What You See is What You Get: Exploiting Visibility for 3D Object Detection
CVPR(2020)
摘要
Recent advances in 3D sensing have created unique challenges for computer vision. One fundamental challenge is finding a good representation for 3D sensor data. Most popular representations (such as PointNet) are proposed in the context of processing truly 3D data (e.g. points sampled from mesh models), ignoring the fact that 3D sensored data such as a LiDAR sweep is in fact 2.5D. We argue that representing 2.5D data as collections of (x, y, z) points fundamentally destroys hidden information about freespace. In this paper, we demonstrate such knowledge can be efficiently recovered through 3D raycasting and readily incorporated into batch-based gradient learning. We describe a simple approach to augmenting voxel-based networks with visibility: we add a voxelized visibility map as an additional input stream. In addition, we show that visibility can be combined with two crucial modifications common to state-of-the-art 3D detectors: synthetic data augmentation of virtual objects and temporal aggregation of LiDAR sweeps over multiple time frames. On the NuScenes 3D detection benchmark, we show that, by adding an additional stream for visibility input, we can significantly improve the overall detection accuracy of a state-of-the-art 3D detector.
更多查看译文
关键词
synthetic data augmentation,LiDAR sweep,NuScenes 3D detection benchmark,visibility input,state-of-the-art 3D detector,exploiting visibility,3D object detection,good representation,3D sensor data,popular representations,truly 3D data,3D sensored data,batch-based gradient learning,voxel-based networks,voxelized visibility map
AI 理解论文
溯源树
样例
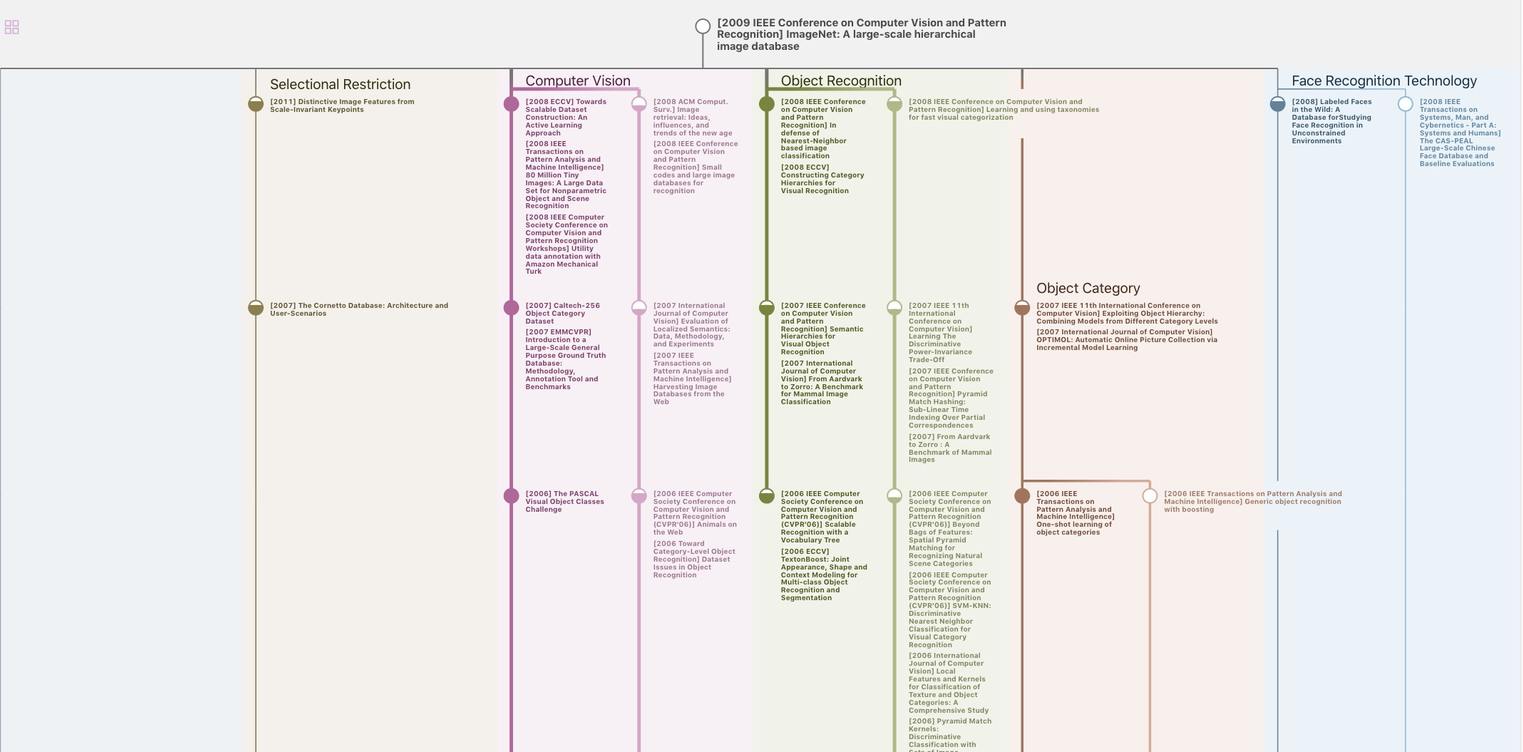
生成溯源树,研究论文发展脉络
Chat Paper
正在生成论文摘要