CsiGAN: Robust Channel State Information-Based Activity Recognition With GANs
IEEE Internet of Things Journal(2019)
Abstract
As a cornerstone service for many Internet of Things applications, channel state information (CSI)-based activity recognition has received immense attention over recent years. However, recognition performance of general approaches might significantly decrease when applying the trained model to the left-out user whose CSI data are not used for model training. To overcome this challenge, we propose a semi-supervised generative adversarial network (GAN) for CSI-based activity recognition (CsiGAN). Based on the general semi-supervised GANs, we mainly design three components for CsiGAN to meet the scenarios that unlabeled data from left-out users are very limited and enhance recognition performance: 1) we introduce a new complement generator, which can use limited unlabeled data to produce diverse fake samples for training a robust discriminator; 2) for the discriminator, we change the number of probability outputs from
$k+1$
into
$2k+1$
(here,
$k$
is the number of categories), which can help to obtain the correct decision boundary for each category; and 3) based on the introduced generator, we propose a manifold regularization, which can stabilize the learning process. The experiments suggest that CsiGAN attains significant gains compared to the state-of-the-art methods.
MoreTranslated text
Key words
Gallium nitride,Activity recognition,Generators,Training,Data models,Semisupervised learning,Manifolds
AI Read Science
Must-Reading Tree
Example
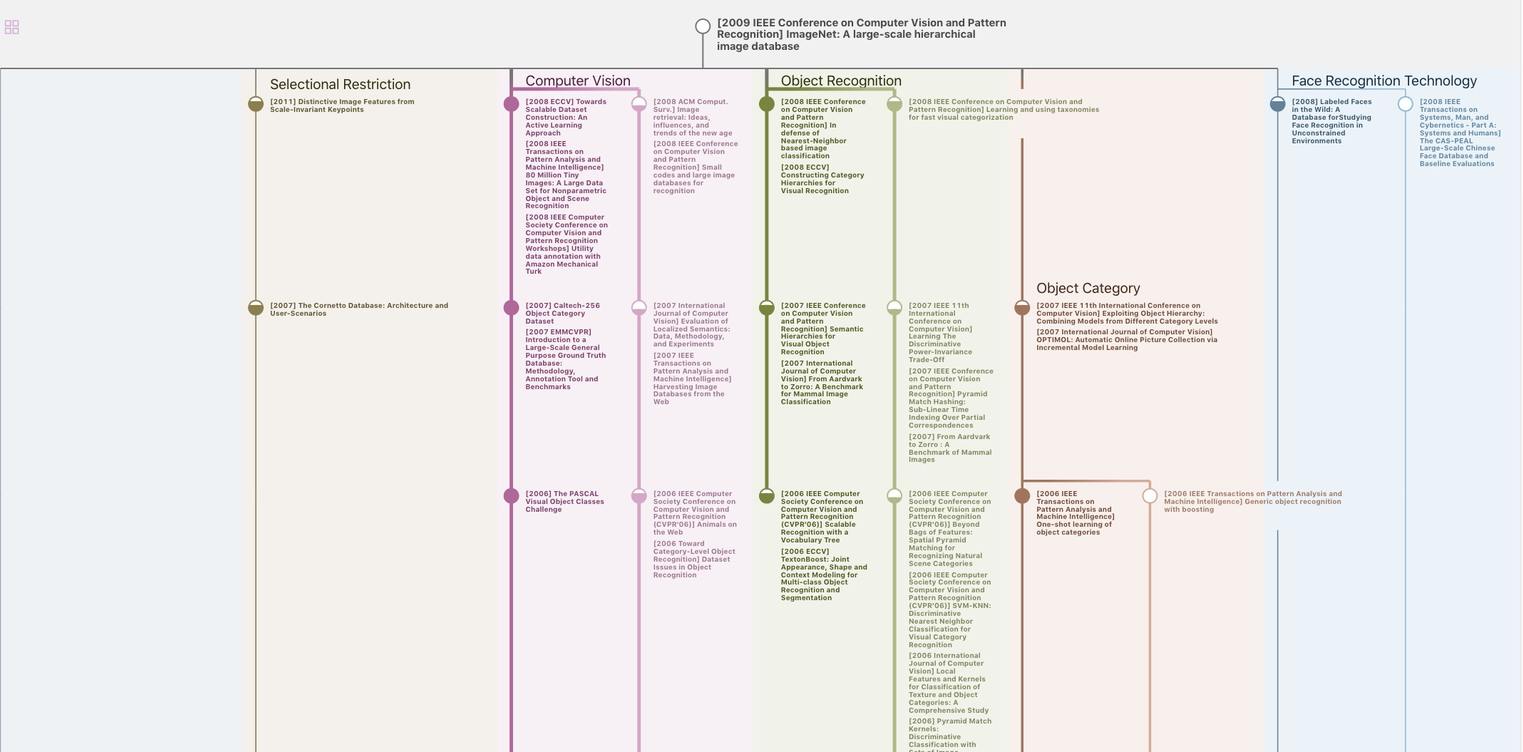
Generate MRT to find the research sequence of this paper
Chat Paper
Summary is being generated by the instructions you defined