From Comparison To Retrieval: Scalable Change Retrieval From Discriminatively Learned Deep Three-Dimensional Neural Codes
2019 IEEE INTELLIGENT TRANSPORTATION SYSTEMS CONFERENCE (ITSC)(2019)
摘要
We present a novel scalable framework for image change detection (ICD) from an on-board 3D imagery system. We argue that existing ICD systems are constrained by the time required to align a given query image with individual reference image coordinates. We utilize an invariant coordinate system (ICS) to replace the time-consuming image alignment with an offline pre-processing procedure. Our key contribution is an extension of the traditional image comparison-based ICD tasks to setups of the image retrieval (IR) task. We replace each component of the 3D ICD system, i.e., (1) image modeling, (2) image alignment, and (3) image differencing, with significantly efficient variants from the bag-of-words (BoW) IR paradigm. Further, we train a deep 3D feature extractor in an unsupervised manner using an unsupervised Siamese network and automatically collected training data. We conducted experiments on a challenging cross-season ICD task using a publicly available dataset and thereby validate the efficacy of the proposed approach.
更多查看译文
关键词
3D imagery system,image comparison-based ICD,deep 3D feature extractor training,invariant coordinate system,image modeling,image differencing,unsupervised siamese network,bag-of-words IR paradigm,image retrieval,image alignment,image coordinates,image change detection
AI 理解论文
溯源树
样例
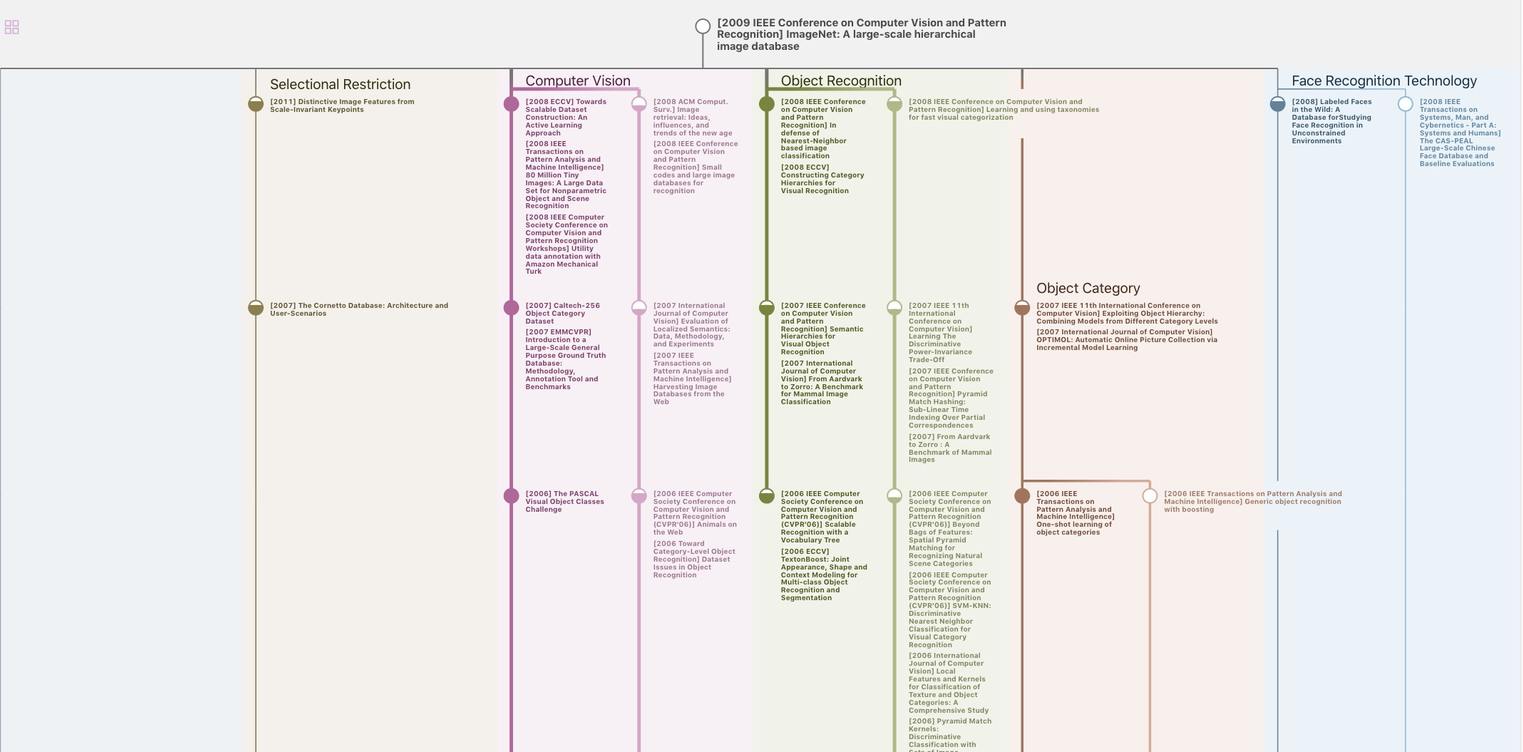
生成溯源树,研究论文发展脉络
Chat Paper
正在生成论文摘要