Sampled Limited Memory Methods For Massive Linear Inverse Problems
INVERSE PROBLEMS(2020)
摘要
In many modern imaging applications the desire to reconstruct high resolution images, coupled with the abundance of data from acquisition using ultra-fast detectors, have led to new challenges in image reconstruction. A main challenge is that the resulting linear inverse problems are massive. The size of the forward model matrix exceeds the storage capabilities of computer memory, or the observational dataset is enormous and not available all at once. Row-action methods that iterate over samples of rows can be used to approximate the solution while avoiding memory and data availability constraints. However, their overall convergence can be slow. In this paper, we introduce a sampled limited memory row-action method for linear least squares problems, where an approximation of the global curvature of the underlying least squares problem is used to speed up the initial convergence and to improve the accuracy of iterates. We show that this limited memory method is a generalization of the damped block Kaczmarz method, and we prove linear convergence of the expectation of the iterates and of the error norm up to a convergence horizon. Numerical experiments demonstrate the benefits of these sampled limited memory row-action methods for massive 2D and 3D inverse problems in tomography applications.
更多查看译文
关键词
least squares problems, row-action methods, Kaczmarz methods, randomized methods, tomography, streaming data
AI 理解论文
溯源树
样例
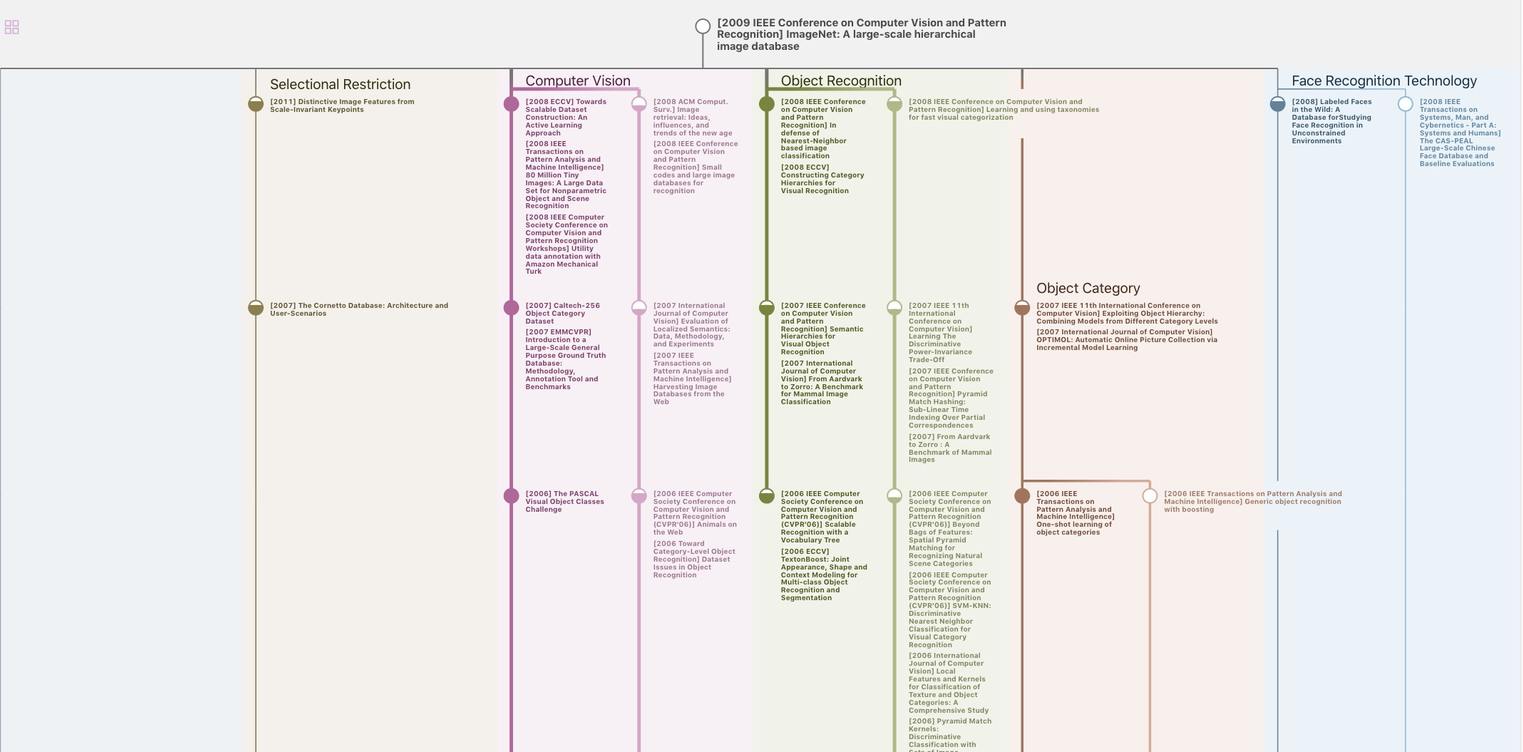
生成溯源树,研究论文发展脉络
Chat Paper
正在生成论文摘要