SAR image super-resolution using deep residual SqueezeNet
Proceedings of the International Conference on Artificial Intelligence, Information Processing and Cloud Computing(2019)
摘要
Enhancing the resolution of SAR image can significantly improve more complicated application such as automatic target detection. This paper proposes a new algorithm to reconstruct super-resolution image by using a deep residual SqueezeNet. It directly achieves end to end mapping from the low-resolution image to a high-resolution image with the convolutional neural network. The lightweight SqueezeNet with fewer parameters but equivalent accuracy compared to a well-known model is used to reduce the computational complexity. Moreover, inspired by ResNet, we utilize the residual learning method with high learning rates to accelerate convergence. Experimental results demonstrate that the new algorithm brought forward in this paper not only has lightweight construction, but also can be faster, and get similar or better results than state-of-the-art methods.
更多查看译文
关键词
CNN, SAR image, image super-resolution, residual SqueezeNet
AI 理解论文
溯源树
样例
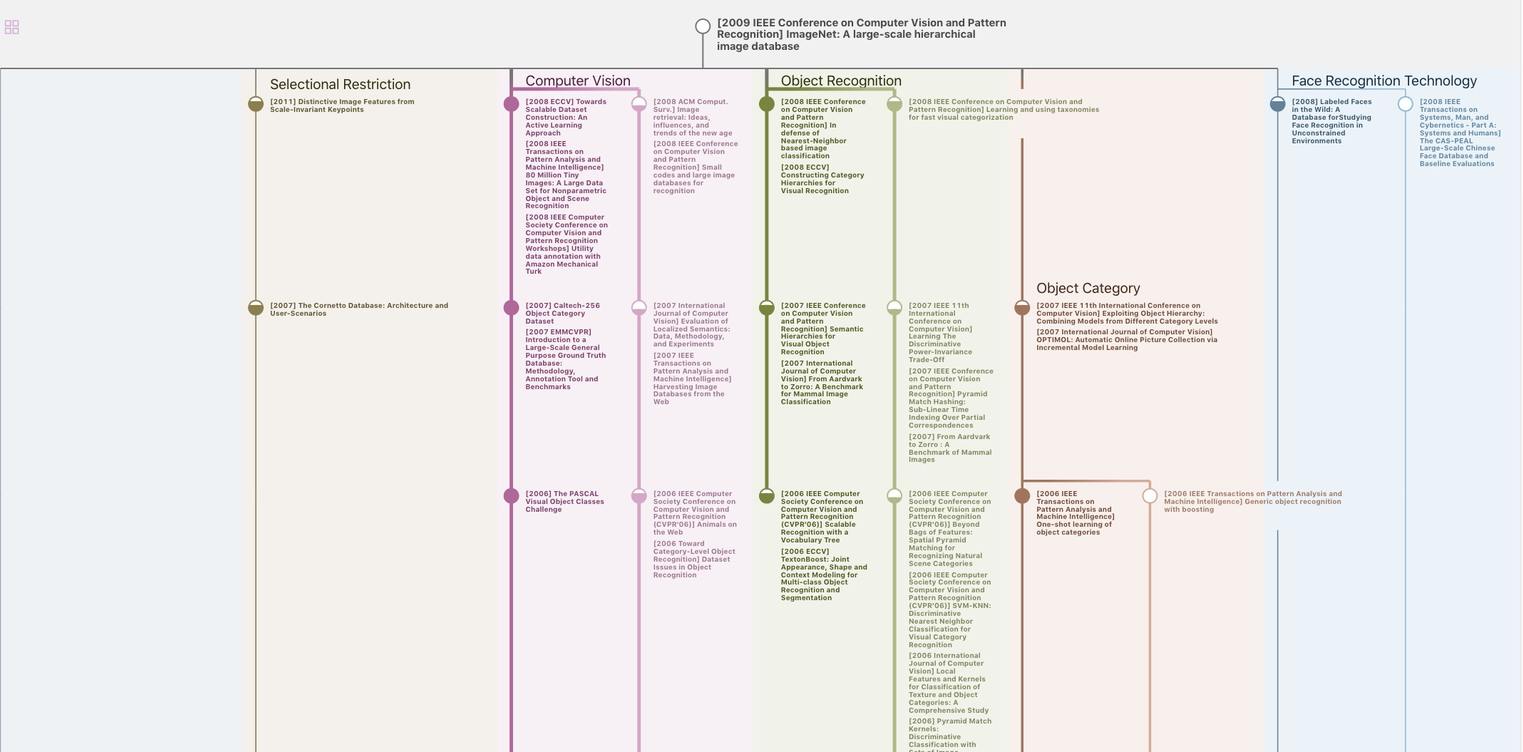
生成溯源树,研究论文发展脉络
Chat Paper
正在生成论文摘要