Data poisoning attacks on neighborhood-based recommender systems
Periodicals(2021)
Abstract
Nowadays, collaborative filtering recommender systems have been widely deployed in many commercial companies to make profit. Neighborhood-based collaborative filtering (CF) is common and effective. To date, despite its effectiveness, there has been little effort to explore their robustness and the impact of data poisoning attacks on their performance. Can the neighborhood-based recommender systems be easily fooled? To this end, we shed light on the robustness of neighborhood-based recommender systems and propose a novel data poisoning attack framework, encoding the purpose of attack and constraint against them. We first illustrate how to calculate the optimal data poisoning attack, namely, UNAttack. We inject a few well-designed fake users into the recommender systems such that target items will be recommended to as many normal users as possible. Extensive experiments are conducted on three real-world datasets to validate the effectiveness and the transferability of our proposed method. In addition, some interesting phenomena can be found. For example, (i) neighborhood-based recommender systems with Euclidean distance-based similarity have strong robustness and (ii) the fake users can be transferred to attack the state-of-the-art CF recommender systems such as neural CF and Bayesian personalized ranking matrix factorization.
MoreTranslated text
Key words
adversarial attack,data poisoning,recommender system,robustness
AI Read Science
Must-Reading Tree
Example
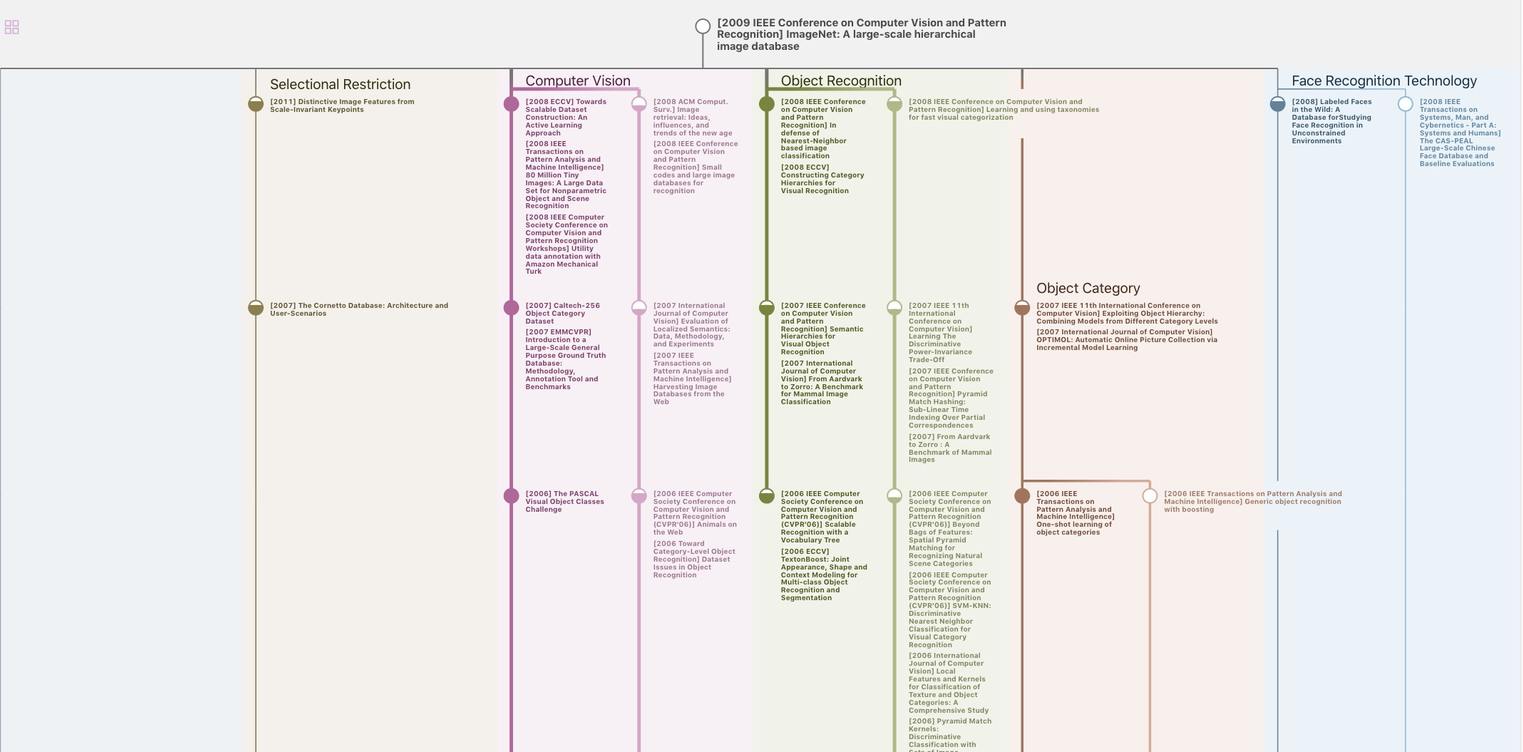
Generate MRT to find the research sequence of this paper
Chat Paper
Summary is being generated by the instructions you defined