PEEPLL: privacy-enhanced event pseudonymisation with limited linkability
PROCEEDINGS OF THE 35TH ANNUAL ACM SYMPOSIUM ON APPLIED COMPUTING (SAC'20)(2020)
摘要
Pseudonymisation provides the means to reduce the privacy impact of data collection and processing on individual subjects. Its application on data records, especially in an environment with additional constraints, like re-identification in the course of incident response, implies assumptions and privacy issues, which contradict the achievement of the desirable privacy level. Proceeding from two real-world scenarios, where personal and identifying data needs to be processed, we identify a system model for pseudonymisation and explicitly state the sustained privacy threats. With this system and threat model, we derive privacy protection goals together with possible technical realisations, which are integrated into our event pseudonymisation framework PEEPLL for the context of event processing, like auditing of user activities. Our framework provides privacy-friendly linkability in order to maintain the possibility for automatic event correlation and evaluation, while at the same time reduces the privacy impact on individuals. With this framework, privacy provided by event pseudonymisation can be enhanced by a more rigorous commitment to the concept of personal data minimisation.
更多查看译文
关键词
personal data minimisation, pseudonym re-usage, privacy protection goals, pseudonymisation framework, indistinguishability, unobservability, limited linkability
AI 理解论文
溯源树
样例
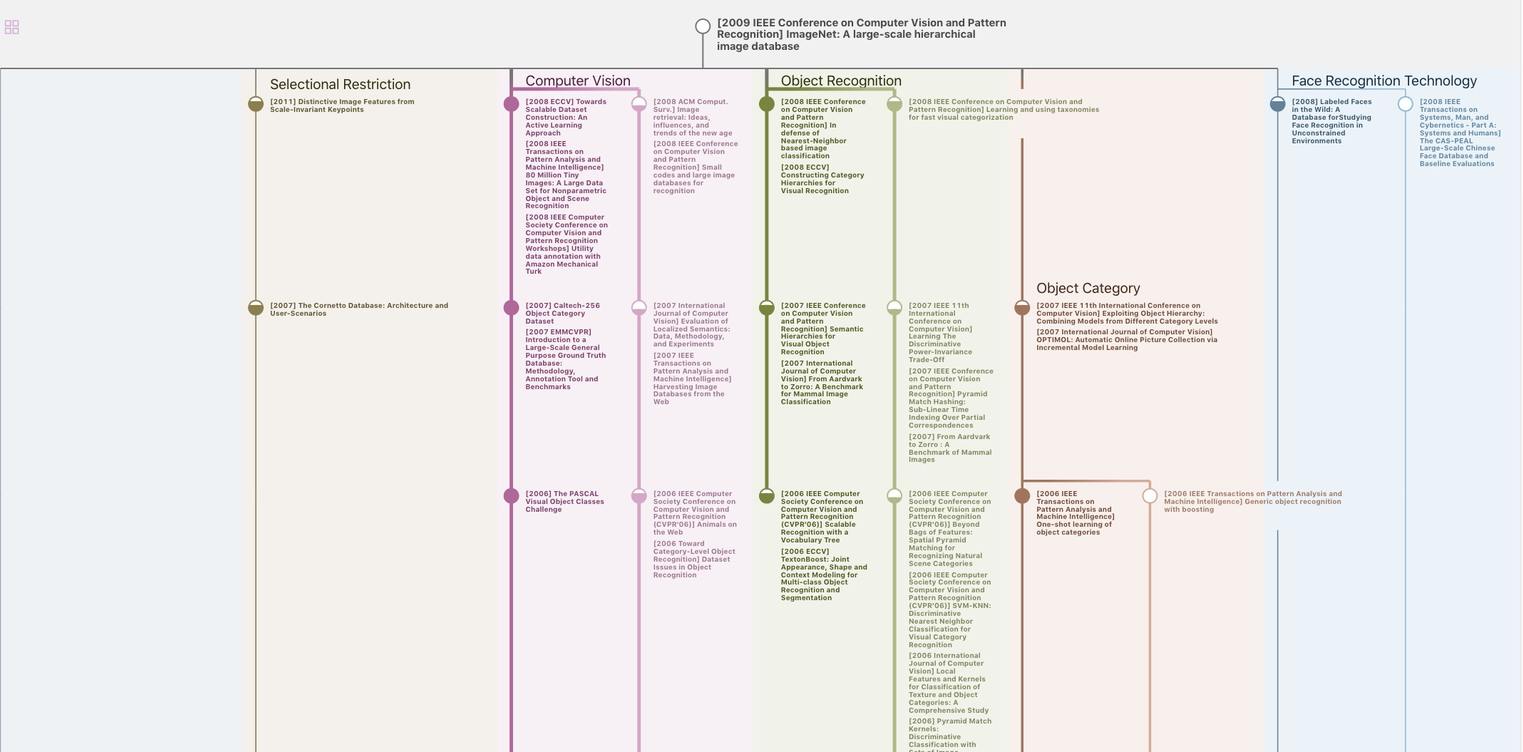
生成溯源树,研究论文发展脉络
Chat Paper
正在生成论文摘要