Data Augmentation For Ambulatory Eeg Based Cognitive State Taxonomy System With Rnn-Lstm
ARTIFICIAL INTELLIGENCE XXXVI(2019)
摘要
Emotion detection is an important step for recognizing a person's mental state. A physiological signal, Electroencephalogram (EEG) is analyzed to detect human emotion with promising results. The cost of information gathering and lack of number of participants incur a limitation on the size of EEG data set. The deficiency in acquired EEG data set makes it difficult to estimate mental states with deep learning models as it requires a larger size of the training data set. In this paper, we propose a novel data augmentation method to address challenges due to scarcity of EEG data for training deep learning models such as Recurrent Neural Network - Long Short Term Memory (RNN-LSTM). To find the performance of mental state estimator such models are applied before and after proposed data augmentation. Experimental results demonstrate that data augmentation improves the performance of mental state estimator with an accuracy of 98%.
更多查看译文
关键词
Data augmentation, EEG (Electroencephalogram), Recurrent Neural Network (RNN), Long Short Term Memory (LSTM)
AI 理解论文
溯源树
样例
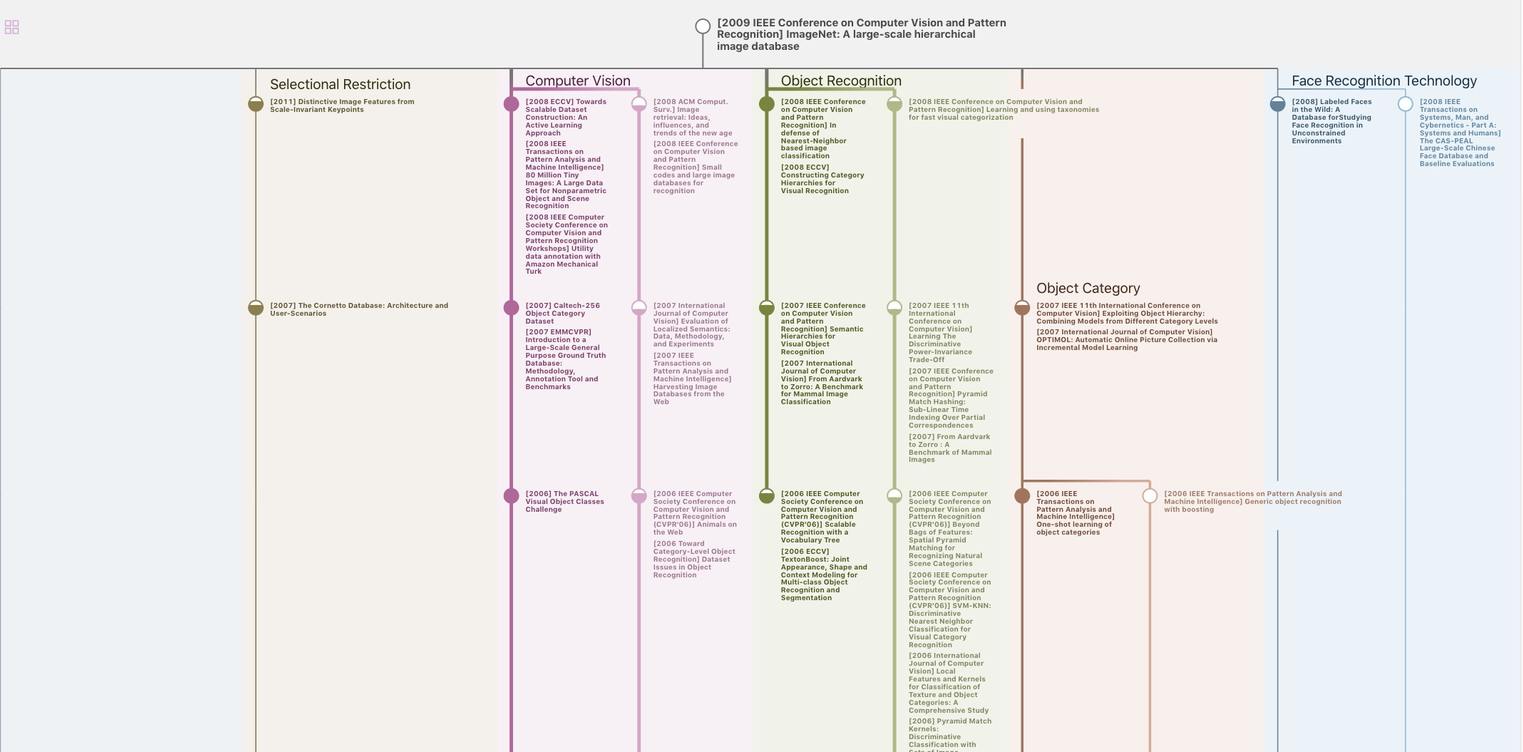
生成溯源树,研究论文发展脉络
Chat Paper
正在生成论文摘要