome (GCO) by combining z-scores of non-motor and motor symptoms, motor signs, overall activities of daily living and global cognition, as a single numeric indicator of prognosis. We classified patients into three subtypes based on multi-domain clinical criteria: 'mild motor-predominant', 'intermediate' and 'diffuse-malignant'. We analyzed diffusion-weighted scans at the early drug-naïve stage and extracted fractional anisotropy and mean diffusivity (MD) of basal ganglia and cortical sub-regions. Then, we employed graph theory to calculate network properties and used network-based statistic to investigate our primary hypothesis.
RESULTS:Baseline MD of globus pallidus was associated with worsening of motor severity, cognition, and GCO after 4.5 years of follow-up. Connectivity disruption at baseline was correlated with decline in cognition, and increase in GCO. Baseline MD of nucleus accumbens, globus pallidus and basal-ganglia were linked to clinical subtypes at 4.5-year of follow-up. Disruption in sub-cortical networks associated with being subtyped as 'diffuse-malignant' versus 'mild motor-predominant' after 4.5 years.
CONCLUSIONS:Diffusion imaging analysis at the early de-novo stage of PD was able to differentiate clinical sub-types of PD after 4.5 years and was highly associated with future clinical outcomes of PD.
更多查看译文
AI 理解论文
溯源树
样例
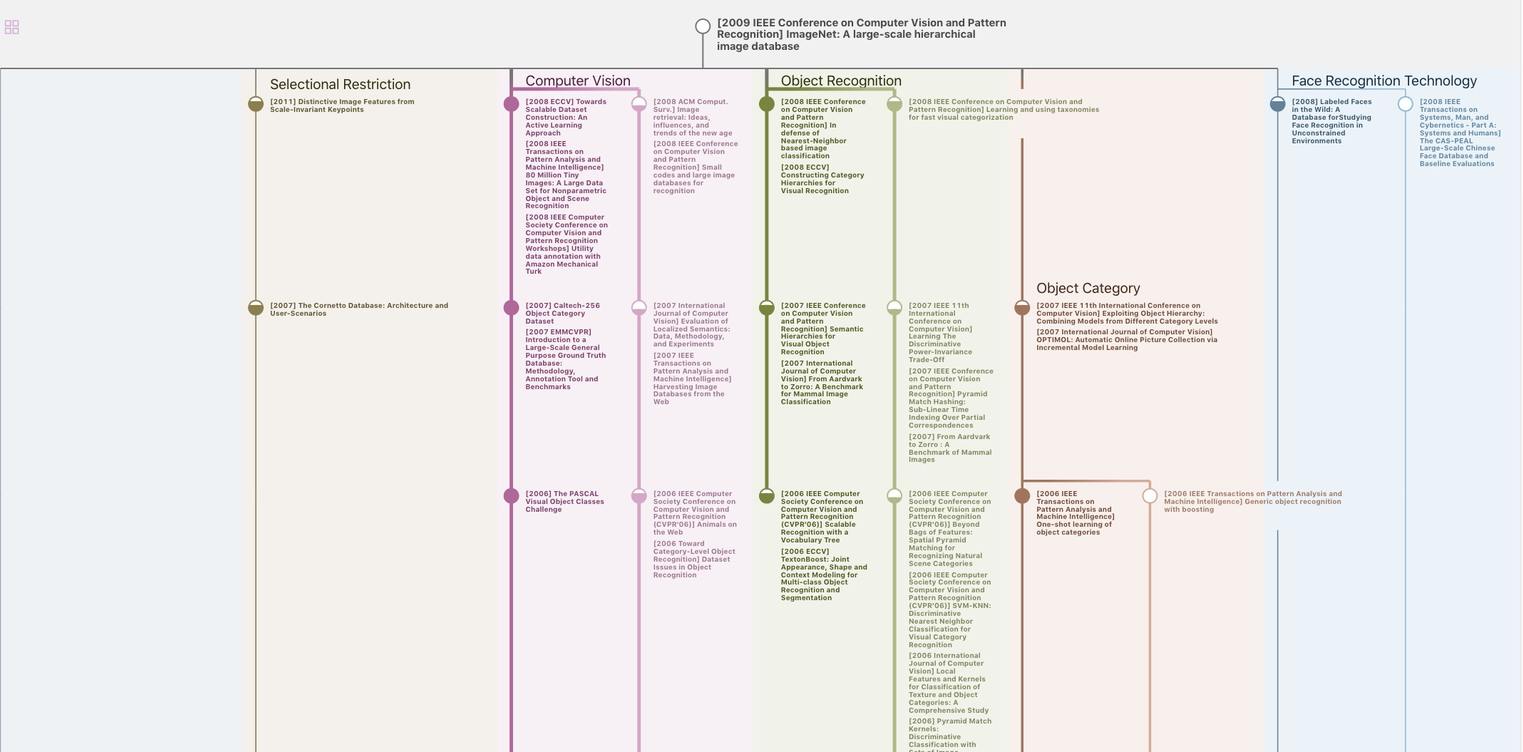
生成溯源树,研究论文发展脉络
Chat Paper
正在生成论文摘要